Receive a weekly summary and discussion of the top papers of the week by leading researchers in the field.
Using classification trees to identify psychotherapy patients at risk for poor treatment adherence.
In Psychotherapy research : journal of the Society for Psychotherapy Research
Regan Timothy, McCredie Morgan N, Harris Bethany, Clark Shaunna
2023-Mar-07
attendance, classification trees, dropout, machine learning, psychotherapy
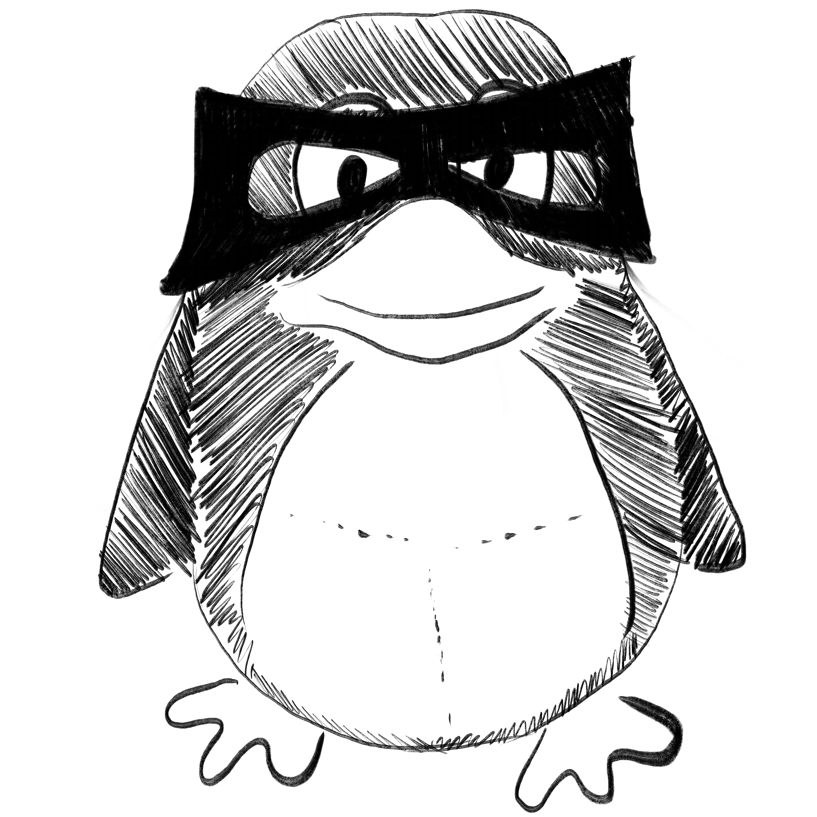
LSTM based stock prediction using weighted and categorized financial news.
