Receive a weekly summary and discussion of the top papers of the week by leading researchers in the field.
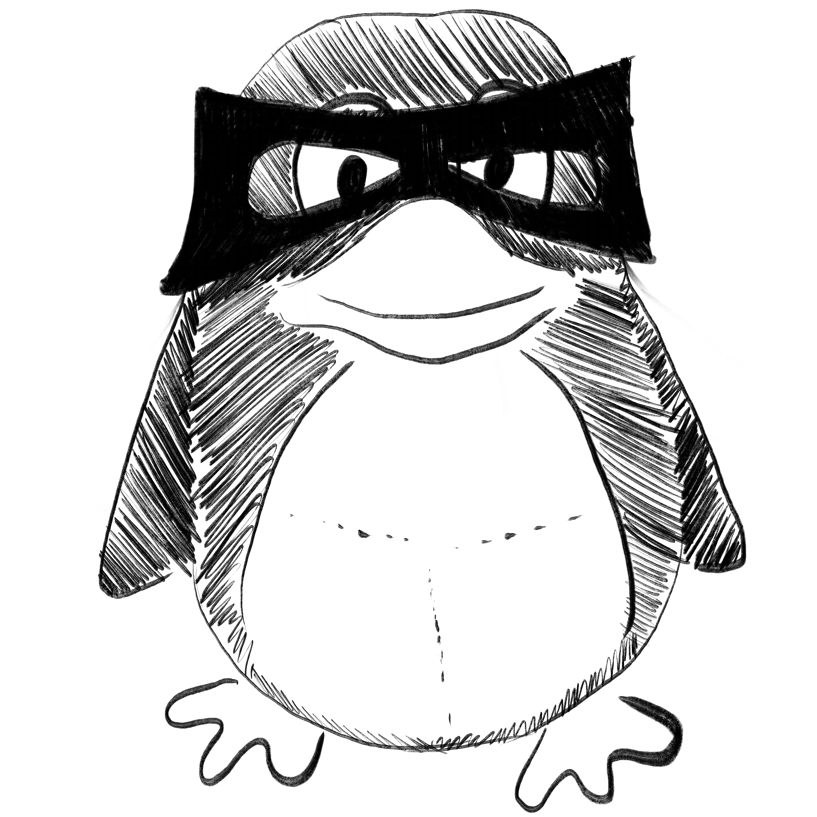
In Situ Spatiotemporal SERS Measurements and Multivariate Analysis of Virally Infected Bacterial Biofilms Using Nanolaminated Plasmonic Crystals.
In ACS sensors
Garg Aditya, Nam Wonil, Wang Wei, Vikesland Peter, Zhou Wei
2023-Mar-09
bacterial biofilms, multivariate analysis, spatiotemporal SERS, surface-enhanced Raman spectroscopy, virus detection
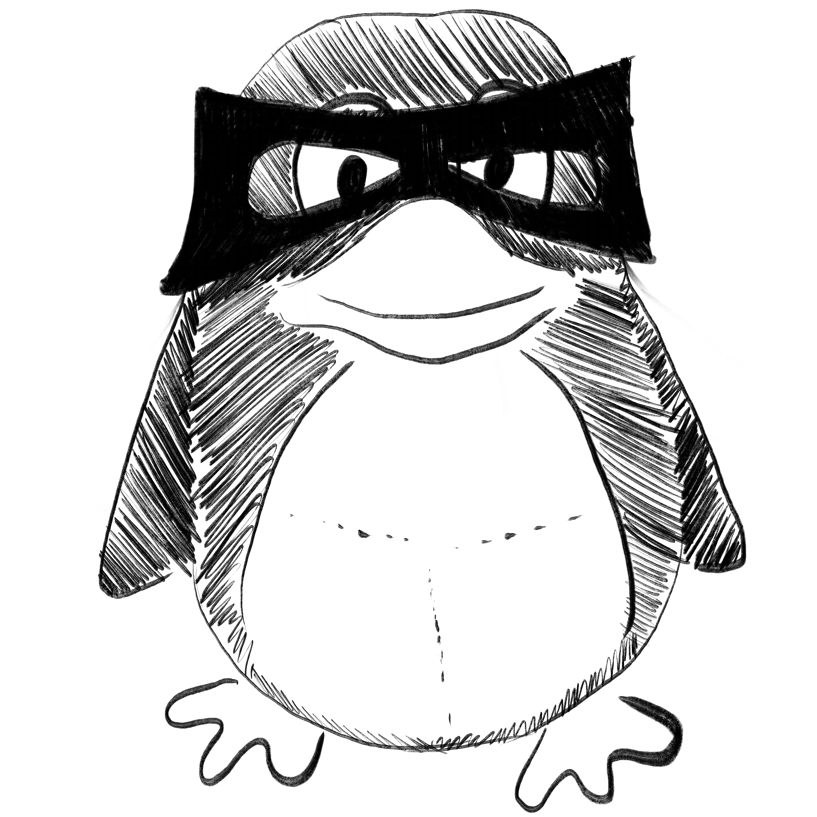
Are Deep Learning Structural Models Sufficiently Accurate for Virtual Screening? Application of Docking Algorithms to AlphaFold2 Predicted Structures.
