Receive a weekly summary and discussion of the top papers of the week by leading researchers in the field.
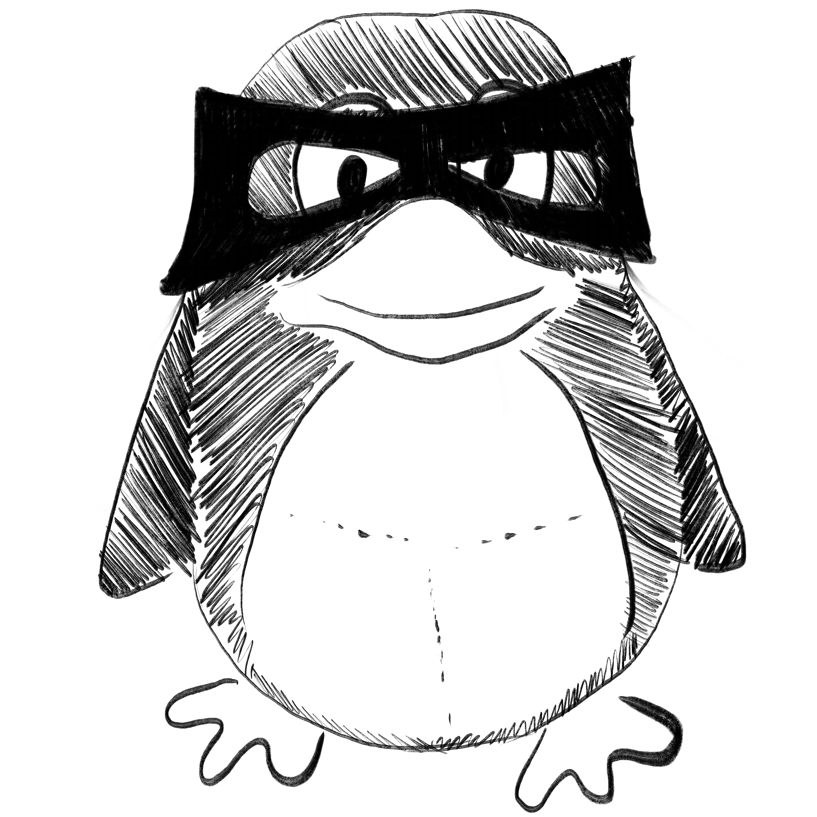
A benchmark for machine-learning based non-invasive blood pressure estimation using photoplethysmogram.
In Scientific data
González Sergio, Hsieh Wan-Ting, Chen Trista Pei-Chun
2023-Mar-21
Predictors of Cyberchondria during the COVID-19 pandemic: A cross-sectional study using supervised machine learning.
In JMIR formative research
BACKGROUND :
OBJECTIVE :
METHODS :
RESULTS :
CONCLUSIONS :
Infanti Alexandre, Starcevic Vladan, Schimmenti Adriano, Khazaal Yasser, Karila Laurent, Giardina Alessandro, Flayelle Maèva, Hedayatzadeh Razavi Seyedeh Boshra, Baggio Stéphanie, Vögele Claus, Billieux Joël
2023-Mar-09
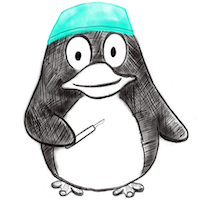
LABRAD-OR: Lightweight Memory Scene Graphs for Accurate Bimodal Reasoning in Dynamic Operating Rooms
ArXiv Preprint
Ege Özsoy, Tobias Czempiel, Felix Holm, Chantal Pellegrini, Nassir Navab
2023-03-23
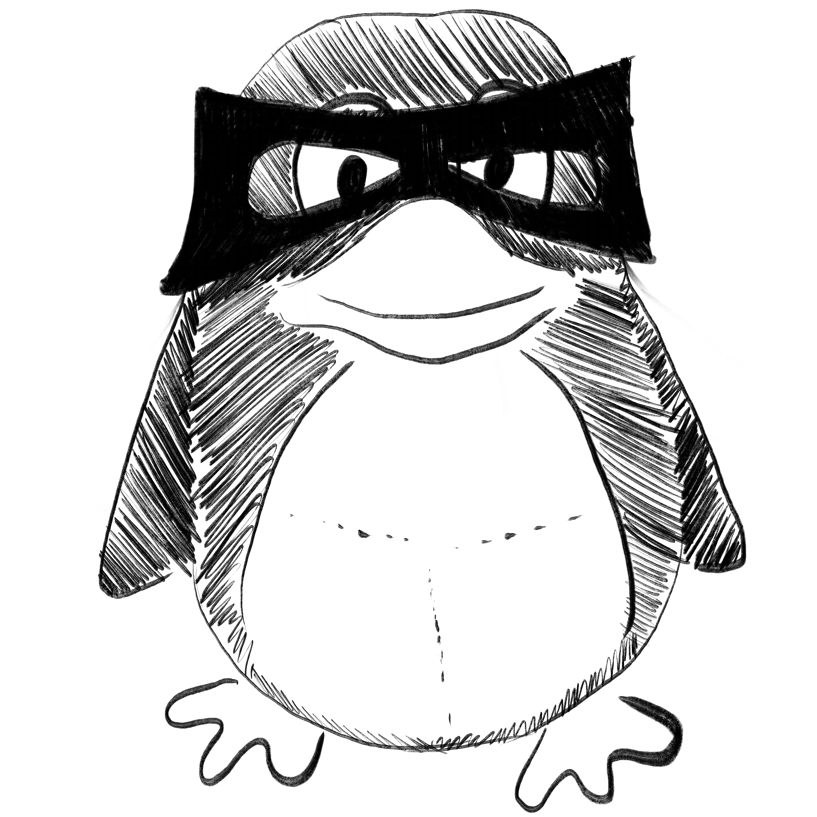
Deep learning-enabled broadband full-Stokes polarimeter with a portable fiber optical spectrometer.
