Receive a weekly summary and discussion of the top papers of the week by leading researchers in the field.
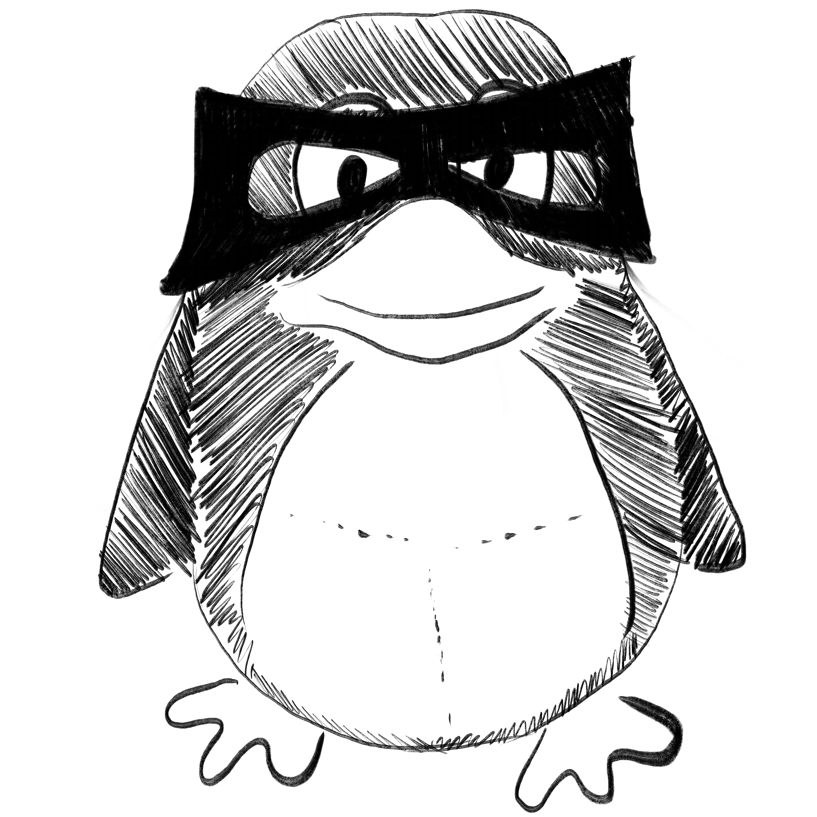
EG-TransUNet: a transformer-based U-Net with enhanced and guided models for biomedical image segmentation.
In BMC bioinformatics
Pan Shaoming, Liu Xin, Xie Ningdi, Chong Yanwen
2023-Mar-07
Channel spatial attention, Medical image segmentation, Progressive enhancement module, Self-attention, Semantic guidance attention, Transformer
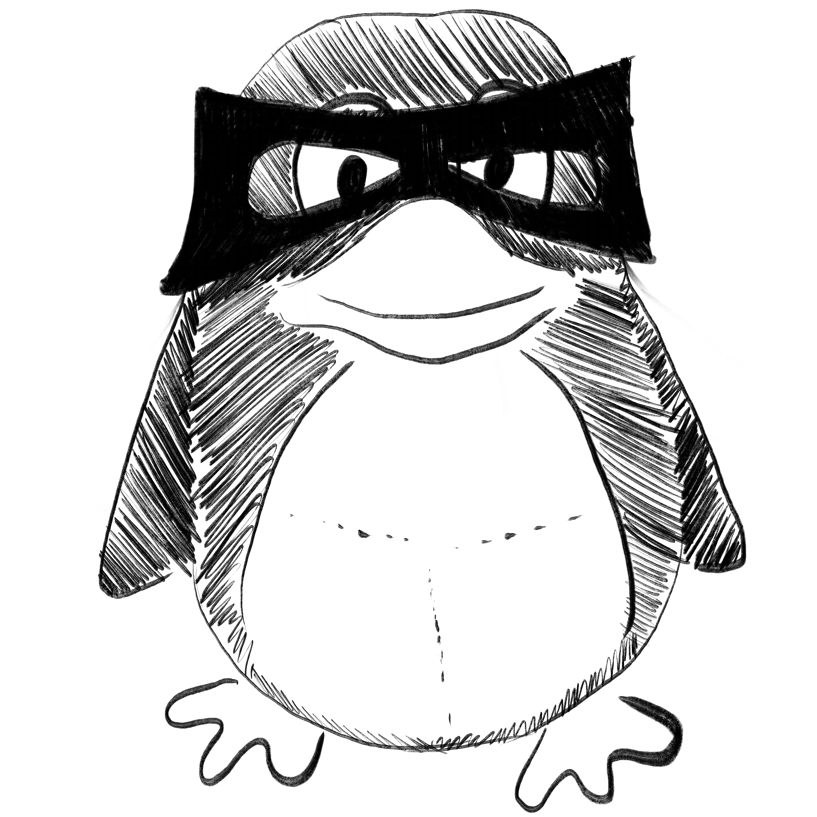
Deep learning for improving PET/CT attenuation correction by elastic registration of anatomical data.
