Receive a weekly summary and discussion of the top papers of the week by leading researchers in the field.
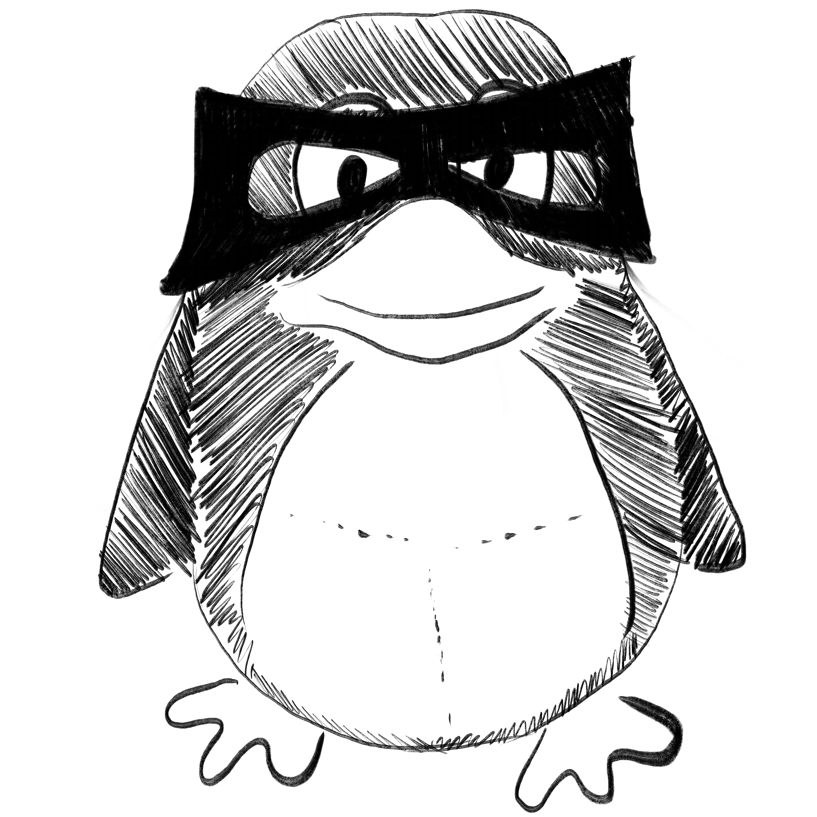
Corn360: a method for quantification of corn kernels.
In Plant methods
BACKGROUND :
RESULTS :
CONCLUSIONS :
Gillette Samantha, Yin Lu, Kianian Penny M A, Pawlowski Wojciech P, Chen Changbin
2023-Mar-09
Corn, High-throughput phenotyping, Image analysis, Kernel color, Kernel texture, Low-cost
Deep phenotyping towards precision psychiatry of first-episode depression - the Brain Drugs-Depression cohort.
In BMC psychiatry
BACKGROUND :
METHODS :
DISCUSSION :
TRIAL REGISTRATION :
Jensen Kristian Høj Reveles, Dam Vibeke H, Ganz Melanie, Fisher Patrick MacDonald, Ip Cheng-Teng, Sankar Anjali, Marstrand-Joergensen Maja Rou, Ozenne Brice, Osler Merete, Penninx Brenda W J H, Pinborg Lars H, Frokjaer Vibe Gedsø, Knudsen Gitte Moos, Jørgensen Martin Balslev
2023-Mar-09
Biomarker, Cognition, EEG, MRI, Major depressive disorder, PET, Precision medicine, Psychotherapy, SSRI, Synaptic density
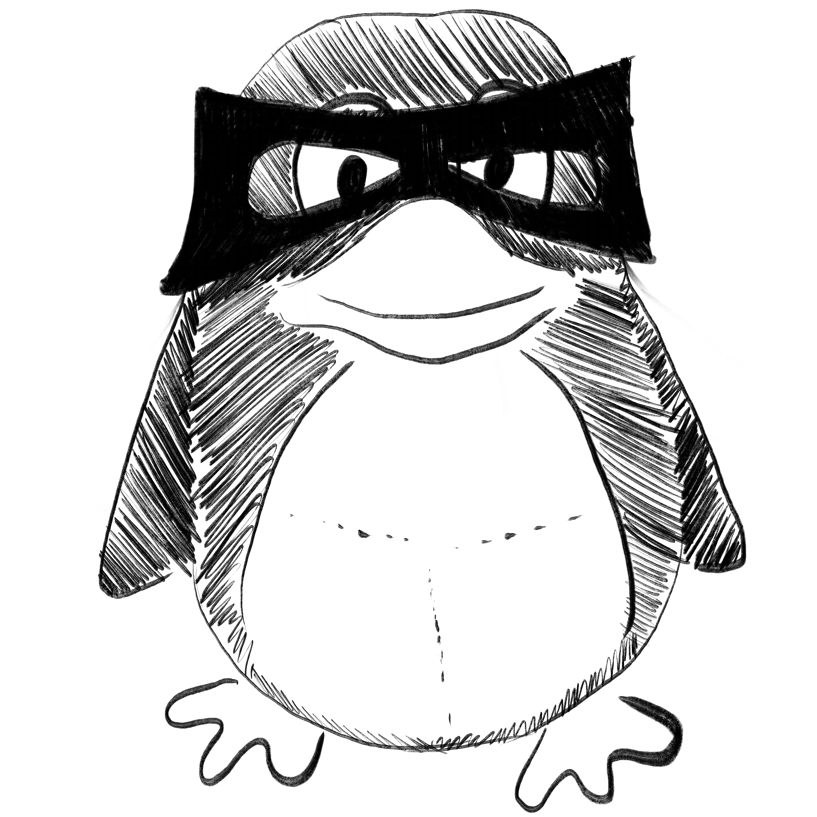
Molecular mechanism and diagnostic marker investigation of endoplasmic reticulum stress on periodontitis.
In BMC oral health ; h5-index 40.0
PURPOSE :
METHODS :
RESULTS :
CONCLUSIONS :
Sun Qianqian, Zhu Enqiang
2023-Mar-09
Diagnostic markers, Drug-gene network, Endoplasmic reticulum stress, Molecular subtype, Periodontitis, miRNA-gene network
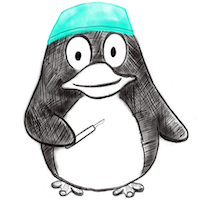
