Receive a weekly summary and discussion of the top papers of the week by leading researchers in the field.
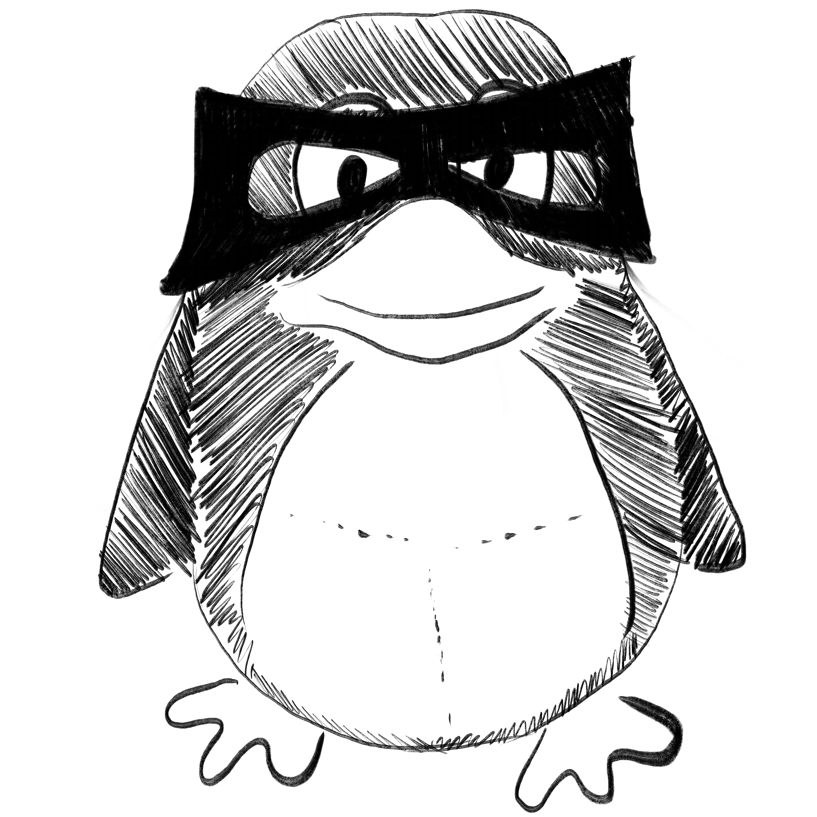
Predicting Progression from Normal to MCI and from MCI to AD Using Clinical Variables in the National Alzheimer's Coordinating Center Uniform Data Set Version 3: Application of Machine Learning Models and a Probability Calculator.
In The journal of prevention of Alzheimer's disease
Pang Y, Kukull W, Sano M, Albin R L, Shen C, Zhou J, Dodge H H
2023
AD, Alzheimer’s disease, MCI, MCI conversion, National Alzheimer’s Coordinating Center (NACC), PET amyloid, dementia, machine learning
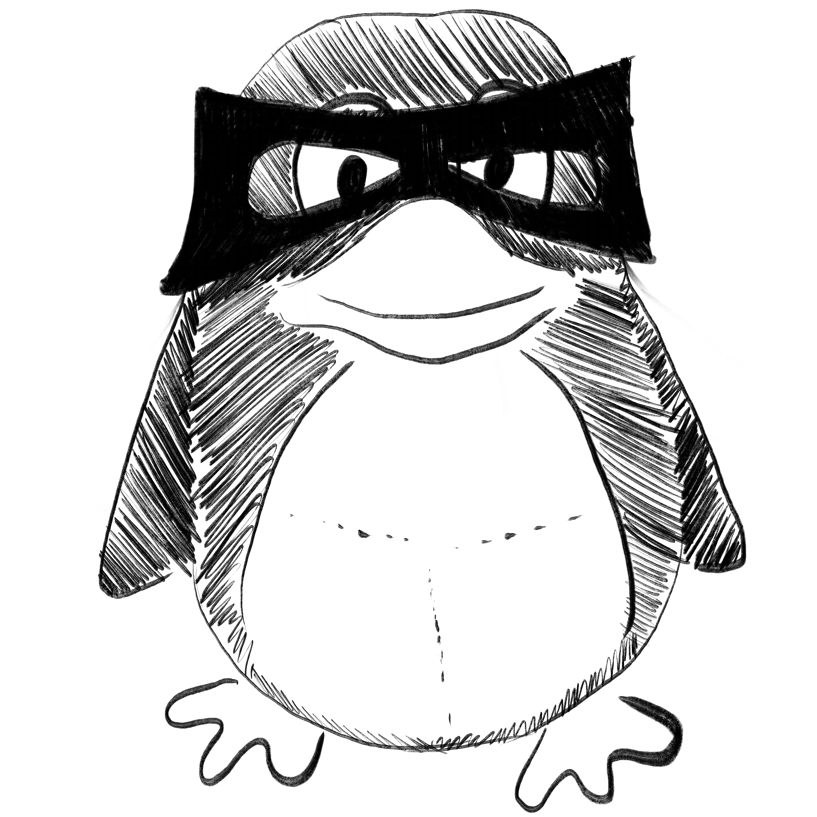
A self-adaptive hardware with resistive switching synapses for experience-based neurocomputing.