In Optics letters
Xian Shilin, Yang Xiu, Zhou Jie, Gao Fuhua, Hou Yidong
2023-Mar-15
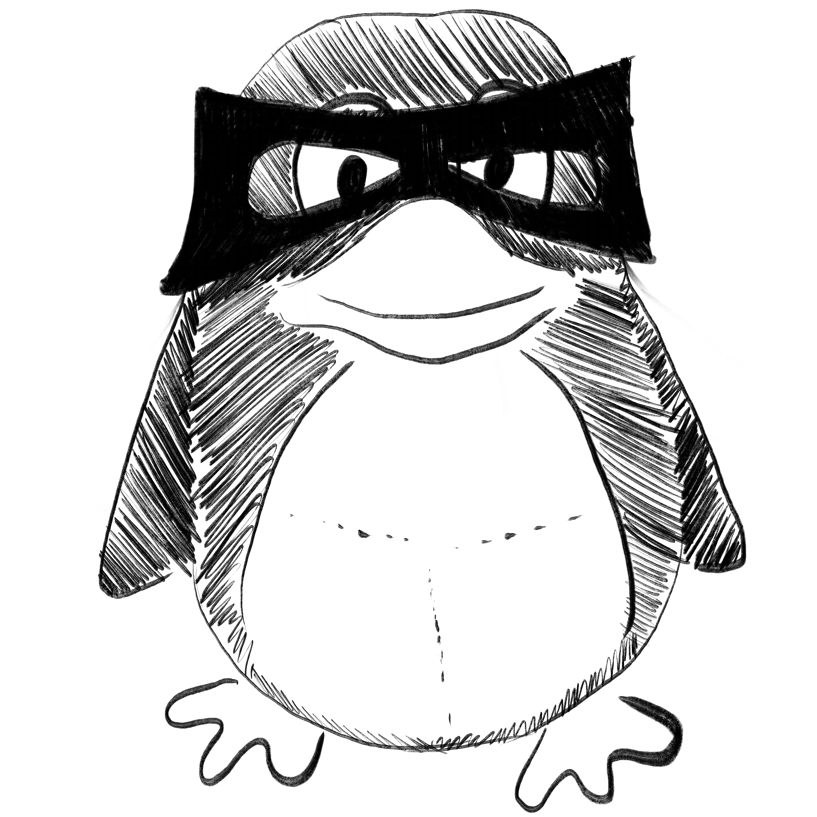
Conditional Forest Models Built Using Metagenomic Data Accurately Predicted Salmonella Contamination in Northeastern Streams.
In Microbiology spectrum
Chung Taejung, Yan Runan, Weller Daniel L, Kovac Jasna
2023-Mar-22
Salmonella, indicators, machine learning, microbiome, surface water, water safety
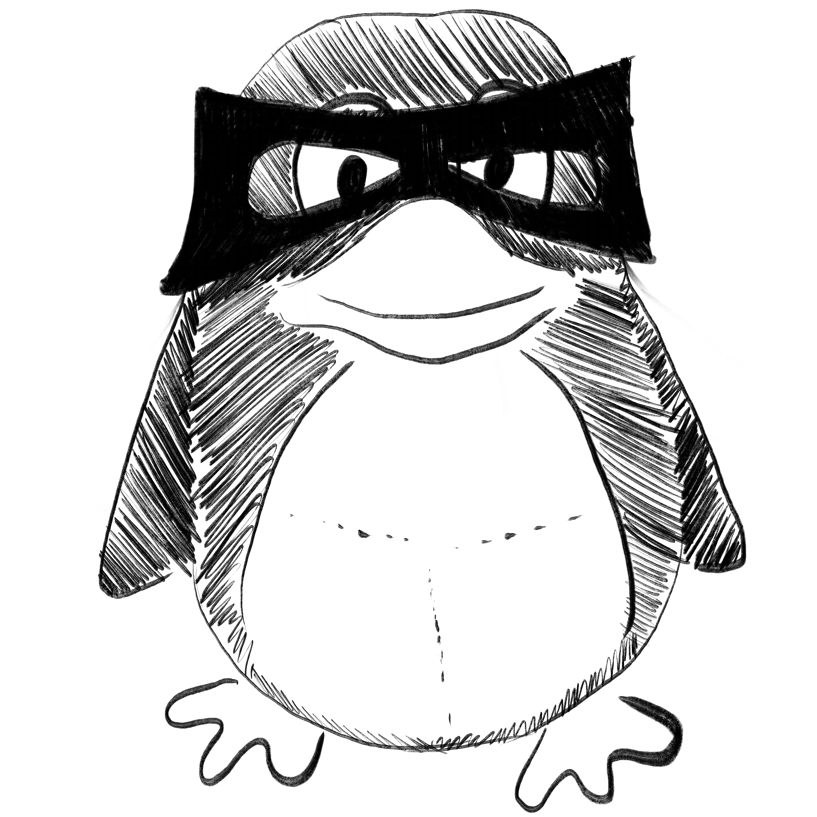
Interpretable machine learning-based approaches for understanding suicide risk and protective factors among South Korean females using survey and social media data.
In Suicide & life-threatening behavior
OBJECTIVE :
MATERIALS AND METHODS :
RESULTS :
CONCLUSIONS :
Kim Donghun, Quan Lihong, Seo Mihye, Kim Kihyun, Kim Jae-Won, Zhu Yongjun
2023-Mar-22
female suicide, model interpretation, protective factors, risk factors, suicide risk prediction
Weekly Summary
Receive a weekly summary and discussion of the top papers of the week by leading researchers in the field.