Clinical application of machine learning and computer vision to indocyanine green quantification for dynamic intraoperative tissue characterisation: how to do it.
In Surgical endoscopy ; h5-index 65.0
INTRODUCTION :
METHODS :
RESULTS :
CONCLUSION :
Hardy Niall P, MacAonghusa Pol, Dalli Jeffrey, Gallagher Gareth, Epperlein Jonathan P, Shields Conor, Mulsow Jurgen, Rogers Ailín C, Brannigan Ann E, Conneely John B, Neary Peter M, Cahill Ronan A
2023-Mar-09
Artificial intelligence, Colorectal cancer, Fluorescence-guided surgery, Indocyanine green quantification, Machine learning
"Will I change nodule management recommendations if I change my CAD system?"-impact of volumetric deviation between different CAD systems on lesion management.
In European radiology ; h5-index 62.0
OBJECTIVES :
METHODS :
RESULTS :
CONCLUSIONS :
KEY POINTS :
Peters Alan A, Christe Andreas, von Stackelberg Oyunbileg, Pohl Moritz, Kauczor Hans-Ulrich, Heußel Claus Peter, Wielpütz Mark O, Ebner Lukas
2023-Mar-10
Artificial intelligence, Computer-assisted diagnosis, Deep learning, Imaging phantoms, Lung neoplasms
Deep convolutional neural network-the evaluation of cervical vertebrae maturation.
In Oral radiology
OBJECTIVES :
METHODS :
RESULTS :
CONCLUSION :
Akay Gülsün, Akcayol M Ali, Özdem Kevser, Güngör Kahraman
2023-Mar-09
Artificial intelligence, Cervical vertebrae maturation, Convolutional neural networks, Deep learning
Weekly Summary
Receive a weekly summary and discussion of the top papers of the week by leading researchers in the field.