Receive a weekly summary and discussion of the top papers of the week by leading researchers in the field.
Validation of an automated machine learning algorithm for the detection and analysis of cerebral aneurysms.
In Journal of neurosurgery ; h5-index 64.0
OBJECTIVE :
METHODS :
RESULTS :
CONCLUSIONS :
Colasurdo Marco, Shalev Daphna, Robledo Ariadna, Vasandani Viren, Luna Zean Aaron, Rao Abhijit S, Garcia Roberto, Edhayan Gautam, Srinivasan Visish M, Sheth Sunil A, Donner Yoni, Bibas Orin, Limzider Nicole, Shaltoni Hashem, Kan Peter
2023-Mar-03
CT angiography, artificial intelligence, cerebral aneurysm, diagnostic technique, endovascular neurosurgery, technology, vascular disorders
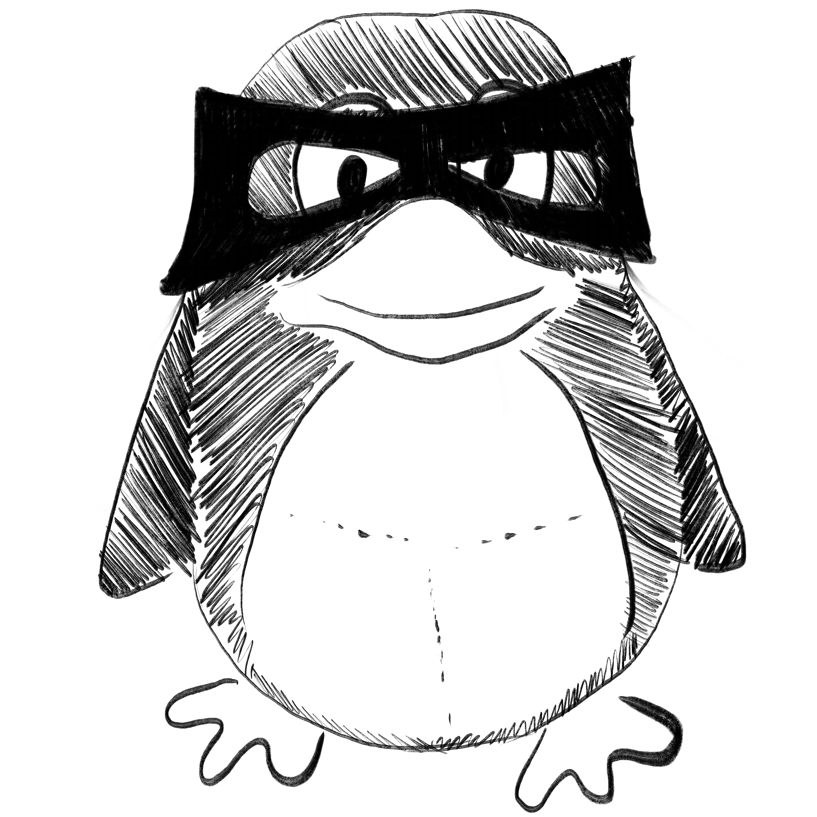
Multiomics and machine-learning identify novel transcriptional and mutational signatures in amyotrophic lateral sclerosis.
In Brain : a journal of neurology
Catanese Alberto, Rajkumar Sandeep, Sommer Daniel, Masrori Pegah, Hersmus Nicole, Van Damme Philip, Witzel Simon, Ludolph Albert, Ho Ritchie, Boeckers Tobias M, Mulaw Medhanie
2023-Mar-08
ALS, deep learning, motor neurons, omics
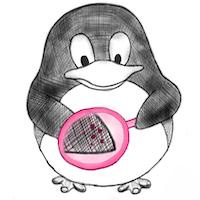
A neutral comparison of statistical methods for analyzing longitudinally measured ordinal outcomes in rare diseases.
In Biometrical journal. Biometrische Zeitschrift
Geroldinger Martin, Verbeeck Johan, Thiel Konstantin E, Molenberghs Geert, Bathke Arne C, Laimer Martin, Zimmermann Georg
2023-Mar-08
Epidermolysis Bullosa simplex, generalized pairwise comparison (GPC), neutral comparison, nonparametric marginal model (nparLD), repeated measures
Prediction of the sarcopenia in peritoneal dialysis using simple clinical information: A machine learning-based model.
