Receive a weekly summary and discussion of the top papers of the week by leading researchers in the field.
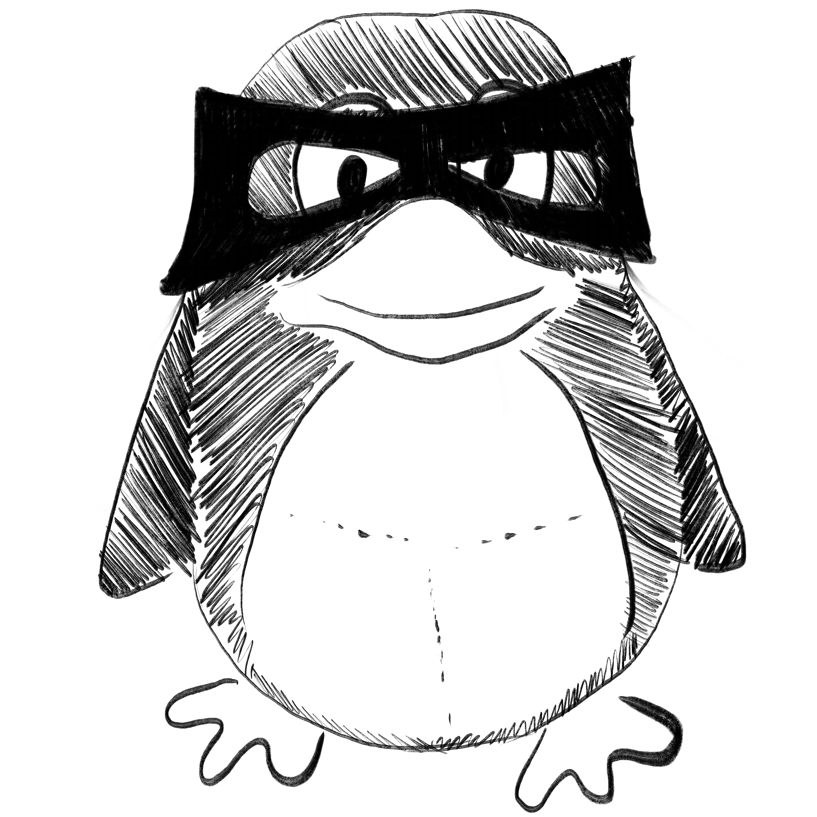
Predictive modeling of pharmaceutical product removal by a managed aquifer recharge system: Comparison and optimization of models using ensemble learners.
In Journal of environmental management
Yaqub Muhammad, Ngoc Nguyen Mai, Park Soohyung, Lee Wontae
2022-Sep-30
Decision tree, Managed aquifer recharge system, Pharmaceutical, Random forest, Xtreme gradient boost
Biomarkers of maternal lead exposure during pregnancy using micro-spatial child deciduous dentine measurements.
In Environment international
BACKGROUND :
AIM :
METHODS :
RESULTS :
DISCUSSION :
Gerbi Lucia, Austin Christine, Pedretti Nicolo Foppa, McRae Nia, Amarasiriwardena Chitra J, Mercado-GarcĂa Adriana, Torres-Olascoaga Libni A, Tellez-Rojo Martha M, Wright Robert O, Arora Manish, Elena Colicino
2022-Sep-16
Blood lead levels, Machine learning, Pregnancy, Prenatal lead exposure, Super-Learner algorithm, Tooth dentine lead levels
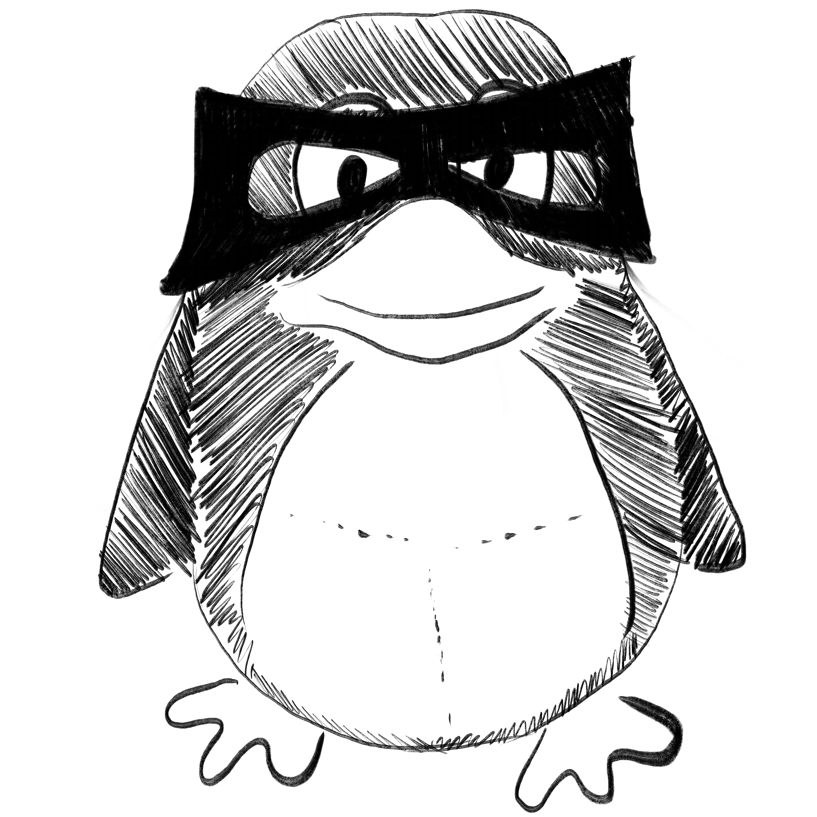
Development and validation of a microenvironment-related prognostic model for hepatocellular carcinoma patients based on histone deacetylase family.
