Receive a weekly summary and discussion of the top papers of the week by leading researchers in the field.
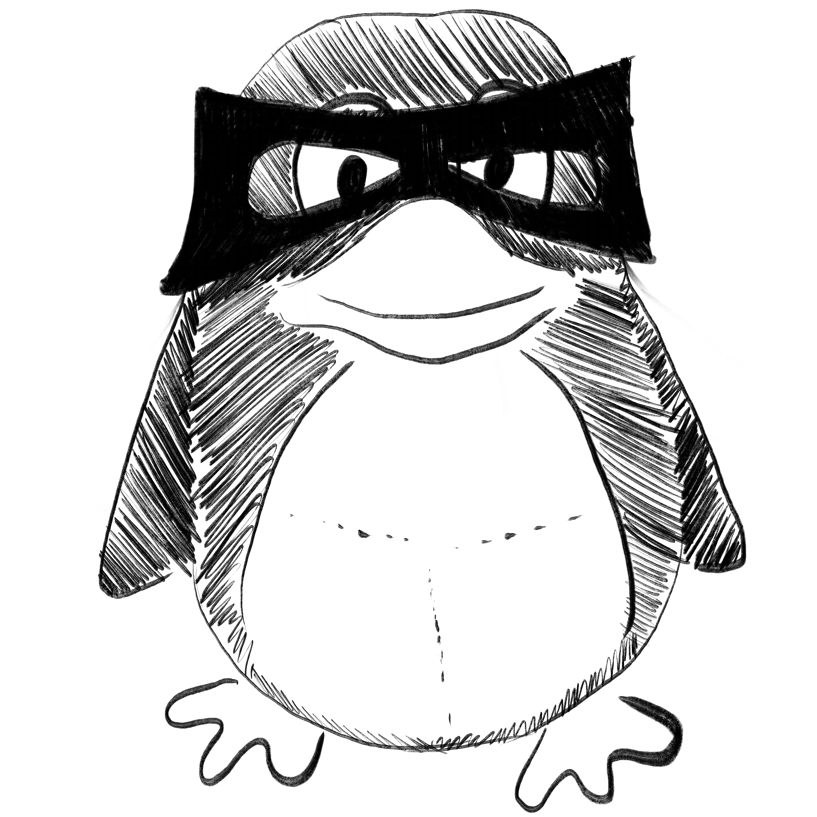
Better Together: Using Multi-task Learning to Improve Feature Selection within Structural Datasets
ArXiv Preprint
S. C. Bee, E. Papatheou, M Haywood-Alexander, R. S. Mills, L. A. Bull, K. Worden, N. Dervilis
2023-03-08
Deep-Learning for Epicardial Adipose Tissue Assessment With Computed Tomography: Implications for Cardiovascular Risk Prediction.
In JACC. Cardiovascular imaging
BACKGROUND :
OBJECTIVES :
METHODS :
RESULTS :
CONCLUSIONS :
West Henry W, Siddique Muhammad, Williams Michelle C, Volpe Lucrezia, Desai Ria, Lyasheva Maria, Thomas Sheena, Dangas Katerina, Kotanidis Christos P, Tomlins Pete, Mahon Ciara, Kardos Attila, Adlam David, Graby John, Rodrigues Jonathan C L, Shirodaria Cheerag, Deanfield John, Mehta Nehal N, Neubauer Stefan, Channon Keith M, Desai Milind Y, Nicol Edward D, Newby David E, Antoniades Charalambos
2023-Jan-10
adipose tissue, atherosclerosis, atrial fibrillation, computed tomography, deep-learning, visceral fat
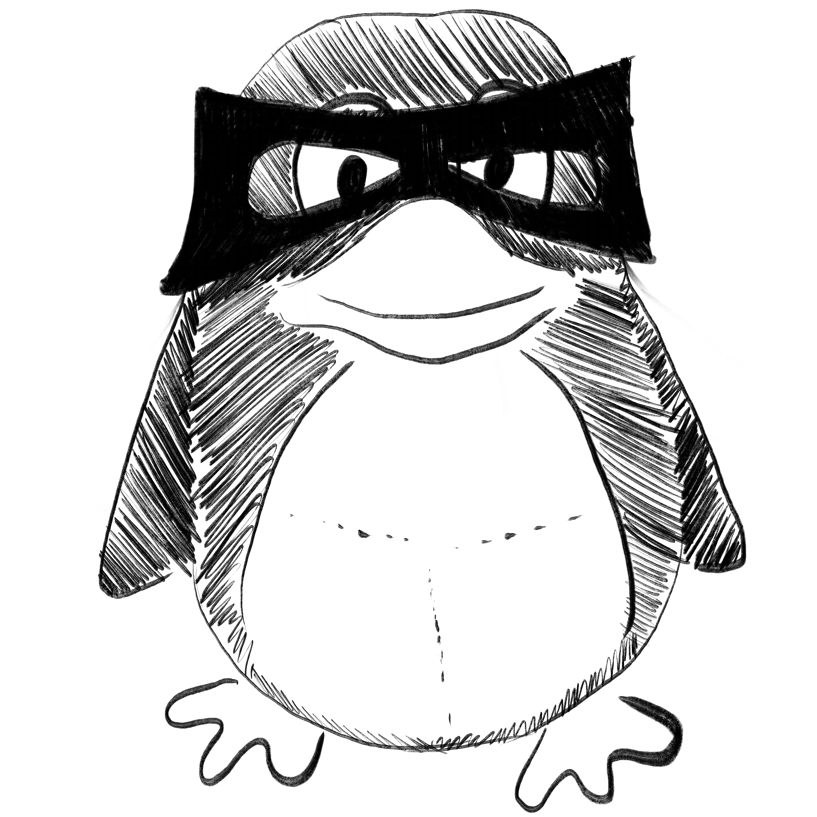
Machine learning-aided scoring of synthesis difficulties for designer chromosomes.
