Receive a weekly summary and discussion of the top papers of the week by leading researchers in the field.
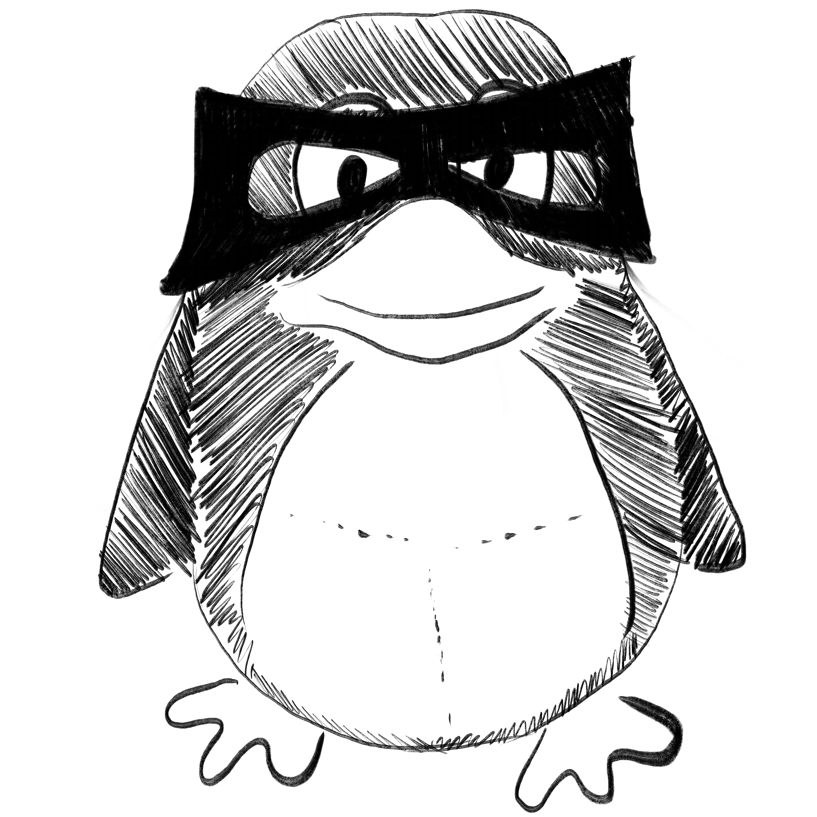
[Artificial intelligence: An introduction for clinicians].
In Revue des maladies respiratoires
Briganti G
2023-Mar-07
Apprentissage machine, Apprentissage profond, Data science, Innovation, Medical informatics, Science des données, Statistics, Statistique
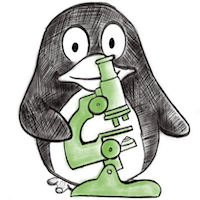
Artificial intelligence in clinical multiparameter flow cytometry and mass cytometry-key tools and progress.
In Seminars in diagnostic pathology
Fuda Franklin, Chen Mingyi, Chen Weina, Cox Andrew
2023-Mar-05
Artificial intelligence, Flow cytometry, Machine learning, Mass cytometry, The Hematology laboratory
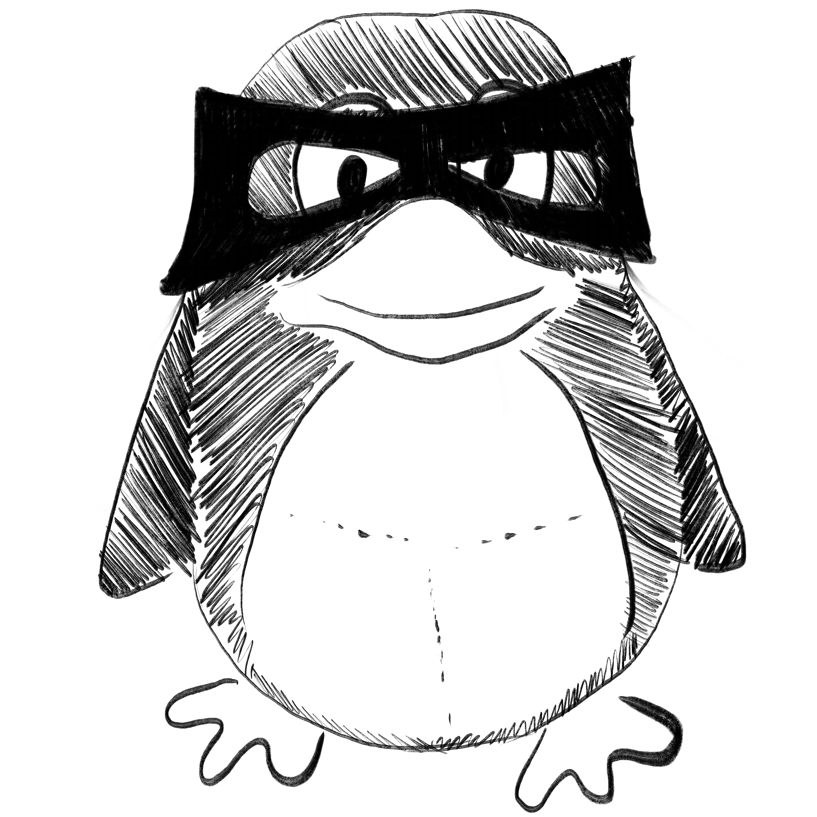
APOC1 as a novel diagnostic biomarker for DN based on machine learning algorithms and experiment.