In PloS one ; h5-index 176.0
Usmani Shazia, Shamsi Jawwad A
2023
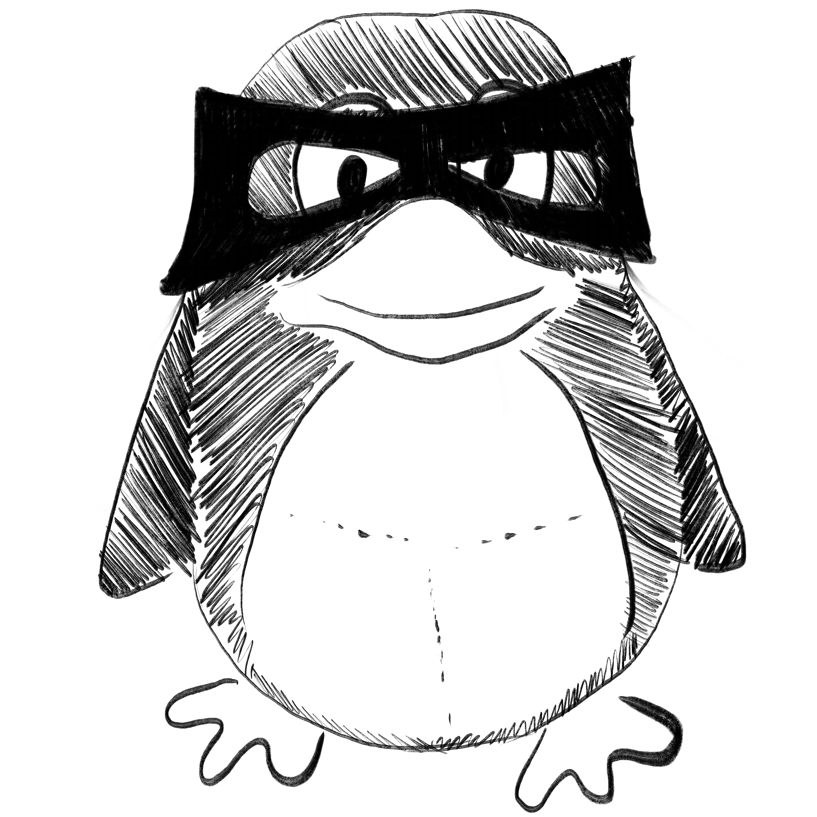
Can individual subjective confidence in training questions predict group performance in test questions?
In PloS one ; h5-index 176.0
Shirasuna Masaru, Honda Hidehito
2023
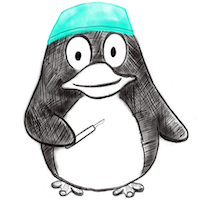
Can a Deep Learning Algorithm Improve Detection of Occult Scaphoid Fractures in Plain Radiographs? A Clinical Validation Study.
In Clinical orthopaedics and related research ; h5-index 71.0
BACKGROUND :
QUESTIONS/PURPOSES :
METHODS :
RESULTS :
CONCLUSION :
LEVEL OF EVIDENCE :
Yoon Alfred P, Chung William T, Wang Chien-Wei, Kuo Chang-Fu, Lin Chihung, Chung Kevin C
2023-Mar-07
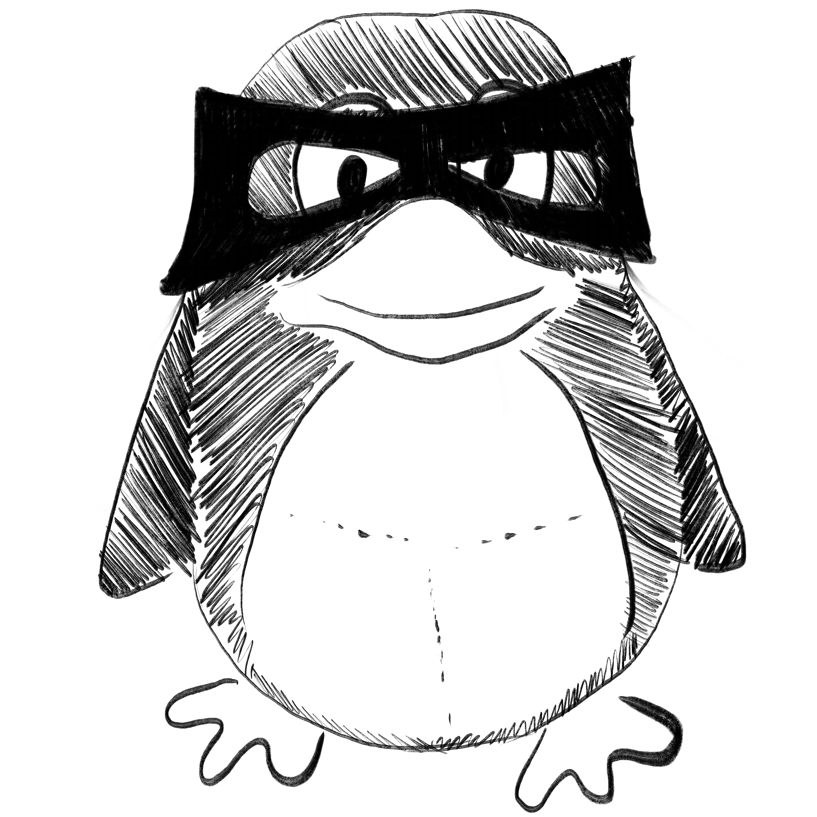
Effects of Antidepressants on COVID Outcome: A Retrospective Study on Large Scale Electronic Health Record Data.