In Science China. Life sciences
Zheng Yan, Song Kai, Xie Ze-Xiong, Han Ming-Zhe, Guo Fei, Yuan Ying-Jin
2023-Mar-03
artificial chromosome, chemical synthesis, machine learning, synthetic biology
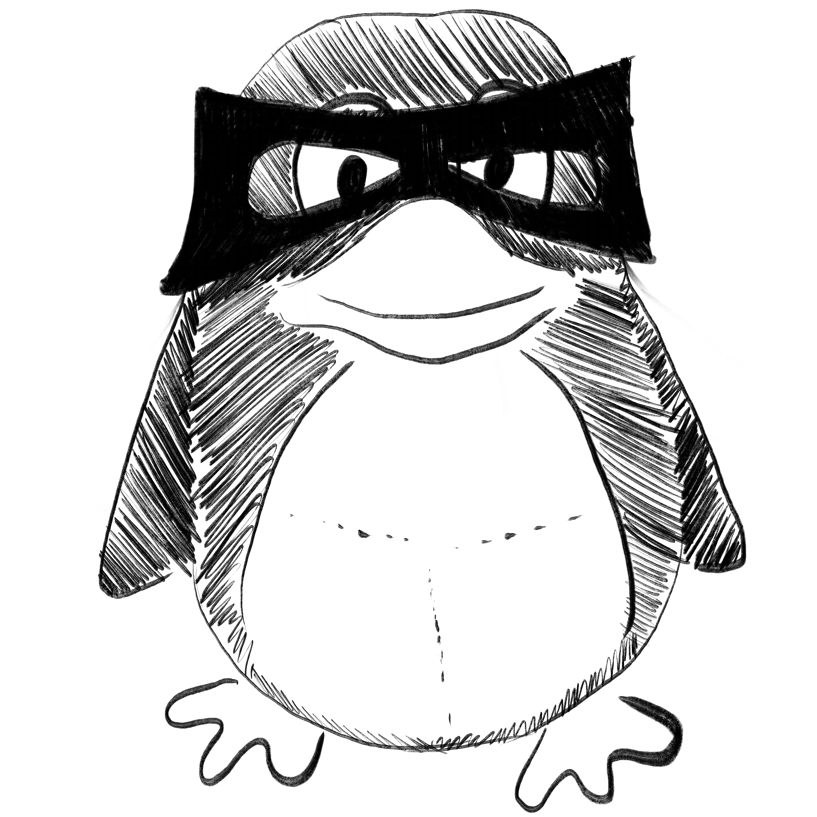
Defining Selective Neuronal Resilience and Identifying Targets of Neuroprotection and Axon Regeneration Using Single-Cell RNA Sequencing: Computational Approaches.
In Methods in molecular biology (Clifton, N.J.)
