Receive a weekly summary and discussion of the top papers of the week by leading researchers in the field.
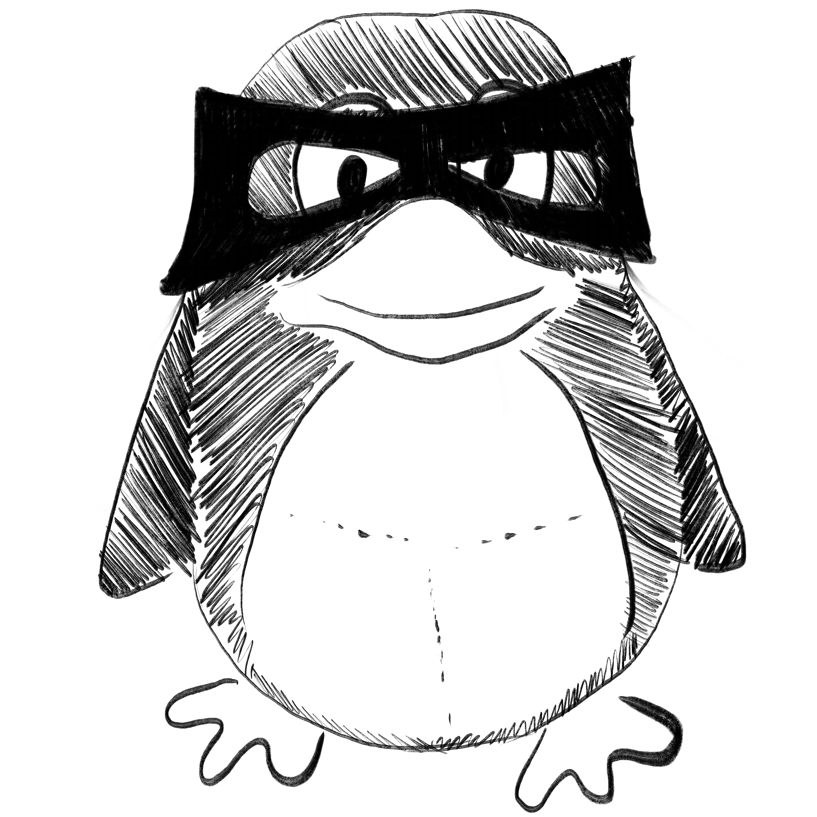
Learning and inferring the diurnal variability of cyanobacterial blooms from high-frequency time-series satellite-based observations.
In Harmful algae
Li Hu, Qin Chengxin, He Weiqi, Sun Fu, Du Pengfei
2023-Mar
CyanoHABs, Diurnal variability, High-frequency remote sensing, Learning and inferring, Spatiotemporal deep learning
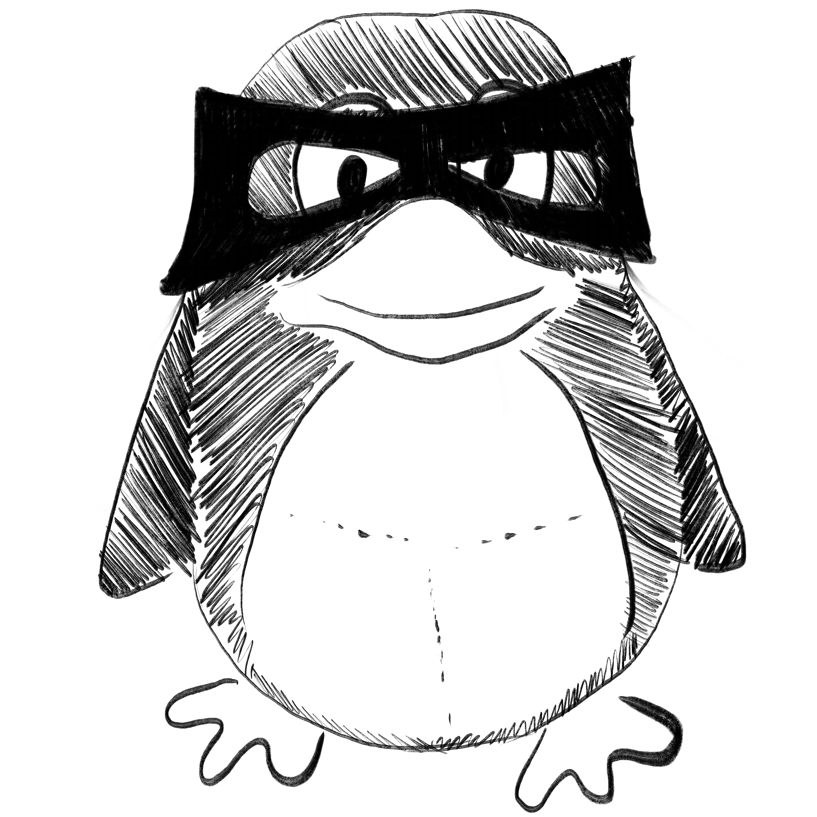
Estimation of reduced greenhouse gas emission from municipal solid waste incineration with electricity recovery in prefecture- and county-level cities of China.