In Translational oncology
BACKGROUND :
METHODS :
RESULTS :
CONCLUSIONS :
Teng Linxin, Li Zhengjun, Shi Yipeng, Gao Zihan, Yang Yang, Wang Yunshan, Bi Lei
2022-Sep-30
Fatty acid metabolism, Hepatocellular carcinoma, Histone deacetylase, Macrophages, Tumor microenvironment
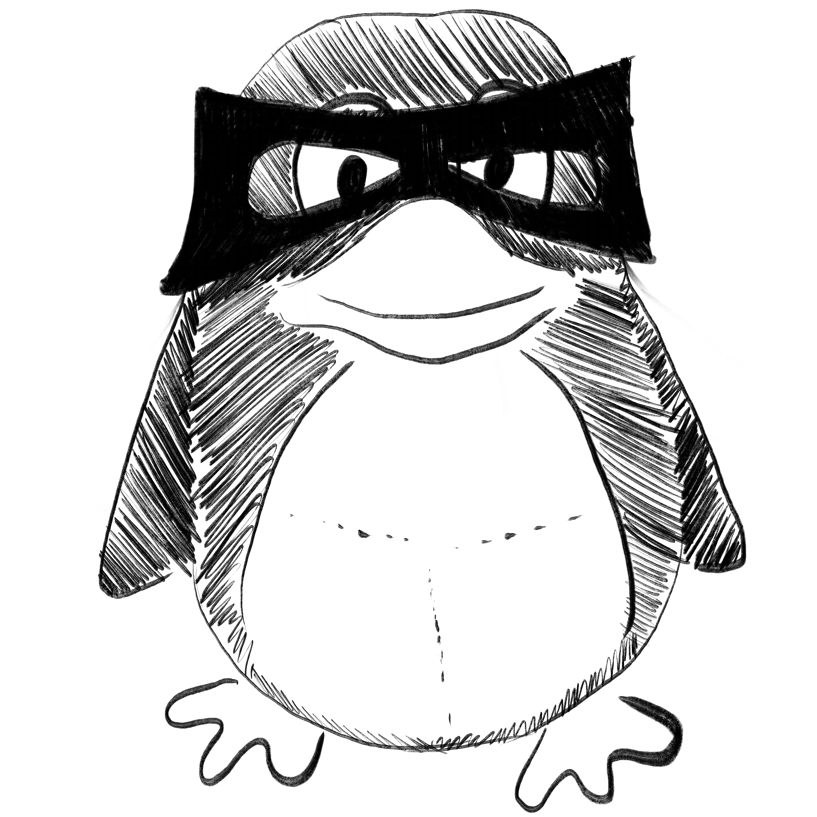
Real-time driving risk assessment using deep learning with XGBoost.
