Receive a weekly summary and discussion of the top papers of the week by leading researchers in the field.
CROPro: a tool for automated cropping of prostate magnetic resonance images.
In Journal of medical imaging (Bellingham, Wash.)
PURPOSE :
APPROACH :
RESULTS :
CONCLUSION :
Patsanis Alexandros, Sunoqrot Mohammed R S, Bathen Tone F, Elschot Mattijs
2023-Mar
deep learning, image cropping, image processing, magnetic resonance imaging, prostate cancer
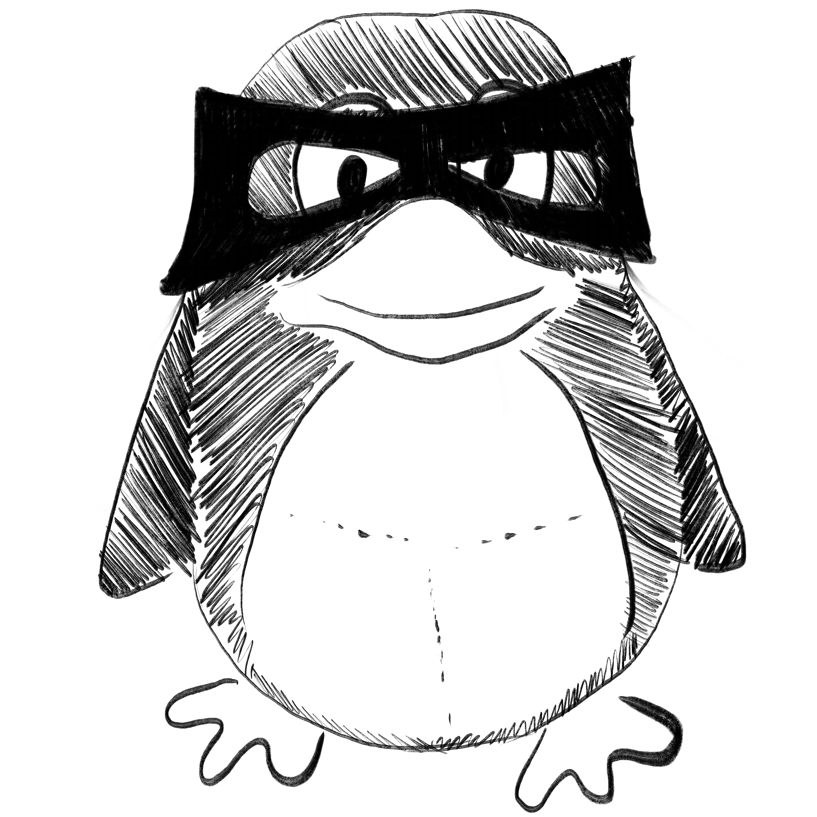
Companion robots to mitigate loneliness among older adults: Perceptions of benefit and possible deception.
