Receive a weekly summary and discussion of the top papers of the week by leading researchers in the field.
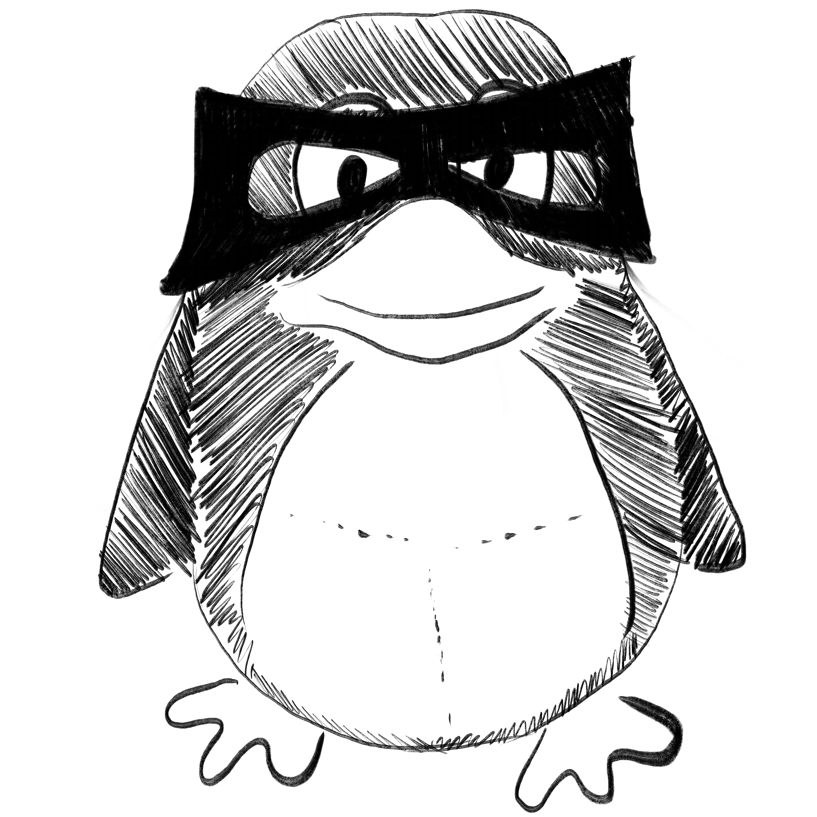
Artificial Intelligence Screening of Medical School Applications: Development and Validation of a Machine-Learning Algorithm.
In Academic medicine : journal of the Association of American Medical Colleges
PURPOSE :
METHOD :
RESULTS :
CONCLUSIONS :
Triola Marc M, Reinstein Ilan, Marin Marina, Gillespie Colleen, Abramson Steven, Grossman Robert I, Rivera Rafael
2023-Mar-06
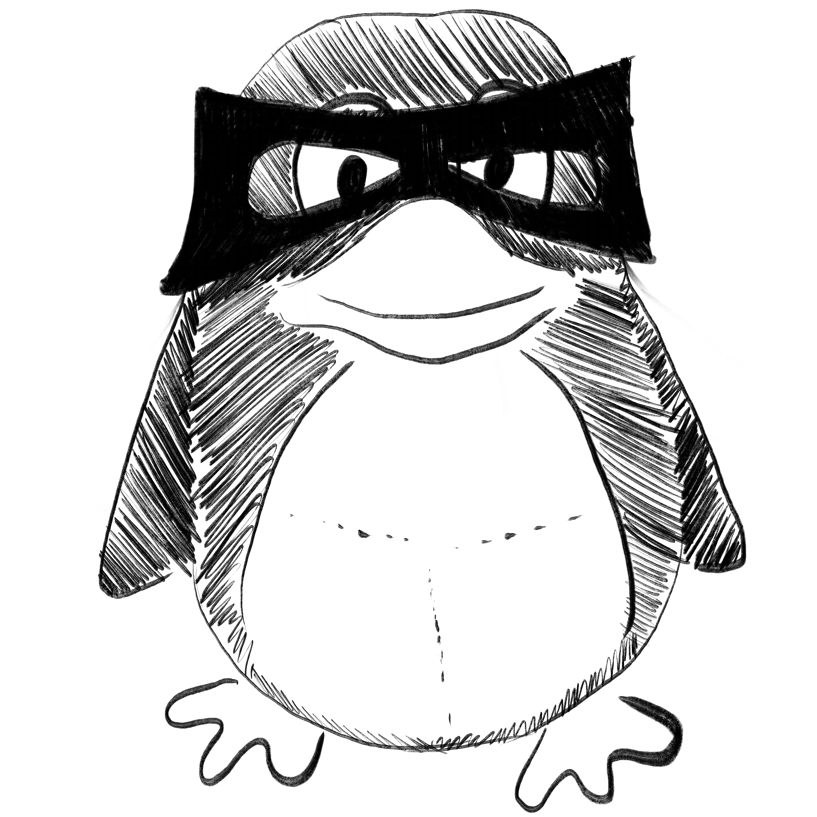
Prediction of septic and hypovolemic shock in intensive care unit patients using machine learning.