In The Science of the total environment
Zhao Qing, Tang Weihao, Han Mengjie, Cui Wenjing, Zhu Lei, Xie Huaijun, Li Wei, Wu Fengchang
2023-Mar-07
Green energy, Greenhouse gas reduction emission, Incineration, Machine-learning, Municipal solid waste
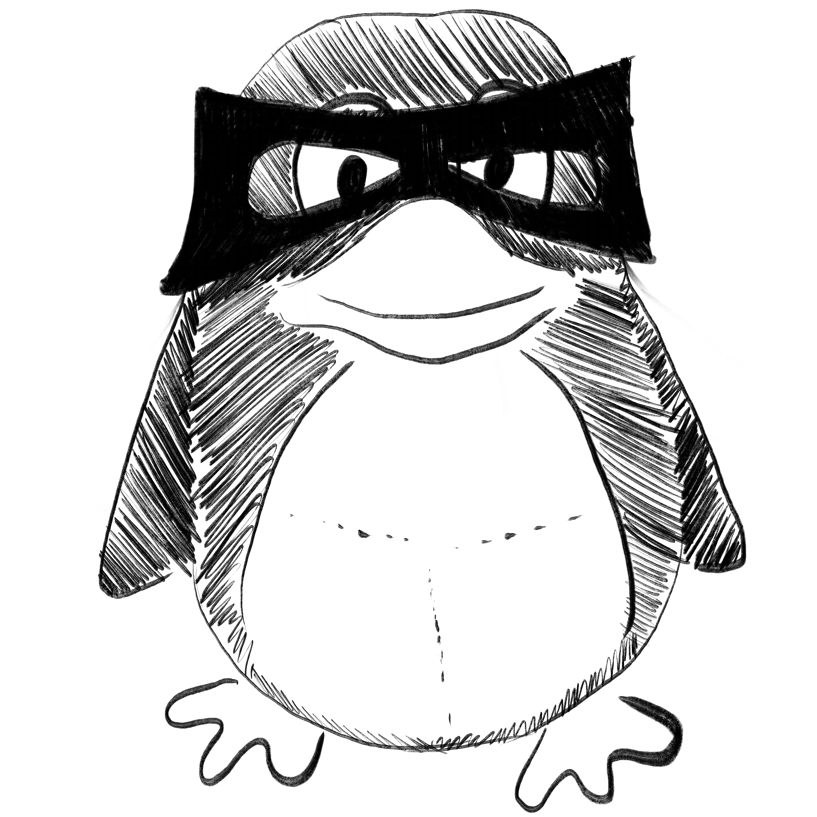
Magnitude and efficiency of straw return in building up soil organic carbon: A global synthesis integrating the impacts of agricultural managements and environmental conditions.