Butrus Salwan, Sagireddy Srikant, Yan Wenjun, Shekhar Karthik
2023
Machine learning, Optic nerve crush, Retinal ganglion cells, Single-cell RNA-sequencing, Supervised classification
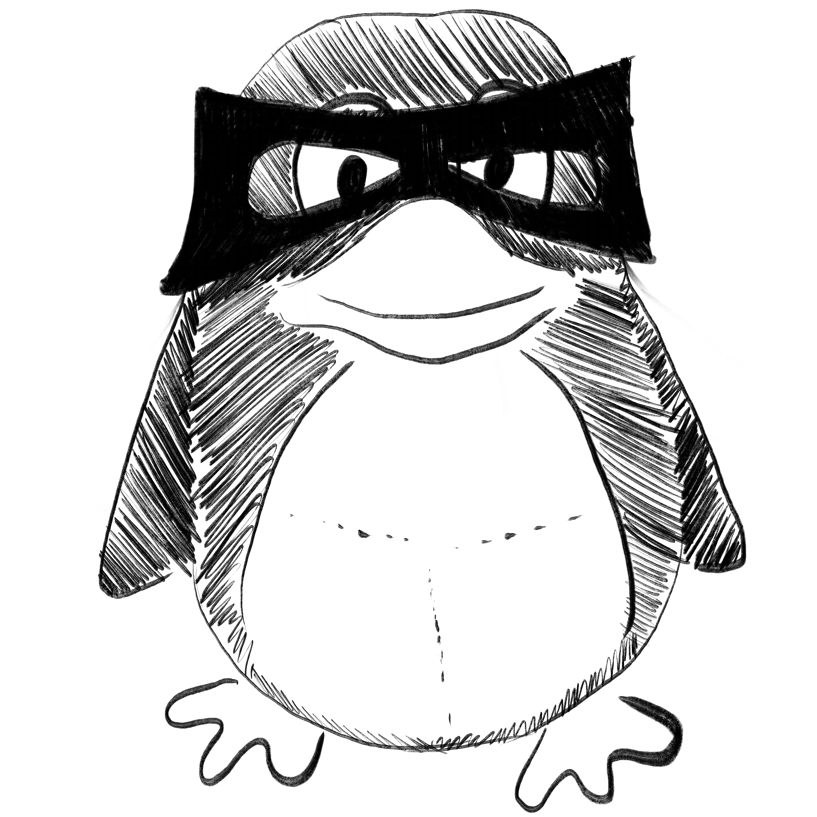
Using serial dependence to predict confidence across observers and cognitive domains.
In Psychonomic bulletin & review
Mei Ning, Rahnev Dobromir, Soto David
2023-Mar-07
Cognition, Confidence, Machine learning, Memory, Metacognition, Perception
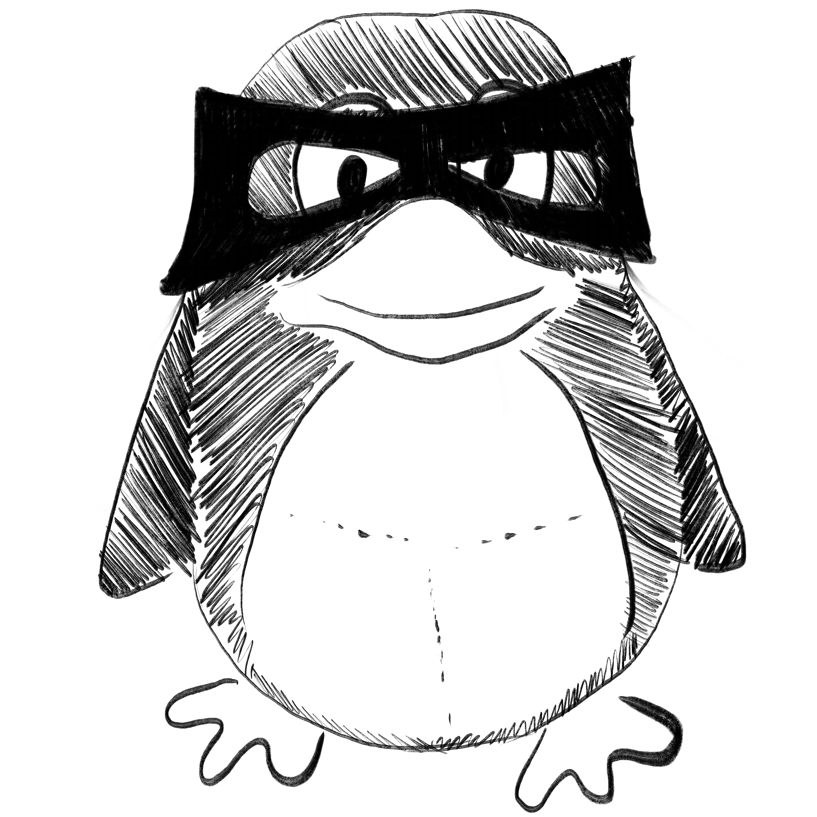
Extreme Learning Machine (ELM) Method for Classification of Preschool Children Brain Imaging.
In Journal of autism and developmental disorders ; h5-index 76.0
Li Deming, De Li, Keqing Li, Gjoni Gazmir
2023-Mar-07
Deep learning, Image classification, Neural networks, Tumor detection
Weekly Summary
Receive a weekly summary and discussion of the top papers of the week by leading researchers in the field.