In Frontiers in psychology ; h5-index 92.0
OBJECTIVE :
METHODS :
RESULTS :
DISCUSSION :
Berridge Clara, Zhou Yuanjin, Robillard Julie M, Kaye Jeffrey
2023
artificial intelligence, dementia, ethics, natural language processesing, robotics
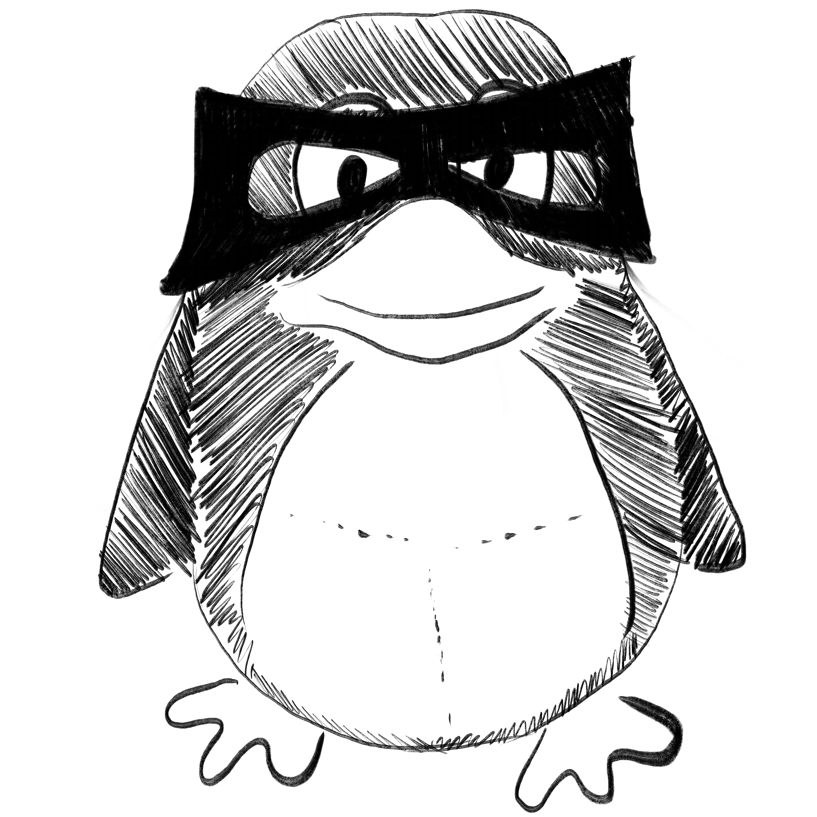
The acute effects of whole-body vibration on motor unit recruitment and discharge properties.
In Frontiers in physiology
Lecce E, Nuccio S, Del Vecchio A, Conti A, Nicolò A, Sacchetti M, Felici F, Bazzucchi I
2023
HDsEMG, MU recruitment, WBV, muscle strength, neuromuscular control
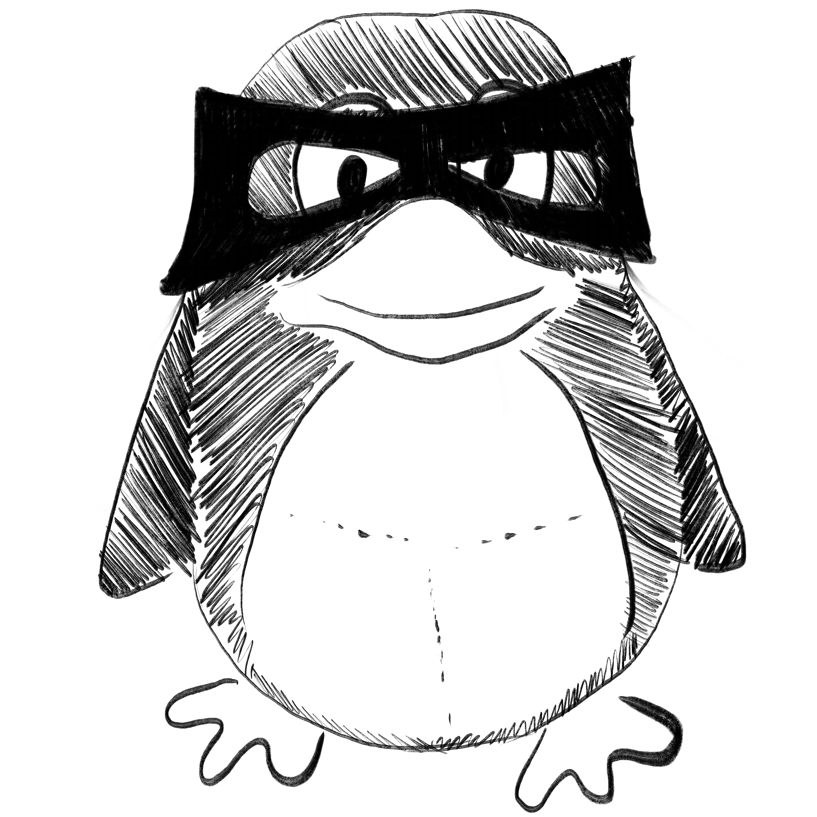
Integration of bulk RNA sequencing and single-cell analysis reveals a global landscape of DNA damage response in the immune environment of Alzheimer's disease.