In Journal of chemical information and modeling
Díaz-Rovira Anna M, Martín Helena, Beuming Thijs, Díaz Lucía, Guallar Victor, Ray Soumya S
2023-Mar-09
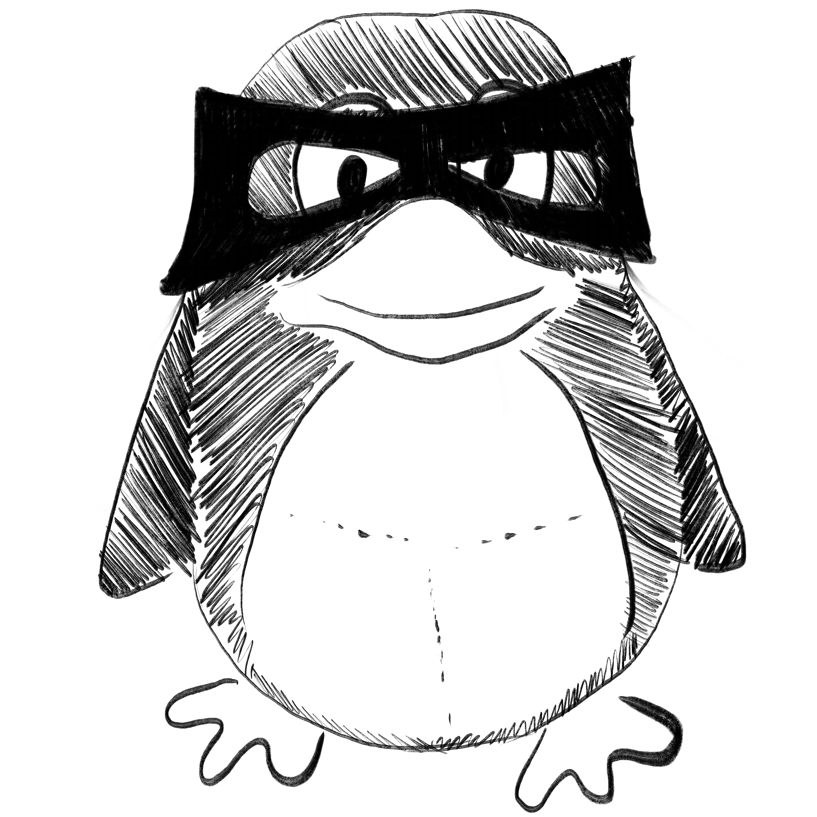
Biochemical identification of prepubertal boys with Klinefelter syndrome by combined reproductive hormone profiling using machine learning.
In Endocrine connections
OBJECTIVE :
METHODS :
RESULTS :
CONCLUSIONS :
Madsen Andre, Juul Anders, Aksglaede Lise
2023-Mar-01
Development and validation of an ensemble machine-learning model for predicting early mortality among patients with bone metastases of hepatocellular carcinoma.
In Frontiers in oncology
PURPOSE :
METHODS :
RESULTS :
CONCLUSIONS :
Long Ze, Yi Min, Qin Yong, Ye Qianwen, Che Xiaotong, Wang Shengjie, Lei Mingxing
2023
bone metastases, early mortality, ensemble model, hepatocellular carcinoma, machine learning
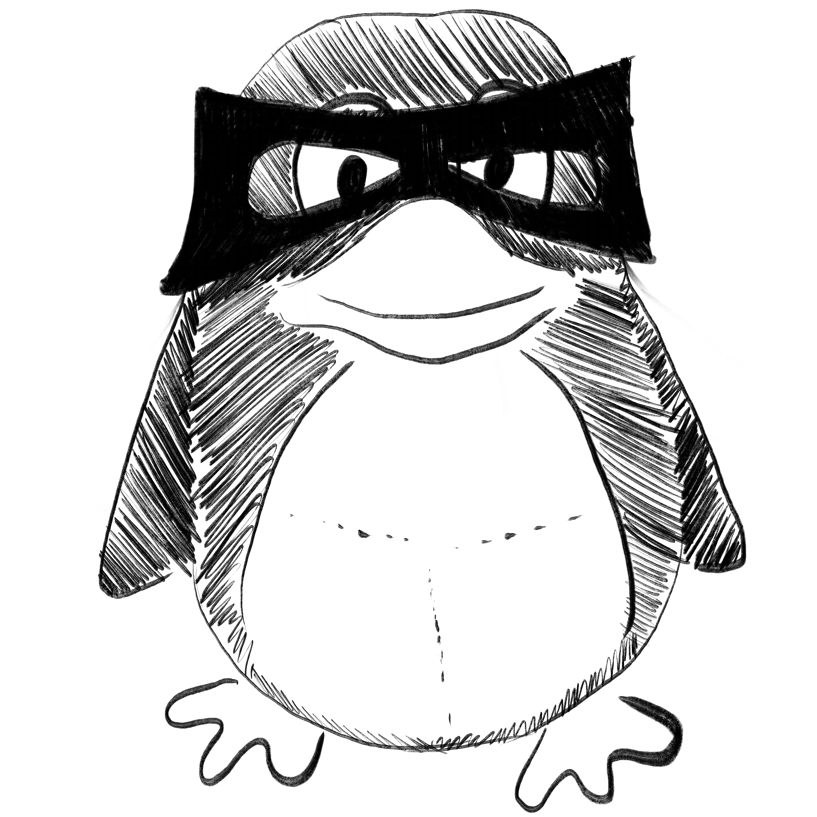
A hybrid CNN and ensemble model for COVID-19 lung infection detection on chest CT scans.
In PloS one ; h5-index 176.0
Akl Ahmed A, Hosny Khalid M, Fouda Mostafa M, Salah Ahmad
2023
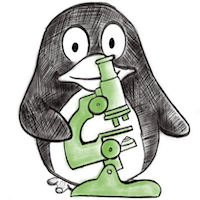
Deep Learning for Predicting Metastasis on Melanoma WSIs
ArXiv Preprint
Christopher Andreassen, Saul Fuster, Helga Hardardottir, Emiel A. M. Janssen, Kjersti Engan
2023-03-10
Weekly Summary
Receive a weekly summary and discussion of the top papers of the week by leading researchers in the field.