In The Science of the total environment
Li Binzhe, Liang Fei, Wang Yajing, Cao Wenchao, Song He, Chen Jingsheng, Guo Jingheng
2023-Mar-07
Agricultural management, Efficiency, Environmental condition, Magnitude, Soil organic carbon, Straw return
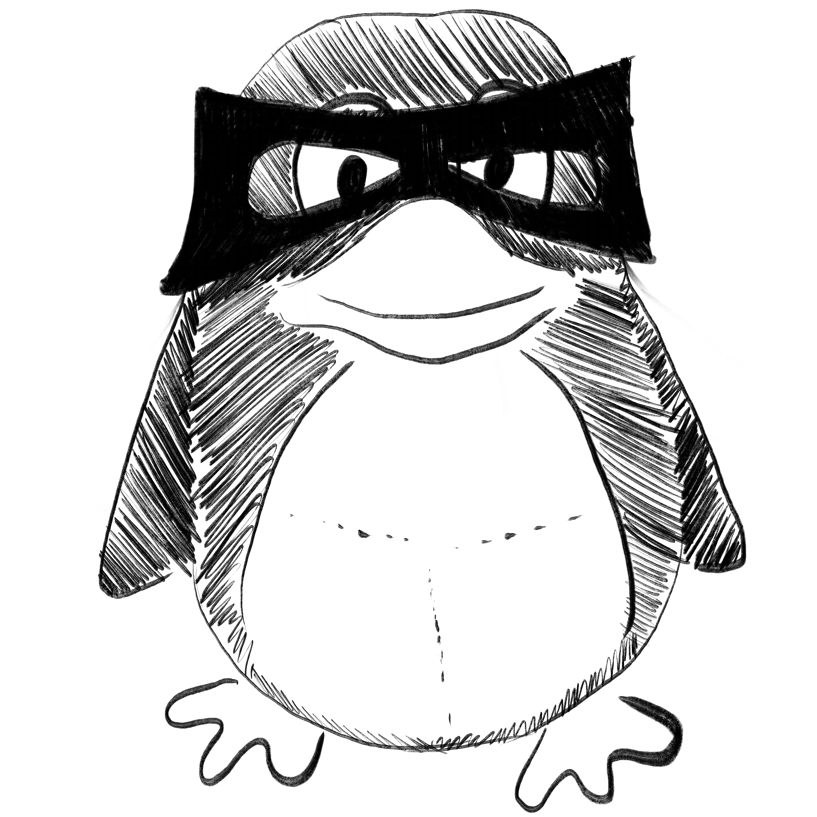
Identification of soil parent materials in naturally high background areas based on machine learning.
In The Science of the total environment
Li Cheng, Zhang Chaosheng, Yu Tao, Ma Xudong, Yang Yeyu, Liu Xu, Hou Qingye, Li Bo, Lin Kun, Yang Zhongfang, Wang Lei
2023-Mar-07
High geological background, Hot spot analysis, Machine learning, Parent material, Soil cadmium
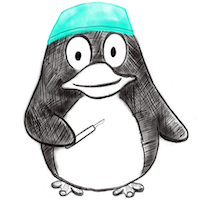
Artificial Intelligence Autonomously Measures Cup Orientation, Corrects for Pelvis Orientation, and Identifies Retroversion from Antero-Posterior Pelvis Radiographs.
In The Journal of arthroplasty ; h5-index 65.0
BACKGROUND :
METHODS :
RESULTS :
CONCLUSION :
Murphy Michael P, Killen Cameron J, Winfrey Sara R, Schmitt Daniel R, Hopkinson William J, Wu Karen, Brown Nicholas M
2023-Mar-07
Abduction, Acetabular component orientation, Anteversion, Inclination, Total hip arthroplasty
Identifying treatment heterogeneity in atrial fibrillation using a novel causal machine learning method.
In American heart journal ; h5-index 58.0
BACKGROUND :
METHODS :
RESULTS :
CONCLUSIONS :
Ngufor Che, Yao Xiaoxi, Inselman Jonathan W, Ross Joseph S, Dhruva Sanket S, Graham David J, Lee Joo-Yeon, Siontis Konstantinos C, Desai Nihar R, Polley Eric, Shah Nilay D, Noseworthy Peter A
2023-Mar-07
Weekly Summary
Receive a weekly summary and discussion of the top papers of the week by leading researchers in the field.