In Frontiers in endocrinology ; h5-index 55.0
INTRODUCTION :
METHODS :
RESULTS :
CONCLUSIONS :
Yu Kuipeng, Li Shan, Wang Chunjie, Zhang Yimeng, Li Luyao, Fan Xin, Fang Lin, Li Haiyun, Yang Huimin, Sun Jintang, Yang Xiangdong
2023
APOC1, DN, biomarker, diagnostic, machine learning algorithms
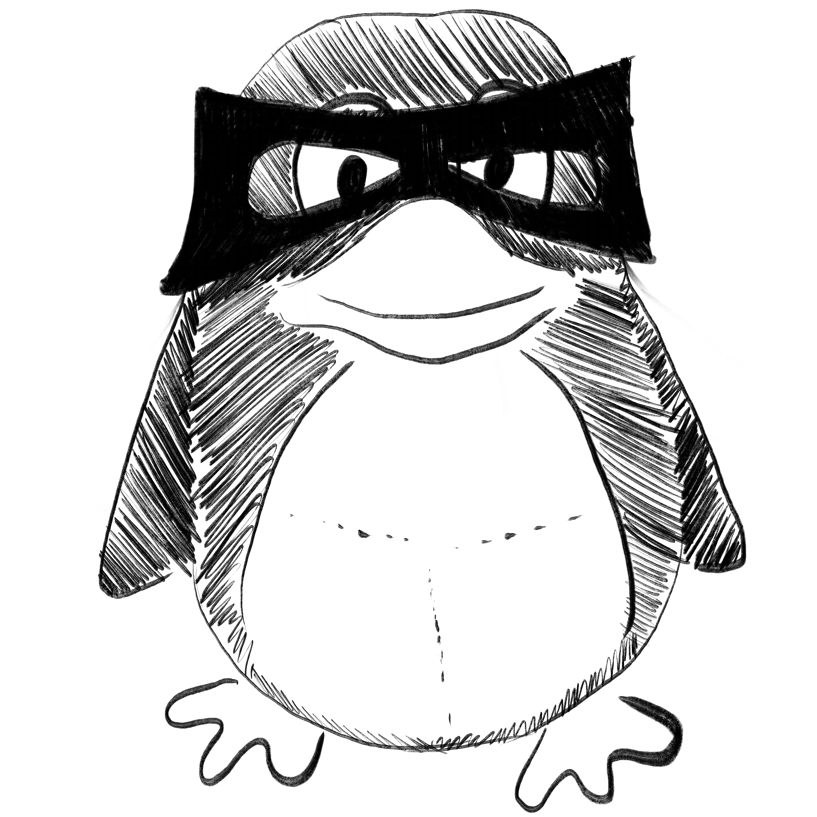
Assessing the effects of data drift on the performance of machine learning models used in clinical sepsis prediction.