In Seminars in dialysis
INTRODUCTION :
METHODS :
RESULT :
CONCLUSION :
Wu Jiaying, Lin Shuangxiang, Guan Jichao, Wu Xiujuan, Ding Miaojia, Shen Shuijuan
2023-Mar-08
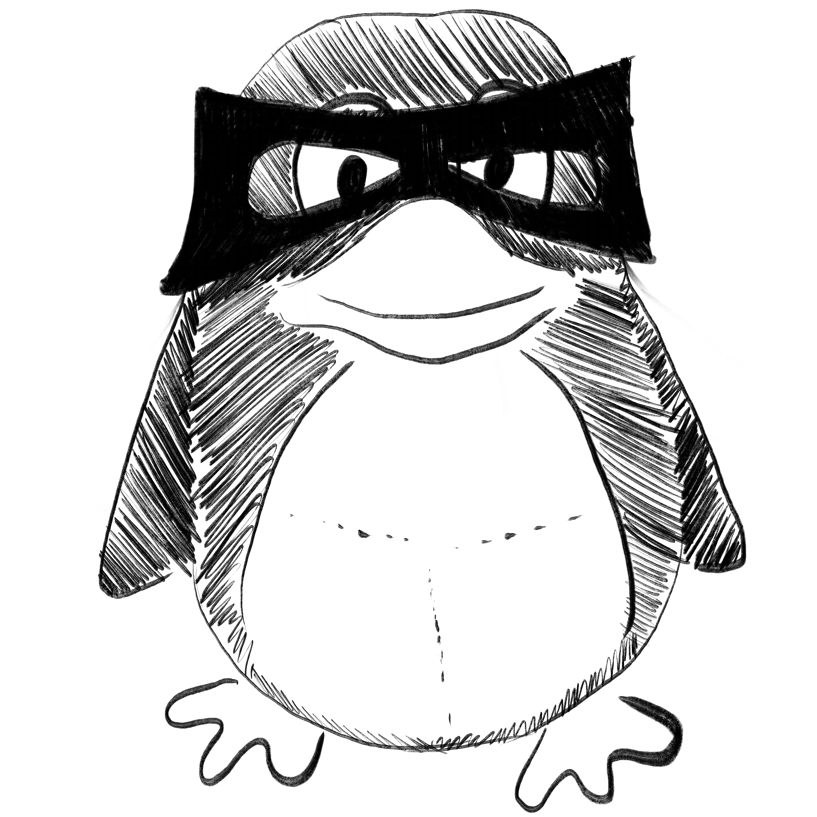
ECG signal feature extraction trends in methods and applications.
In Biomedical engineering online
Singh Anupreet Kaur, Krishnan Sridhar
2023-Mar-08
Artificial intelligence, Digital health, ECG, Feature extraction, Signal analysis, Telehealth
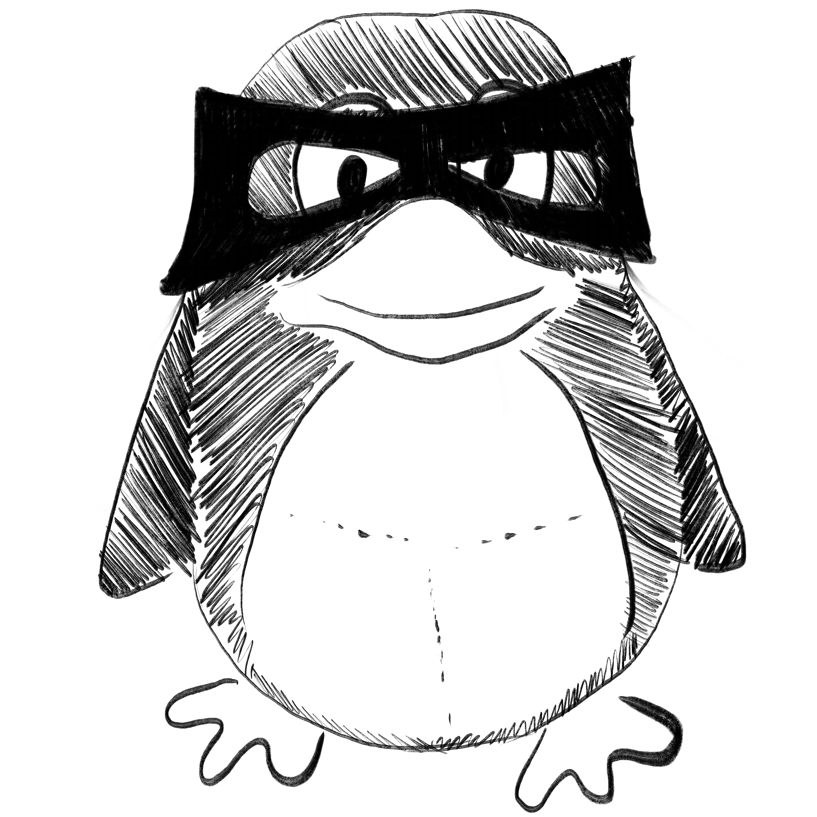
Application of machine learning to identify risk factors of birth asphyxia.
In BMC pregnancy and childbirth ; h5-index 58.0
BACKGROUND :
METHODS :
RESULTS :
CONCLUSION :
Darsareh Fatemeh, Ranjbar Amene, Farashah Mohammadsadegh Vahidi, Mehrnoush Vahid, Shekari Mitra, Jahromi Malihe Shirzadfard
2023-Mar-08
Birth asphyxia, Machine learning, Risk factors
Weekly Summary
Receive a weekly summary and discussion of the top papers of the week by leading researchers in the field.