In Interactive journal of medical research
BACKGROUND :
OBJECTIVE :
METHODS :
RESULTS :
CONCLUSIONS :
Rahman Md Mahmudur, Mahi Atqiya Munawara, Melamed Rachel D, Alam Mohammad Arif Ul
2023-Mar-05
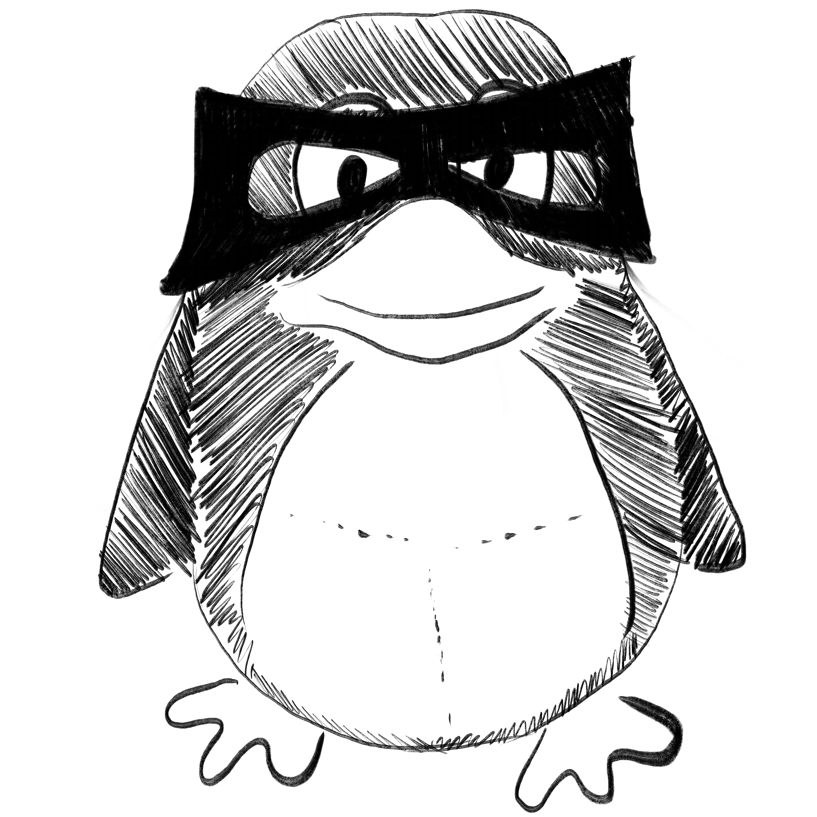
Development and validation of a respiratory-responsive vocal biomarker-based tool for generalizable detection of respiratory impairment: independent case-control studies in multiple respiratory conditions including asthma, chronic obstructive pulmonary disease, and COVID-19.
In Journal of medical Internet research ; h5-index 88.0
BACKGROUND :
OBJECTIVE :
METHODS :
RESULTS :
CONCLUSIONS :
CLINICALTRIAL :
Kaur Savneet, Larsen Erik, Harper James, Purandare Bharat, Uluer Ahmet, Hasdianda Mohammad Adrian, Umale Nikita, Killeen James, Castillo Edward, Jariwala Sunit
2023-Feb-28
Weekly Summary
Receive a weekly summary and discussion of the top papers of the week by leading researchers in the field.