In Revista Brasileira de terapia intensiva
OBJECTIVE :
METHODS :
RESULTS :
CONCLUSION :
Pessoa Stela Mares Brasileiro, Oliveira Bianca Silva de Sousa, Santos Wendy Gomes Dos, Oliveira Augusto Novais Macedo, Camargo Marianne Silveira, Matos Douglas Leandro Aparecido Barbosa de, Silva Miquéias Martins Lima, Medeiros Carolina Cintra de Queiroz, Coelho Cláudia Soares de Sousa, Andrade Neto José de Souza, Mistro Sóstenes
2022
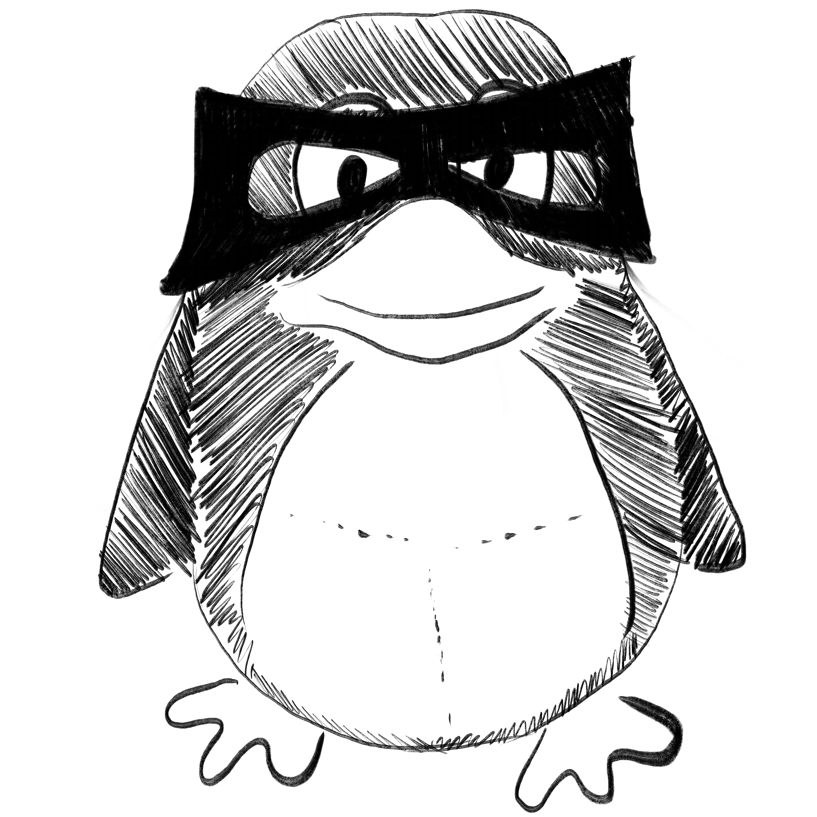
A self-attention-based neural network for three-dimensional multivariate modeling and its skillful ENSO predictions.
