Receive a weekly summary and discussion of the top papers of the week by leading researchers in the field.
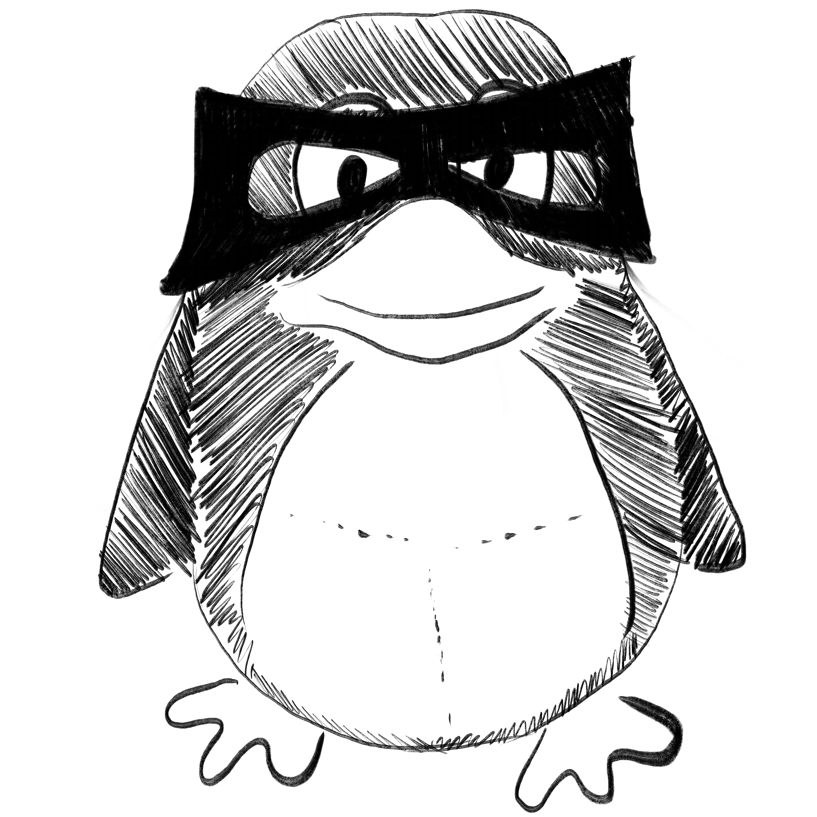
Automated postural asymmetry assessment in infants neurodevelopmental evaluation using novel video-based features.
In Computer methods and programs in biomedicine
BACKGROUND AND OBJECTIVE :
METHODS :
RESULTS :
CONCLUSIONS :
Ledwoń Daniel, Danch-Wierzchowska Marta, Doroniewicz Iwona, Kieszczyńska Katarzyna, Affanasowicz Alicja, Latos Dominika, Matyja Małgorzata, Mitas Andrzej W, Myśliwiec Andrzej
2023-Mar-05
Asymmetry, Computer-aided diagnosis, Features extraction, Infant, Machine learning, Physiotherapy
Differences in Cardiac Mechanics and Exercise Physiology Among Heart Failure With Preserved Ejection Fraction Phenomapping Subgroups.
In The American journal of cardiology ; h5-index 64.0
Dixon Debra D, Beussink-Nelson Lauren, Deo Rahul, Shah Sanjiv J
2023-Mar-06
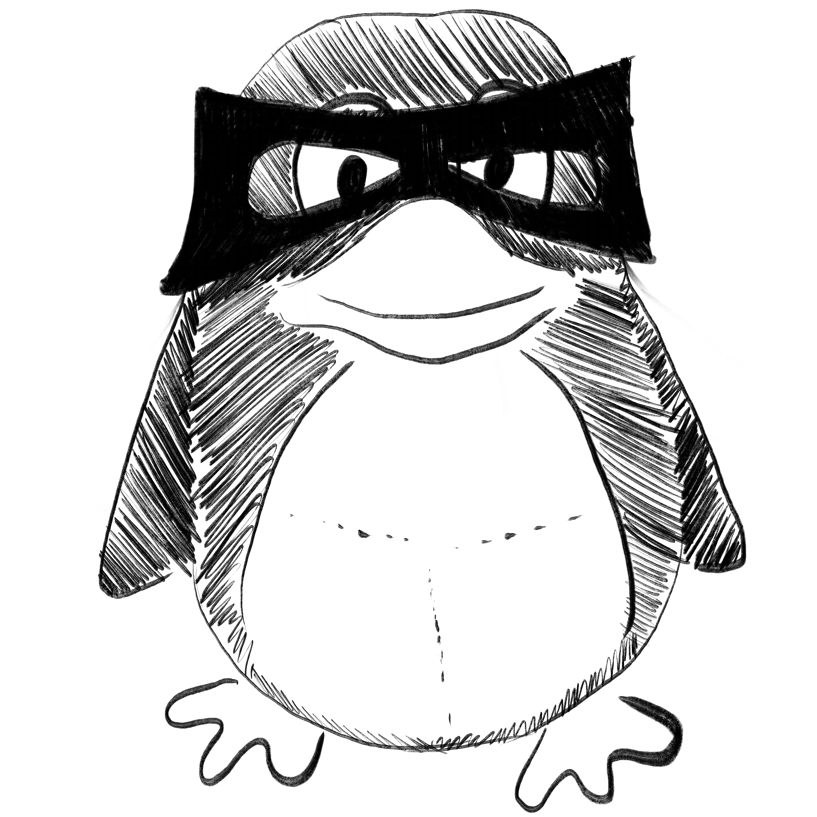
Improved space breakdown method - A robust clustering technique for spike sorting.
