Receive a weekly summary and discussion of the top papers of the week by leading researchers in the field.
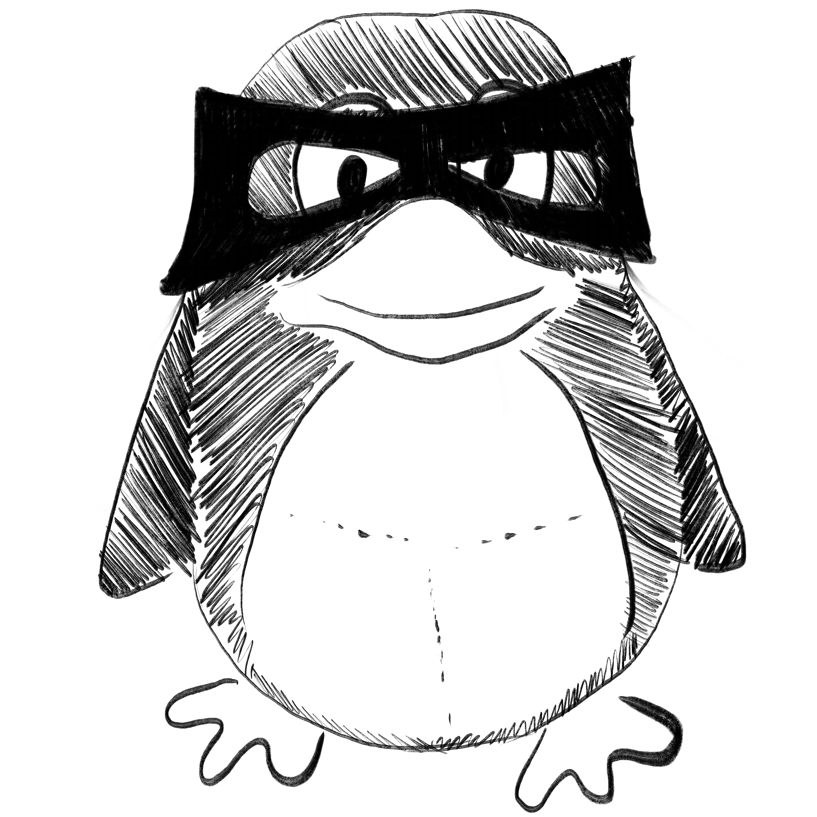
Determination of conditioning factors for mapping nickel contamination susceptibility in groundwater in Kanchanaburi Province, Thailand, using random forest and maximum entropy.
In Environmental geochemistry and health
Thanh Nguyen Ngoc, Chotpantarat Srilert, Ha Nam-Thang, Trung Nguyen H
2023-Mar-07
Groundwater, Maximum entropy, Nickel, Random forest, Recursive feature elimination, Thailand
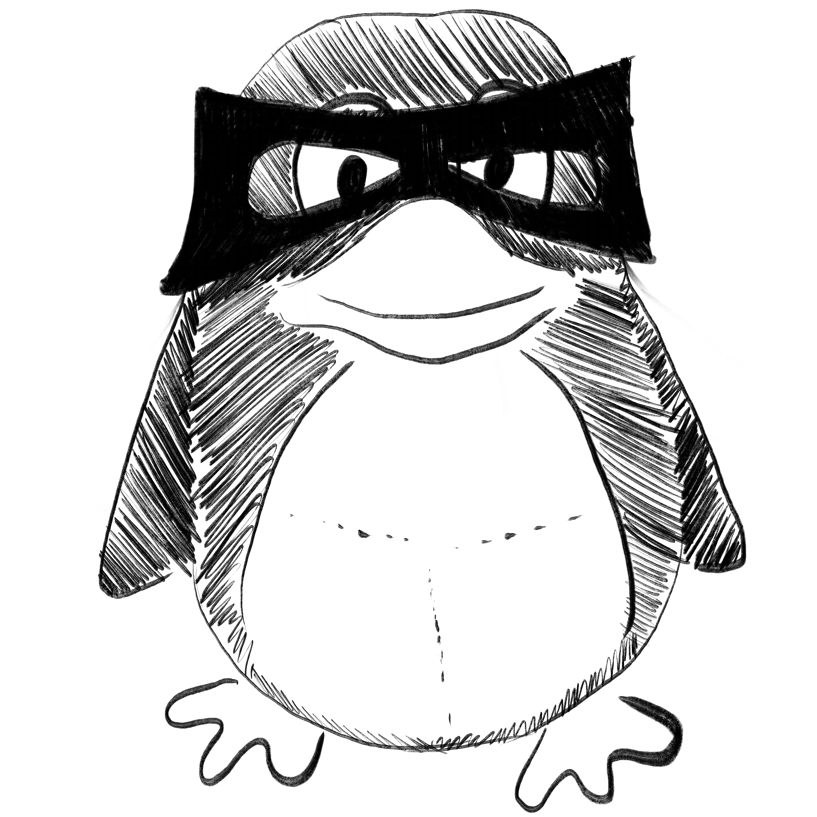
Linear Binary Classifier to Predict Bacterial Biofilm Formation on Polyacrylates.