In Science advances
Zhou Lu, Zhang Rong-Hua
2023-Mar-10
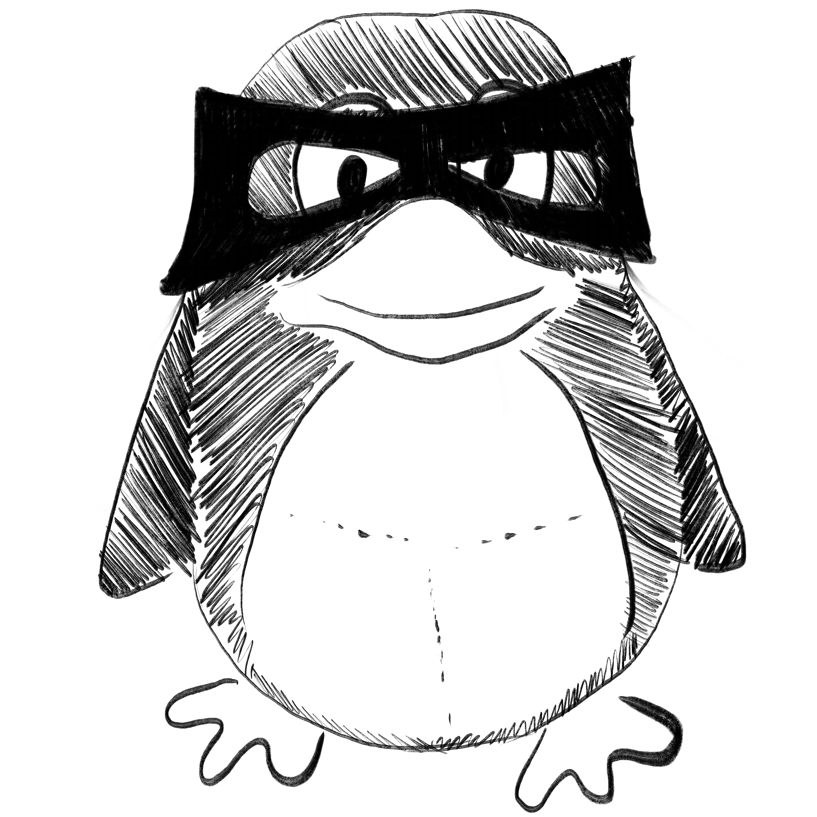
Sloppiness: Fundamental study, new formalism and its application in model assessment.
In PloS one ; h5-index 176.0
Jagadeesan Prem, Raman Karthik, Tangirala Arun K
2023
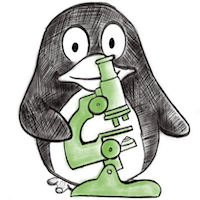
Detection of malignancy in whole slide images of endometrial cancer biopsies using artificial intelligence.
In PloS one ; h5-index 176.0
Fell Christina, Mohammadi Mahnaz, Morrison David, Arandjelović Ognjen, Syed Sheeba, Konanahalli Prakash, Bell Sarah, Bryson Gareth, Harrison David J, Harris-Birtill David
2023
A Comparison of Single and Combined Schemes of Asia-Pacific Colorectal Screening, Faecal Immunochemical and Stool Deoxyribonucleic Acid Testing for Community Colorectal Cancer Screening.
In Journal of multidisciplinary healthcare
OBJECTIVE :
METHODS :
RESULTS :
CONCLUSION :
Ze Yuan, Tu Huiming, Zhang Lin, Bai Yu, Ren Yilin, Chen Xin, Xue Yuzheng, Sun Renjuan, Yang Yuling, Yang Jie, Zhou Xuan, Liu Li
2023
colonoscopy, colorectal cancer, faecal immunochemical testing, primary screening, stool DNA test
Weekly Summary
Receive a weekly summary and discussion of the top papers of the week by leading researchers in the field.