Receive a weekly summary and discussion of the top papers of the week by leading researchers in the field.
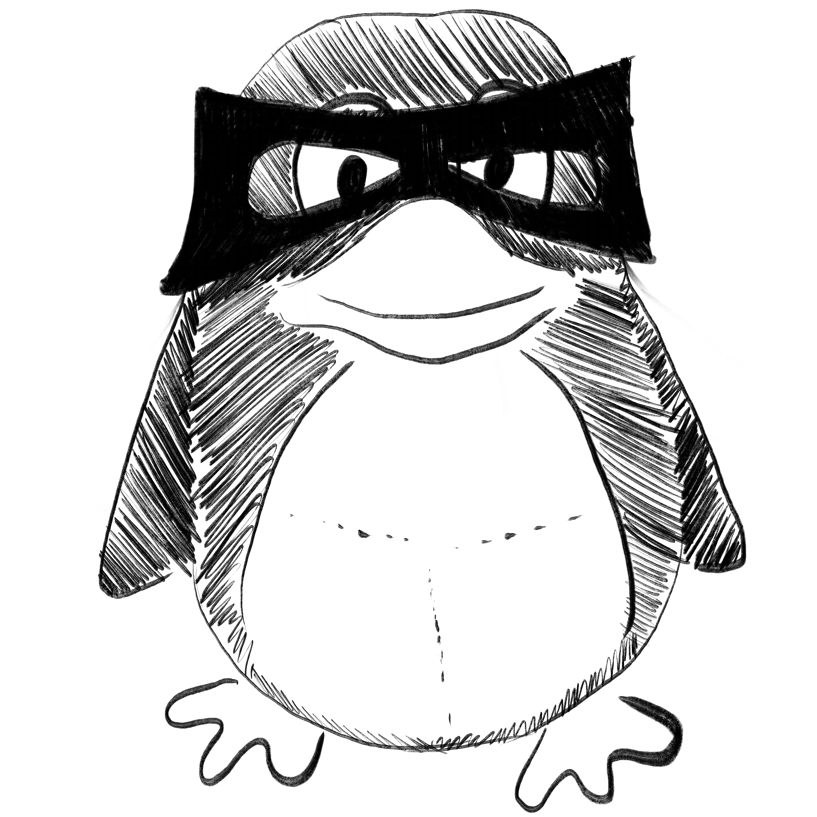
Evaluation of computer aided detection during colonoscopy in the community (AI-SEE): a multicenter randomized clinical trial.
In The American journal of gastroenterology
BACKGROUND :
METHODS :
RESULTS :
CONCLUSIONS :
Wei Mike T, Shankar Uday, Parvin Russell, Hasan Abbas Syed, Chaudhary Sushant, Friedlander Yishai, Friedland Shai
2023-Mar-09
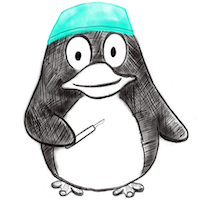
Mechanism of Injury and Age Predict Operative Intervention in Pediatric Perineal Injury.
In Pediatric emergency care
OBJECTIVES :
METHODS :
RESULTS :
CONCLUSIONS :
McLaughlin Christopher J, Martin Kathryn L
2023-Mar-07
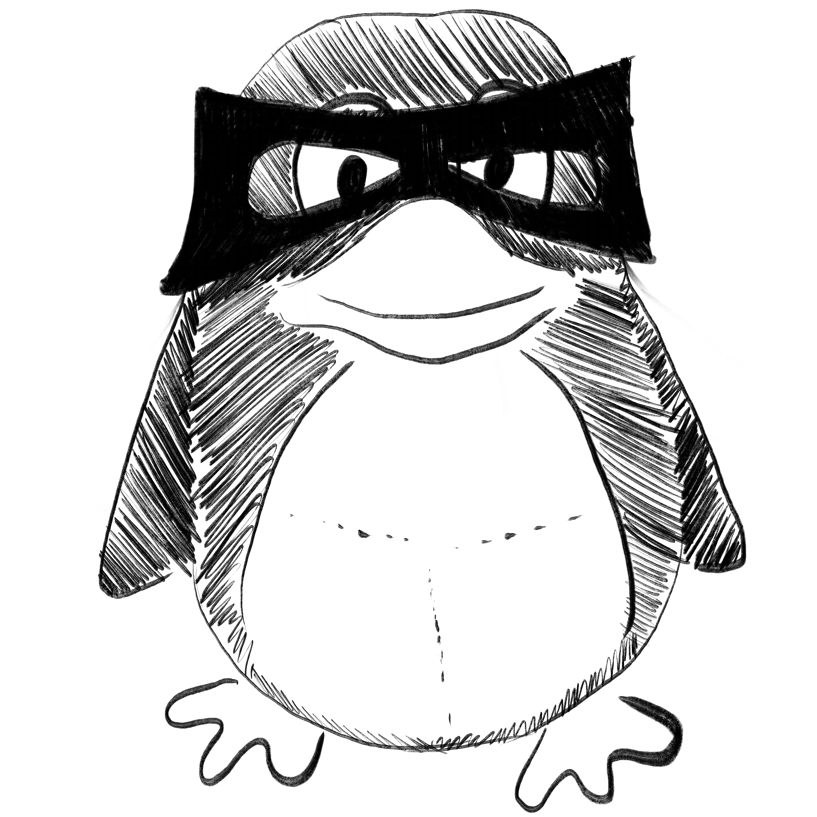
Machine learning quantitatively characterizes the deformation and destruction of explosive molecules.
In Physical chemistry chemical physics : PCCP
Zhang Kaining, Chen Lang, Zhang Teng, Lu Jianying, Liu Danyang, Wu Junying
2023-Mar-09
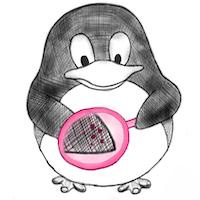
Attitudes Towards Artificial Intelligence Among Dermatologists Working in Saudi Arabia.
In Dermatology practical & conceptual
INTRODUCTION :
OBJECTIVE :
METHODS :
RESULTS :
CONCLUSION :
Al-Ali Fatima, Polesie Sam, Paoli John, Aljasser Mohammed, Salah Louai A
2023-Jan-01
Preoperative prediction of tumor deposits in rectal cancer with clinical-magnetic resonance deep learning-based radiomic models.
In Frontiers in oncology
BACKGROUND :
METHODS :
RESULTS :
CONCLUSIONS :
Fu Chunlong, Shao Tingting, Hou Min, Qu Jiali, Li Ping, Yang Zebin, Shan Kangfei, Wu Meikang, Li Weida, Wang Xuan, Zhang Jingfeng, Luo Fanghong, Zhou Long, Sun Jihong, Zhao Fenhua
2023
deep learning, diffusion-weighted imaging, magnetic resonance imaging, rectal cancer, tumor deposit
COVID-19 imaging, where do we go from here? Bibliometric analysis of medical imaging in COVID-19.
In European radiology ; h5-index 62.0
OBJECTIVES :
METHODS :
RESULTS :
CONCLUSIONS :
Wen Ru, Zhang Mudan, Xu Rui, Gao Yingming, Liu Lin, Chen Hui, Wang Xingang, Zhu Wenyan, Lin Huafang, Liu Chen, Zeng Xianchun
2023-Mar-09
Bibliometrics, COVID-19, CiteSpace, Medical imaging, Treads
Weekly Summary
Receive a weekly summary and discussion of the top papers of the week by leading researchers in the field.