In European journal of nuclear medicine and molecular imaging ; h5-index 66.0
BACKGROUND :
PURPOSE :
MATERIALS AND METHODS :
RESULTS :
CONCLUSION :
Schaefferkoetter Joshua, Shah Vijay, Hayden Charles, Prior John O, Zuehlsdorff Sven
2023-Mar-08
Artificial intelligence, Attenuation correction, Deep learning, Elastic registration, PET/CT
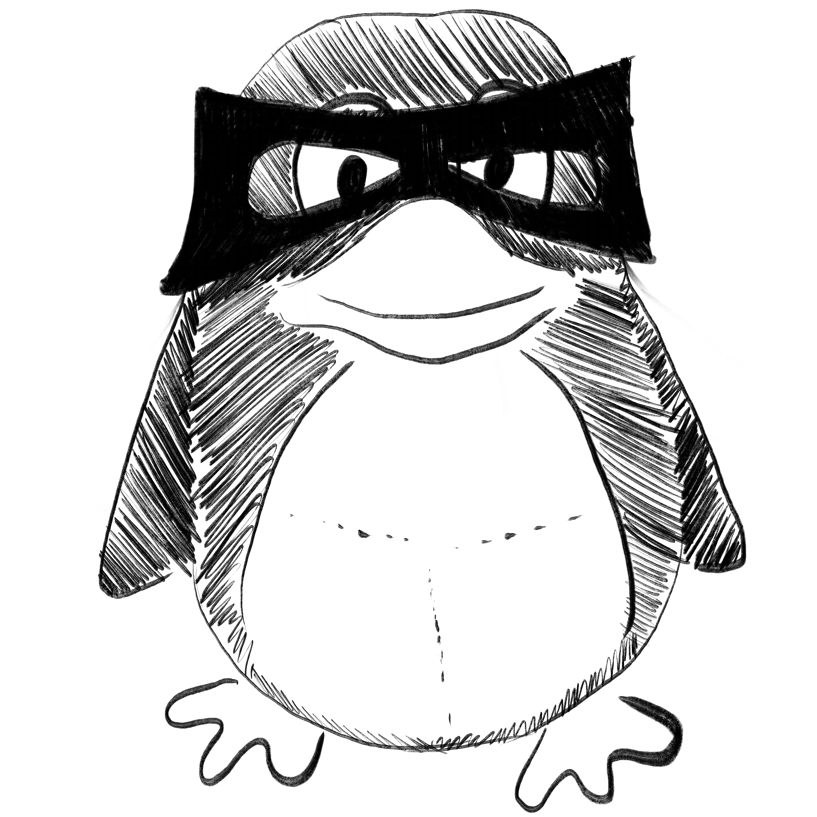
Noble metal catalyst detection in rocks using machine-learning: The future to low-cost, green energy materials?