In Accident; analysis and prevention
Shi Liang, Qian Chen, Guo Feng
2022-Sep-30
Convolutional neural network, Crash prediction, Deep learning, Gated recurrent unit, High frequency kinematic driving data, Naturalistic driving study, XGBoost
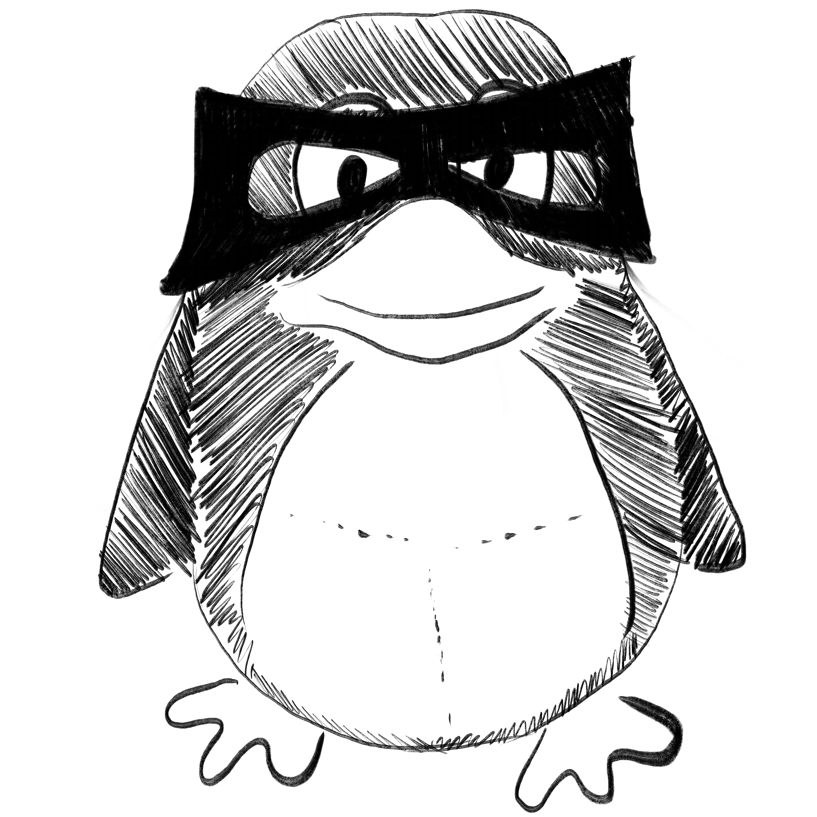
Quantization-aware training for low precision photonic neural networks.
In Neural networks : the official journal of the International Neural Network Society
Kirtas M, Oikonomou A, Passalis N, Mourgias-Alexandris G, Moralis-Pegios M, Pleros N, Tefas A
2022-Sep-19
Constrained-aware training, Neural network quantization, Photonic deep learning
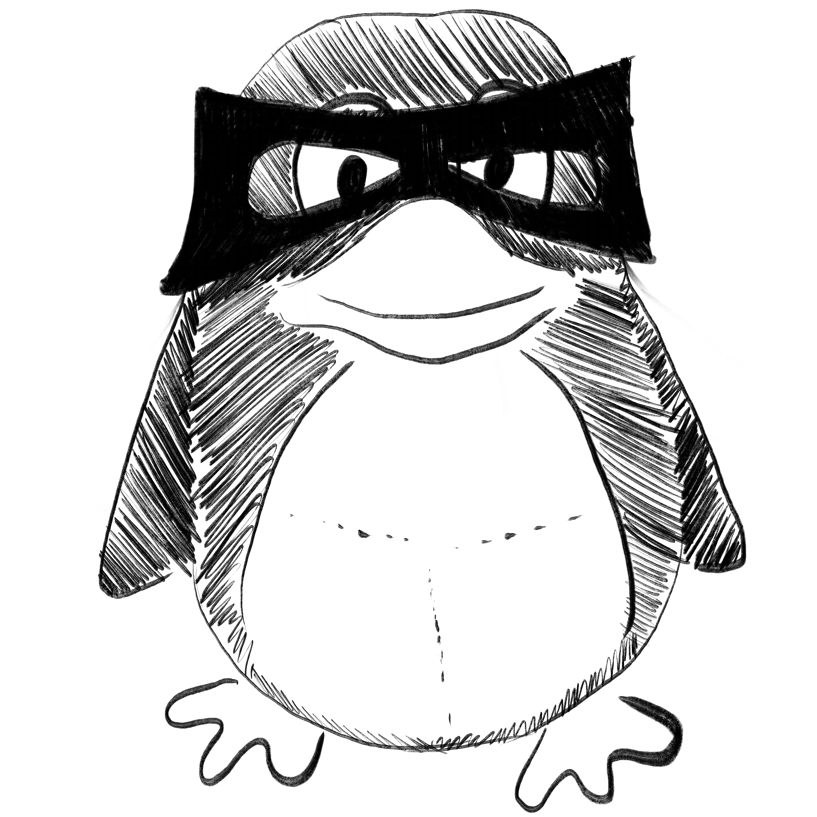
Raman spectroscopy and FTIR spectroscopy fusion technology combined with deep learning: A novel cancer prediction method.
In Spectrochimica acta. Part A, Molecular and biomolecular spectroscopy
Leng Hongyong, Chen Cheng, Chen Chen, Chen Fangfang, Du Zijun, Chen Jiajia, Yang Bo, Zuo Enguang, Xiao Meng, Lv Xiaoyi, Liu Pei
2022-Sep-20
FTIR spectroscopy, Feature fusion, Low-level fusion, MFCNN, Raman
Weekly Summary
Receive a weekly summary and discussion of the top papers of the week by leading researchers in the field.