In International journal of medical informatics ; h5-index 49.0
BACKGROUND :
METHODS :
RESULTS :
CONCLUSION :
Rahmani Keyvan, Thapa Rahul, Tsou Peiling, Casie Chetty Satish, Barnes Gina, Lam Carson, Foon Tso Chak
2022-Nov-19
Clinical decision support, Data drift, Machine learning, Sepsis
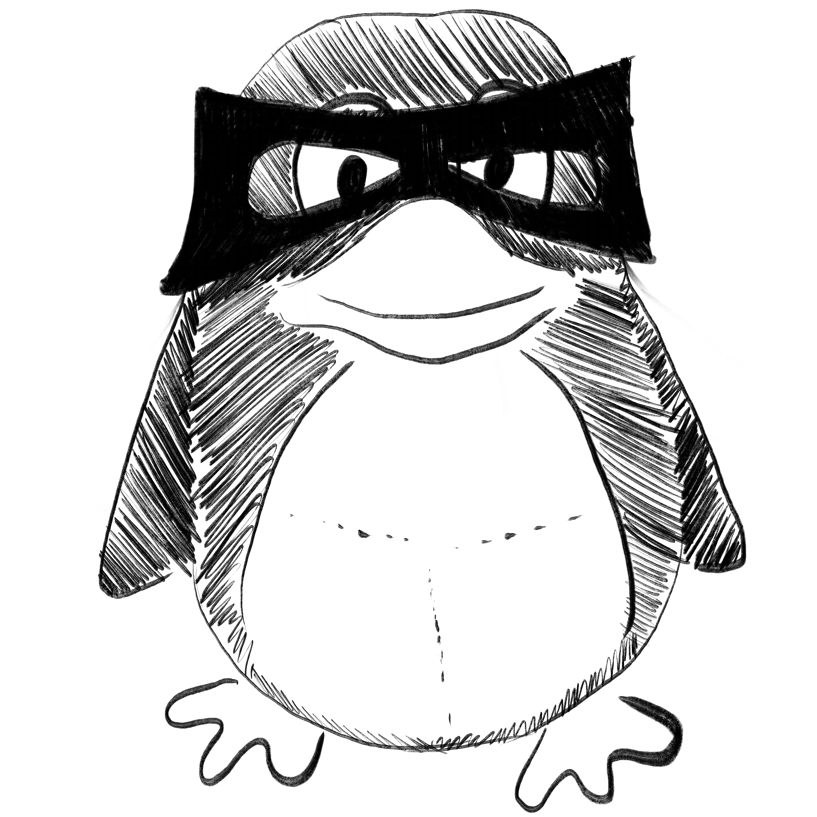
Predicting Corrosion Damage in the Human Body Using Artificial Intelligence: In Vitro Progress and Future Applications.
In The Orthopedic clinics of North America
Kurtz Michael A, Yang Ruoyu, Elapolu Mohan S R, Wessinger Audrey C, Nelson William, Alaniz Kazzandra, Rai Rahul, Gilbert Jeremy L
2023-Apr
Artificial intelligence, Corrosion, Fretting, Machine learning, Neural network, Orthopedic biomaterials, Pitting, Support vector machine, Systematic review
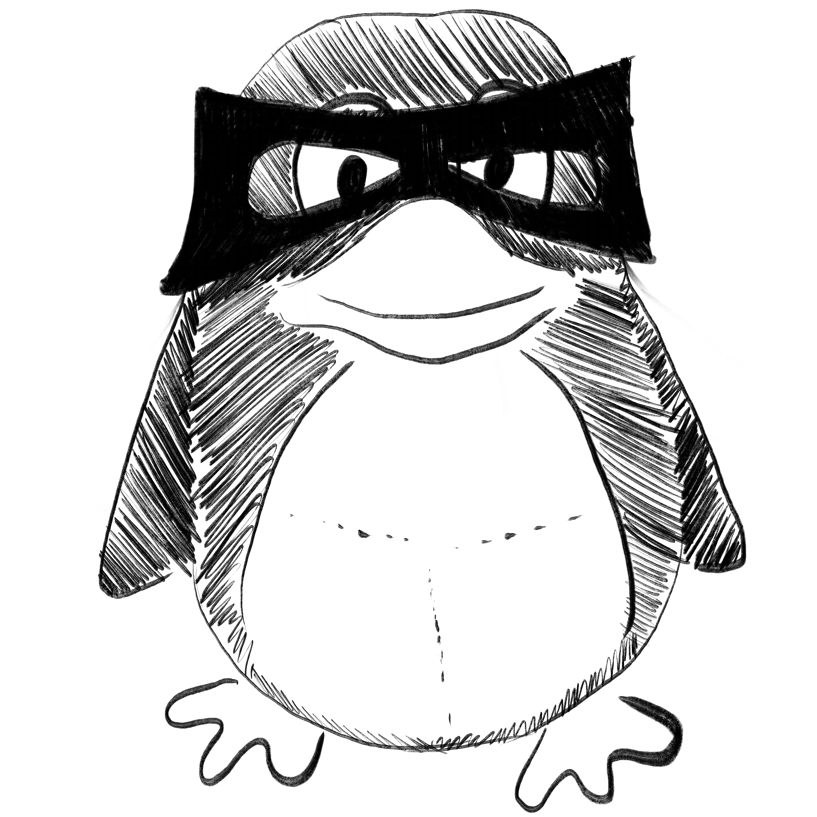
Principles and Validations of an Artificial Intelligence-Based Recommender System Suggesting Acceptable Food Changes.
In The Journal of nutrition ; h5-index 61.0
BACKGROUND :
OBJECTIVES :
METHODS :
RESULTS :
CONCLUSIONS :
Vandeputte Jules, Herold Pierrick, Kuslii Mykyt, Viappiani Paolo, Muller Laurent, Martin Christine, Davidenko Olga, Delaere Fabien, Manfredotti Cristina, Cornuéjols Antoine, Darcel Nicolas
2023-Feb
artificial intelligence, behavior change, decision sciences, food recommendation algorithms, healthy diets
Weekly Summary
Receive a weekly summary and discussion of the top papers of the week by leading researchers in the field.