Receive a weekly summary and discussion of the top papers of the week by leading researchers in the field.
Liquid Health. Medicine in the age of surveillance capitalism.
In Social science & medicine (1982)
Rubeis Giovanni
2023-Feb-25
Artificial intelligence, Big data, Digital health, Ethics, Surveillance capitalism, Zygmunt bauman
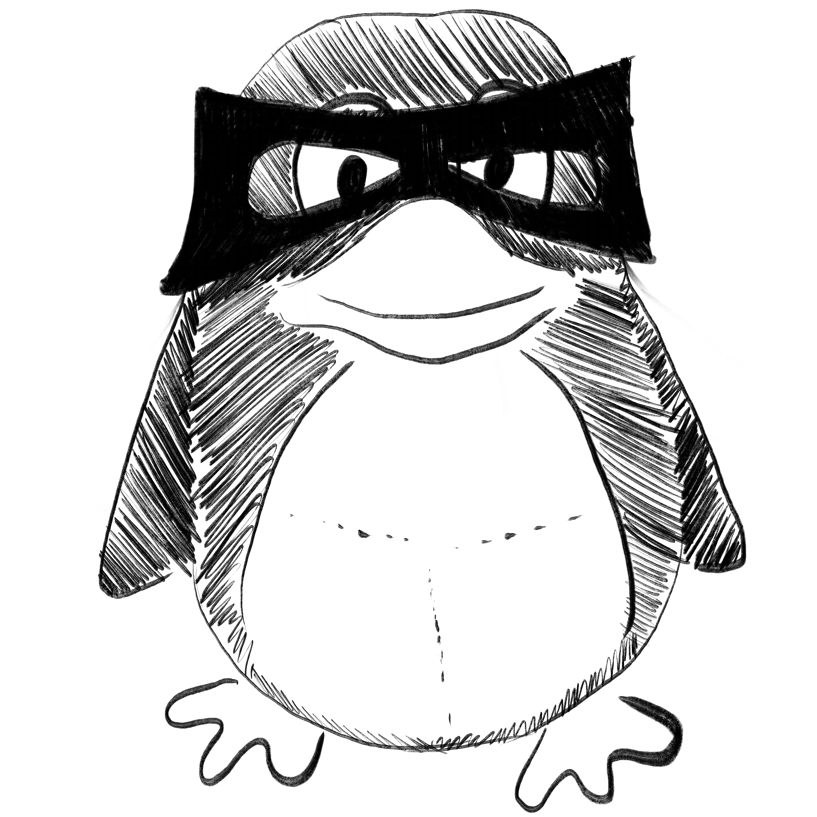
Convolutional neural network classifies visual stimuli from cortical response recorded with wide-field imaging in mice.
In Journal of neural engineering ; h5-index 52.0
De Luca Daniela, Moccia Sara, Lupori Leonardo, Mazziotti Raffaele, Pizzorusso Tommaso, Micera Silvestro
2023-Mar-09
deep learning, optic nerve, visual prostheses, visual stimuli decoding, wide-field imaging
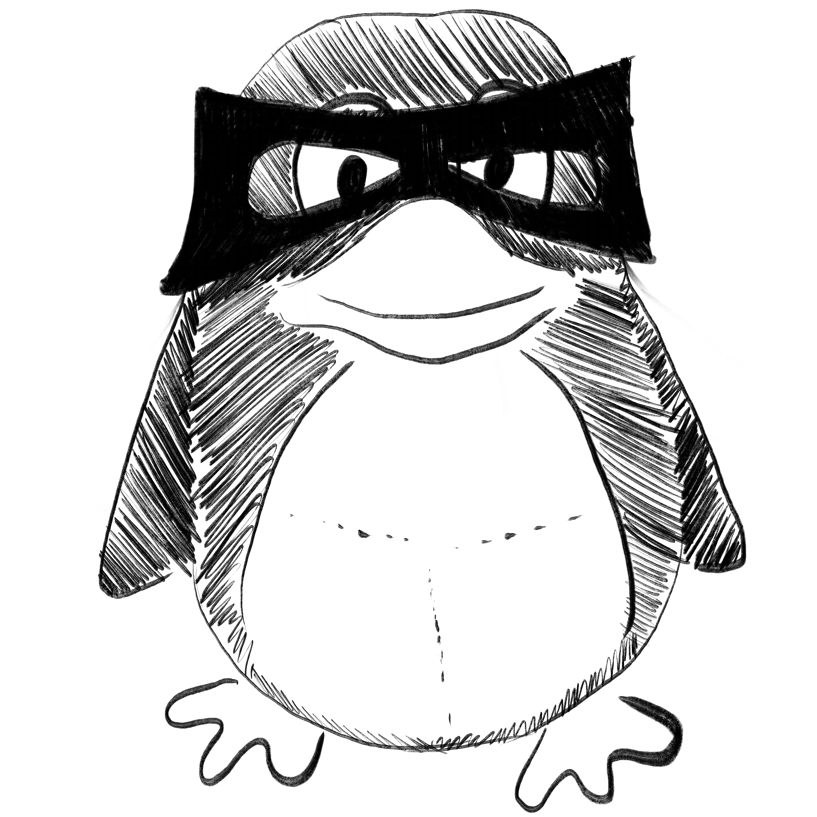
High-Density Guide RNA Tiling and Machine Learning for Designing CRISPR Interference in Synechococcus sp. PCC 7002.