In Nature communications ; h5-index 260.0
Bianchi S, Muñoz-Martin I, Covi E, Bricalli A, Piccolboni G, Regev A, Molas G, Nodin J F, Andrieu F, Ielmini D
2023-Mar-21
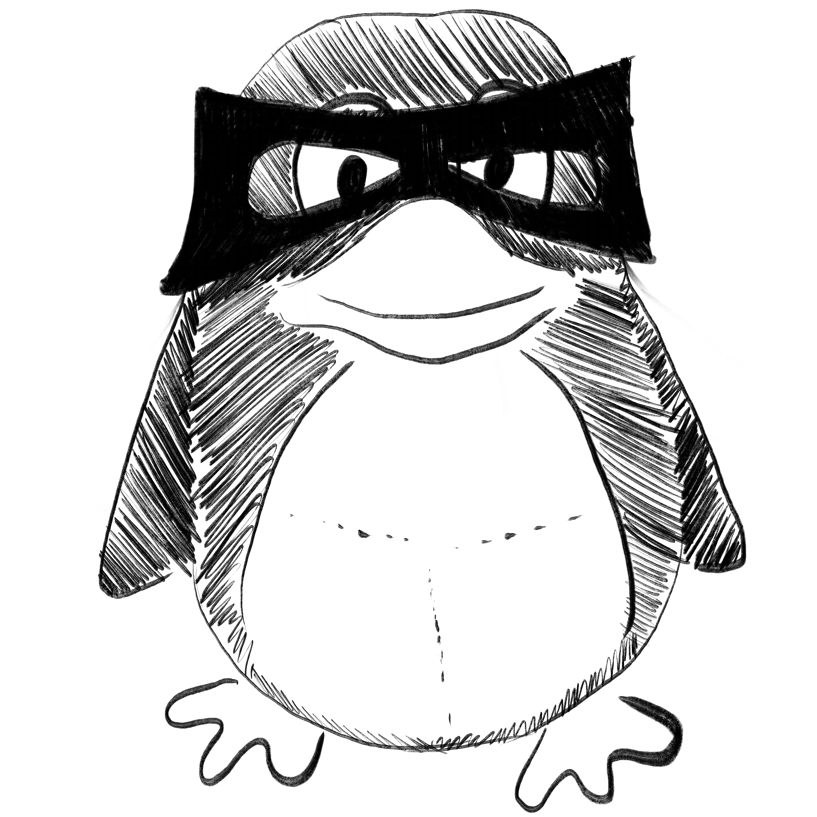
Understanding Prospective Physicians' Intention to Use Artificial Intelligence in Their Future Medical Practice: Configurational Analysis.