In ACS applied materials & interfaces ; h5-index 147.0
Contreas Leonardo, Hook Andrew L, Winkler David A, Figueredo Grazziela, Williams Paul, Laughton Charles A, Alexander Morgan R, Williams Philip M
2023-Mar-07
bacterial attachment, classification, healthcare-associated infections, machine learning, polyacrylates
A Machine Learning Approach to Developing an Accurate Prediction of Maximal Heart Rate During Exercise Testing in Apparently Healthy Adults.
In Journal of cardiopulmonary rehabilitation and prevention
PURPOSE :
METHODS :
RESULTS :
CONCLUSIONS :
Cundrič Larsen, Bosnić Zoran, Kaminsky Leonard A, Myers Jonathan, Peterman James E, Markovic Vidan, Arena Ross, Popović Dejana
2023-Mar-08
Autonomous Chest Radiograph Reporting Using AI: Estimation of Clinical Impact.
In Radiology ; h5-index 91.0
Plesner Louis L, Müller Felix C, Nybing Janus D, Laustrup Lene C, Rasmussen Finn, Nielsen Olav W, Boesen Mikael, Andersen Michael B
2023-Mar-07
Deep Learning for Head and Neck CT Angiography: Stenosis and Plaque Classification.
In Radiology ; h5-index 91.0
Fu Fan, Shan Yi, Yang Guang, Zheng Chao, Zhang Miao, Rong Dongdong, Wang Ximing, Lu Jie
2023-Mar-07
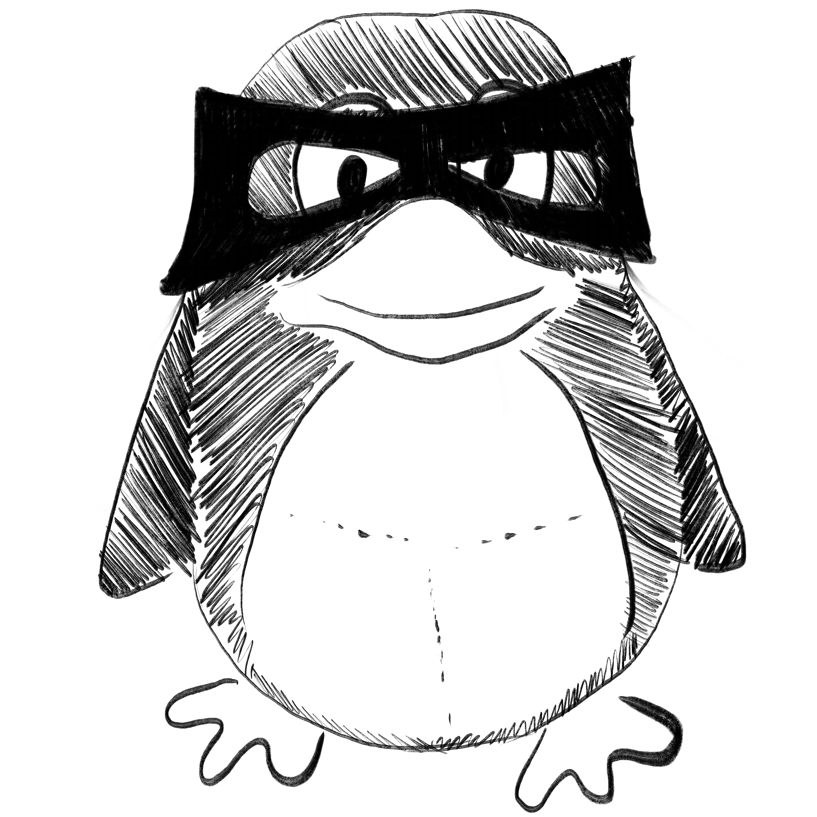
A data set of earthquake bulletin and seismic waveforms for Ghana obtained by deep learning.
In Data in brief
Mohammadigheymasi Hamzeh, Tavakolizadeh Nasrin, Matias Luís, Mousavi S Mostafa, Moradichaloshtori Yahya, Mousavirad Seyed Jalaleddin, Fernandes Rui
2023-Apr
Deep learning, Earthquake waveforms, Live Matlab figures, Seismic catalog
Weekly Summary
Receive a weekly summary and discussion of the top papers of the week by leading researchers in the field.