In ACS synthetic biology
Dallo Tessa, Krishnakumar Raga, Kolker Stephanie D, Ruffing Anne M
2023-Mar-09
CRISPRi, Synechococcus, Synechococcus sp. PCC 7002, cyanobacteria, gRNA design, machine learning
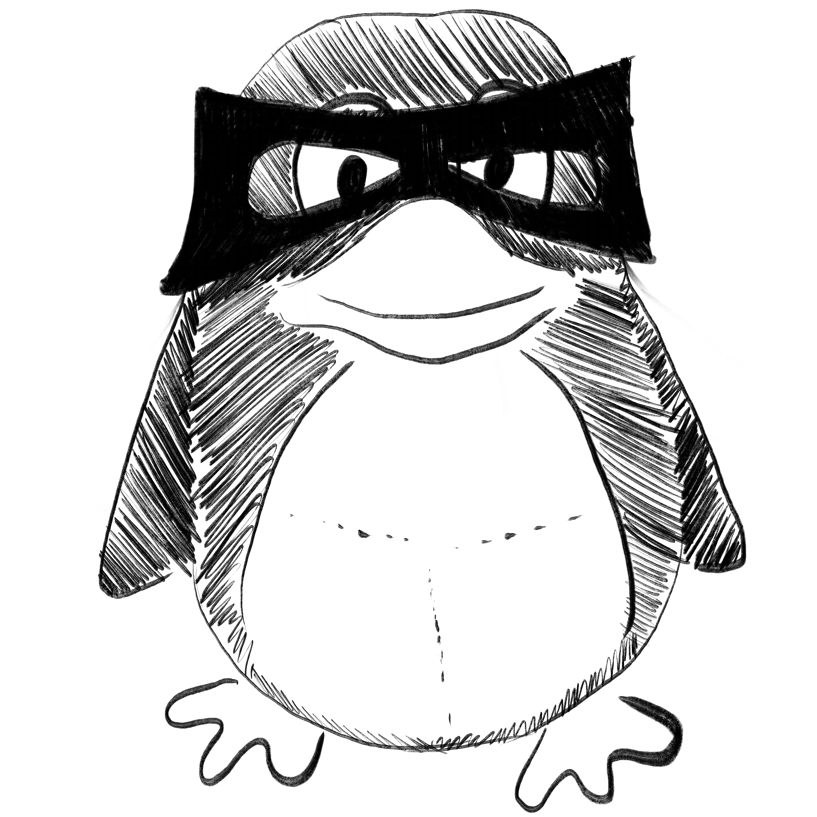
Using Explainable Artificial Intelligence to Predict Potentially Preventable Hospitalizations: A Population-Based Cohort Study in Denmark.
In Medical care
BACKGROUND :
OBJECTIVES :
METHODS :
RESULTS :
CONCLUSION :
Riis Anders Hammerich, Kristensen Pia Kjær, Lauritsen Simon Meyer, Thiesson Bo, Jørgensen Marianne Johansson
2023-Apr-01
Ethics Principles for Artificial Intelligence-Based Telemedicine for Public Health.
In American journal of public health ; h5-index 90.0
Tiribelli Simona, Monnot Annabelle, Shah Syed F H, Arora Anmol, Toong Ping J, Kong Sokanha
2023-Mar-09
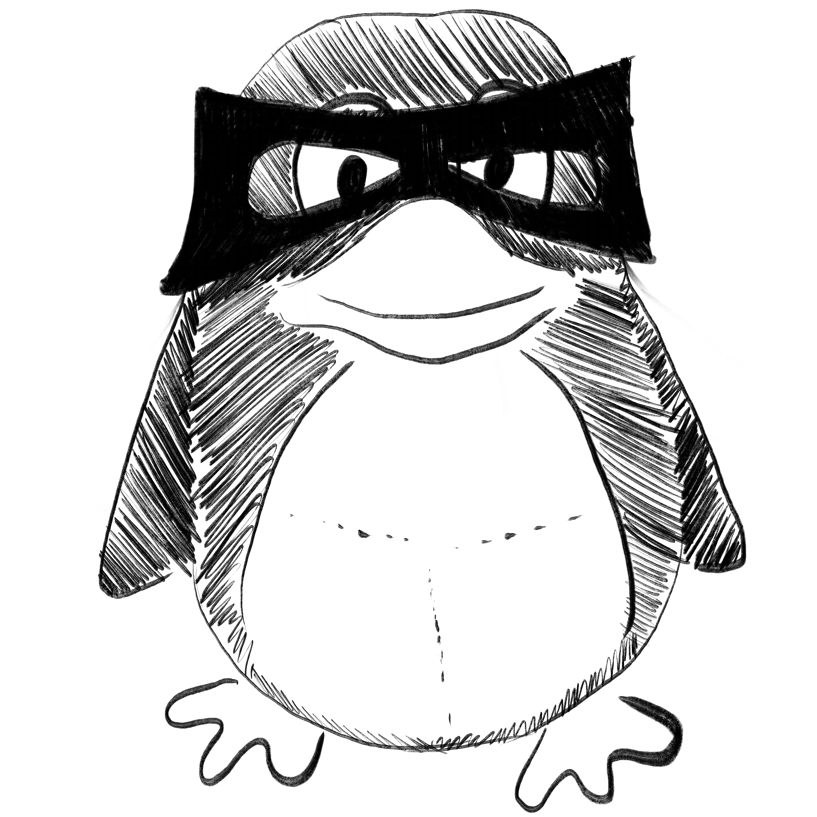
Using Decomposed Error for Reproducing Implicit Understanding of Algorithms.
In Evolutionary computation
Owen Caitlin A, Dick Grant, Whigham Peter A
2023-Mar-09
Genetic programming, biasvariance trade-off, ensemble learning, error decomposition, evolutionary machine learning, geometric semantic genetic programming, stochastic algorithms, symbolic regression
Weekly Summary
Receive a weekly summary and discussion of the top papers of the week by leading researchers in the field.