In Frontiers in computational neuroscience
UNLABELLED :
CODE AVAILABLE AT :
Ardelean Eugen-Richard, Ichim Ana-Maria, Dînşoreanu Mihaela, Mureşan Raul Cristian
2023
clustering, density, different density, grid, machine learning, overlapping clusters, spike sorting
Determinants of COVID-19 vaccine hesitancy among students and parents in Sentinel Schools Network of Catalonia, Spain.
In PloS one ; h5-index 176.0
Ganem Fabiana, Folch Cinta, Colom-Cadena Andreu, Bordas Anna, Alonso Lucia, Soriano-Arandes Antoni, Casabona Jordi
2023
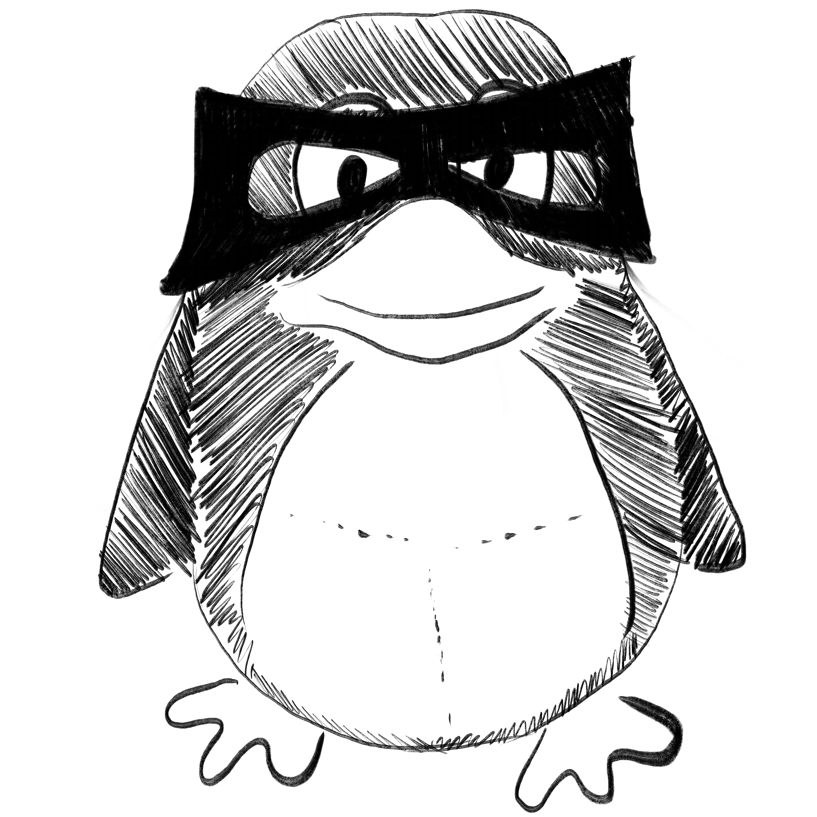
Assessing long-term climate change impact on spatiotemporal changes of groundwater level using autoregressive-based and ensemble machine learning models.
In Journal of environmental management
Nourani Vahid, Ghareh Tapeh Ali Hasanpour, Khodkar Kasra, Huang Jinhui Jeanne
2023-Mar-07
Ardabil plain, GCM, Groundwater, K-means clustering, Machine learning
Does social distancing impact pediatric upper airway infections? An observational controlled study and a brief literature review.
In American journal of otolaryngology ; h5-index 23.0
PURPOSE :
MATERIALS AND METHODS :
RESULTS :
CONCLUSIONS :
Franchella Sebastiano, Favaretto Niccolò, Frigo Annachiara, Franz Leonardo, Pilo Simona, Mularoni Francesca, Marciani Silvia, Nicolai Piero, Marioni Gino, Cazzador Diego
2023-Mar-01
COVID-19, Pediatric, SARS-CoV-2, Social distancing, Upper airways infection
Weekly Summary
Receive a weekly summary and discussion of the top papers of the week by leading researchers in the field.