In Frontiers in immunology ; h5-index 100.0
BACKGROUND :
METHODS :
RESULTS :
CONCLUSIONS :
Lai Yongxing, Lin Han, Chen Manli, Lin Xin, Wu Lijuan, Zhao Yinan, Lin Fan, Lin Chunjin
2023
Alzheimer’s disease, DNA damage response, immunity, machine learning, molecular subtypes, single-cell
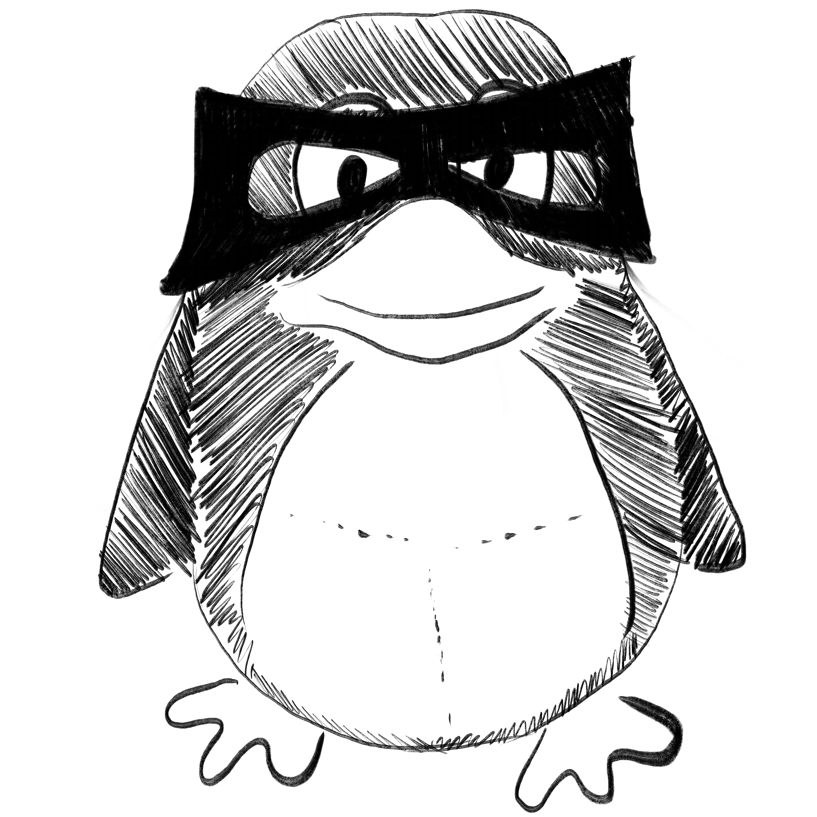
Systemic Inflammatory Response Syndrome on Admission and Clinical Outcomes After Intracerebral Hemorrhage.
In Journal of inflammation research
BACKGROUND :
PATIENTS AND METHODS :
RESULTS :
CONCLUSION :
Liu Lijun, Wang Anxin, Wang Dandan, Guo Jiahuan, Zhang Xiaoli, Zhao Xingquan, Wang Wenjuan
2023
death, disability, in-hospital infections, intracerebral hemorrhage, systemic inflammatory response syndrome
Early detection of myocardial ischemia in resting ECG: analysis by HHT.
In Biomedical engineering online
BACKGROUND :
METHODS :
RESULTS :
CONCLUSIONS :
Wang Chun-Lin, Wei Chiu-Chi, Tsai Cheng-Ting, Lee Ying-Hsiang, Liu Lawrence Yu-Min, Chen Kang-Ying, Lin Yu-Jen, Lin Po-Lin
2023-Mar-10
Exercise electrocardiography, Hilbert–Huang transform, Myocardial energy, Power spectral density
Weekly Summary
Receive a weekly summary and discussion of the top papers of the week by leading researchers in the field.