In JMIR medical education
BACKGROUND :
OBJECTIVE :
METHODS :
RESULTS :
CONCLUSIONS :
Wagner Gerit, Raymond Louis, Paré Guy
2023-Mar-22
artificial intelligence, attitudes and beliefs, behavioral intentions, fsQCA, fuzzy-set qualitative comparative analysis, knowledge and experience, medical education
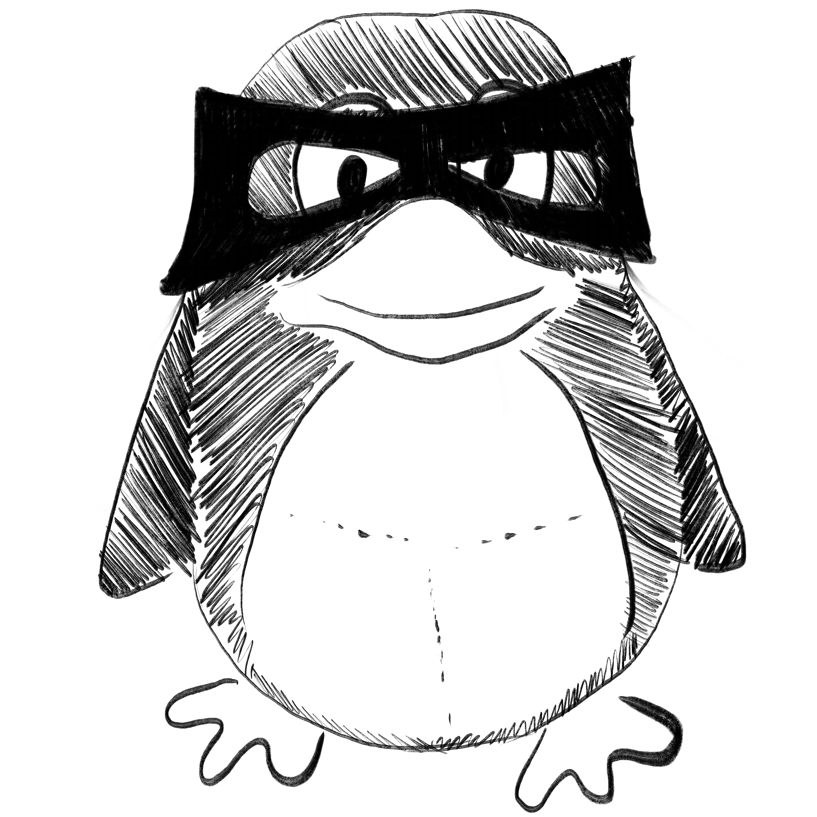
Human Behavior in the Time of COVID-19: Learning from Big Data
ArXiv Preprint
Hanjia Lyu, Arsal Imtiaz, Yufei Zhao, Jiebo Luo
2023-03-23
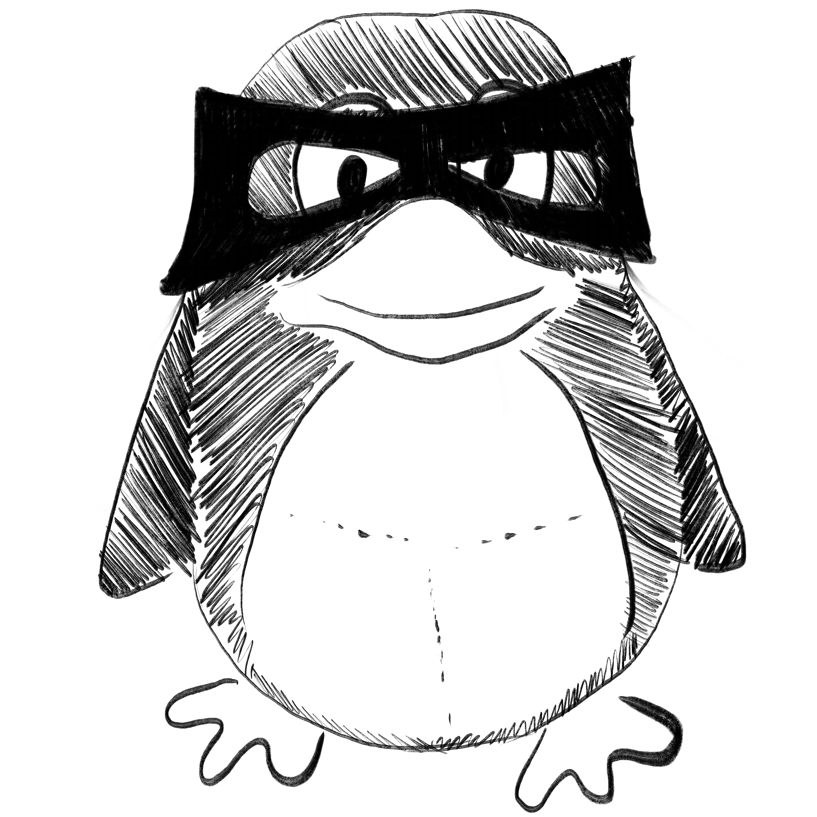
Utility of Environmental Complexity as a Predictor of Alzheimer's Disease Diagnosis: A Big-Data Machine Learning Approach.