In Scientific reports ; h5-index 158.0
Ifandi Elena, Lai Daphne Teck Ching, Kalaitzidis Stavros, Abu Bakar Muhammad Saifullah, Grammatikopoulos Tassos, Lai Chun-Kit, Tsikouras Basilios
2023-Mar-07
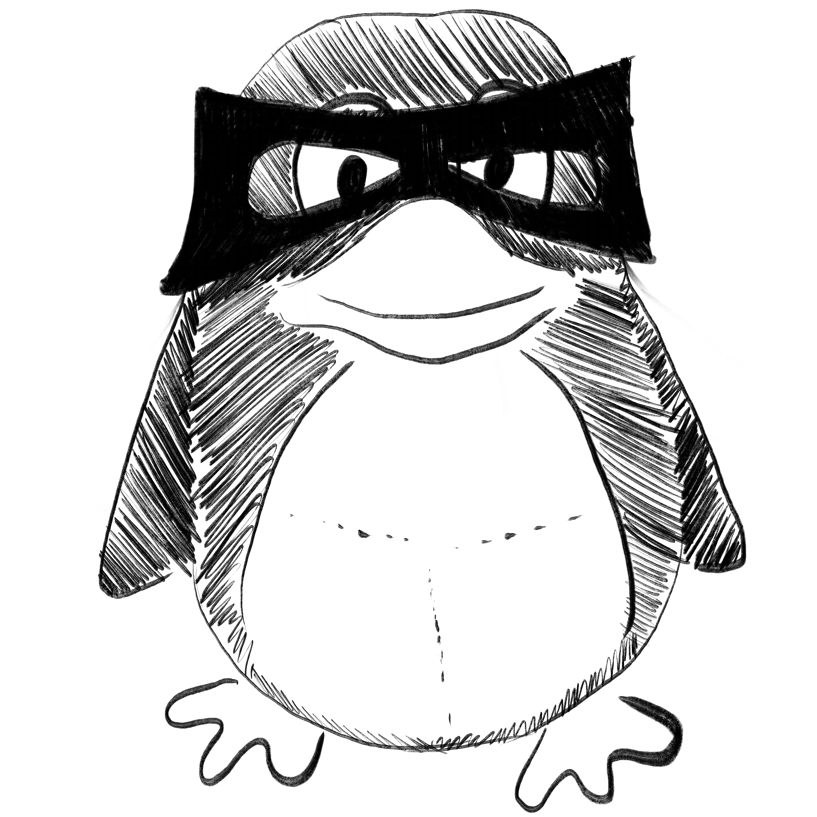
Differentially expressed discriminative genes and significant meta-hub genes based key genes identification for hepatocellular carcinoma using statistical machine learning.
In Scientific reports ; h5-index 158.0
Hasan Md Al Mehedi, Maniruzzaman Md, Shin Jungpil
2023-Mar-07
A transparent artificial intelligence framework to assess lung disease in pulmonary hypertension.
In Scientific reports ; h5-index 158.0
Mamalakis Michail, Dwivedi Krit, Sharkey Michael, Alabed Samer, Kiely David, Swift Andrew J
2023-Mar-07
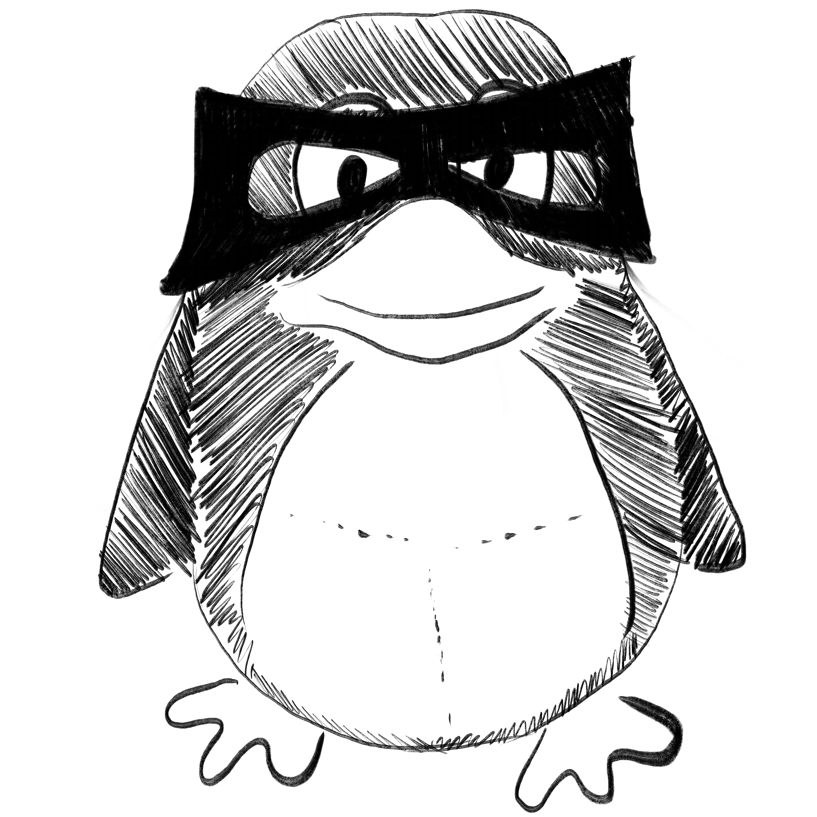
Key therapeutic targets implicated at the early stage of hepatocellular carcinoma identified through machine-learning approaches.
In Scientific reports ; h5-index 158.0
Hosseiniyan Khatibi Seyed Mahdi, Najjarian Farima, Homaei Rad Hamed, Ardalan Mohammadreza, Teshnehlab Mohammad, Zununi Vahed Sepideh, Pirmoradi Saeed
2023-Mar-07
Weekly Summary
Receive a weekly summary and discussion of the top papers of the week by leading researchers in the field.