In The journal of prevention of Alzheimer's disease
BACKGROUND :
METHODS :
RESULTS :
CONCLUSION :
Yuan M, Kennedy K M
2023
Alzheimer’s disease, cognitive map, environmental complexity, geospatial mapping, neural network modelling
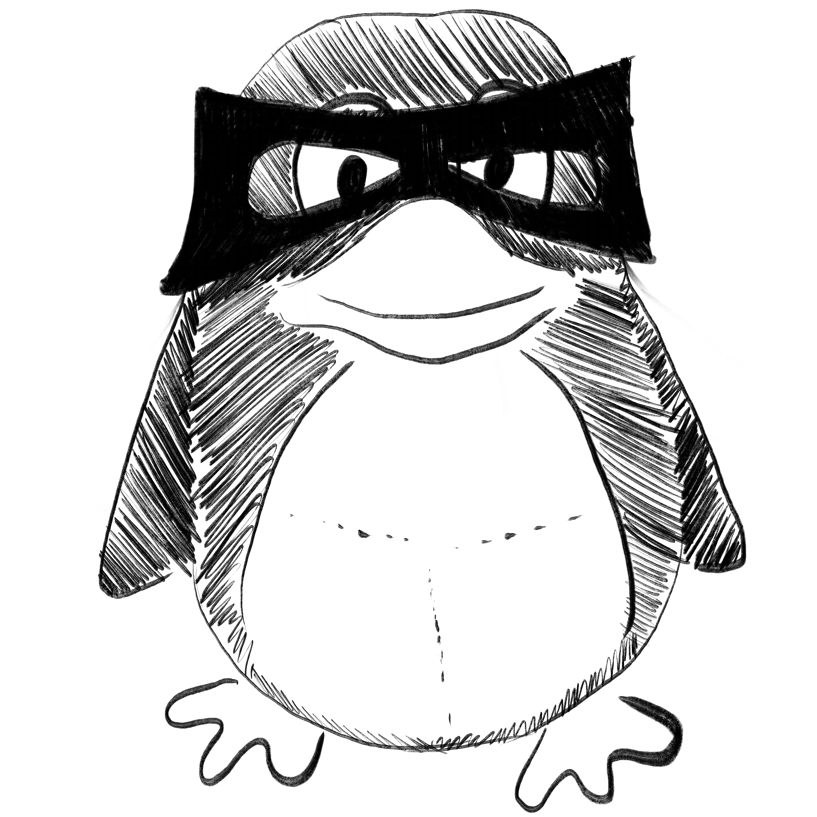
Poincaré maps for visualization of large protein families.
In Briefings in bioinformatics
Susmelj Anna Klimovskaia, Ren Yani, Vander Meersche Yann, Gelly Jean-Christophe, Galochkina Tatiana
2023-Mar-22
data visualization, dimensionality reduction, machine learning, multiple sequence alignment, protein evolution, protein function, protein sequence, sequence similarity
Weekly Summary
Receive a weekly summary and discussion of the top papers of the week by leading researchers in the field.