Receive a weekly summary and discussion of the top papers of the week by leading researchers in the field.
Elicitation of domain knowledge for a machine learning model for paediatric critical illness in South Africa.
In Frontiers in pediatrics
OBJECTIVES :
DESIGN :
SETTING :
PARTICIPANTS :
INTERVENTIONS :
MEASUREMENTS AND MAIN RESULTS :
CONCLUSION :
Pienaar Michael A, Sempa Joseph B, Luwes Nicolaas, George Elizabeth C, Brown Stephen C
2023
children, critical care, domain knowledge, machine learning, severity of illness, triage
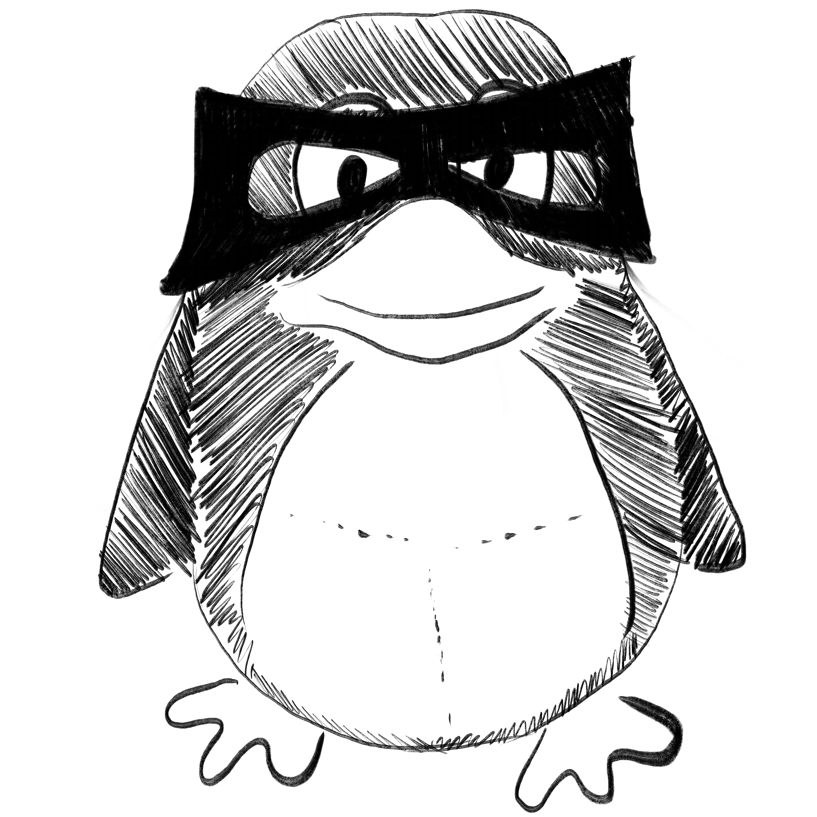
Probing the relevance of the accelerated aging mouse line SAMP8 as a model for certain types of neuropsychiatric symptoms in dementia.
In Frontiers in psychiatry
INTRODUCTION :
METHODS :
RESULTS :
DISCUSSION :
Bergamini Giorgio, Massinet Helene, Hart Aaron, Durkin Sean, Pierlot Gabin, Steiner Michel Alexander
2023
SAMP8 mice, aggression, aging, agitation, deep learning, dementia, sociability
Radiomics model using preoperative computed tomography angiography images to differentiate new from old emboli of acute lower limb arterial embolism.
In Open medicine (Warsaw, Poland)
Liu Rong, Yang Junlin, Zhang Wei, Li Xiaobo, Shi Dai, Cai Wu, Zhang Yue, Fan Guohua, Li Chenglong, Jiang Zhen
2023
X-ray computed tomography, arterial embolization, radiomics, thrombosis
CoviExpert: COVID-19 detection from chest X-ray using CNN.
In Measurement. Sensors
Arivoli A, Golwala Devdatt, Reddy Rayirth
2022-Oct
CNN, COVID-19, CT scan, CoviExpert, Deep learning, X-ray
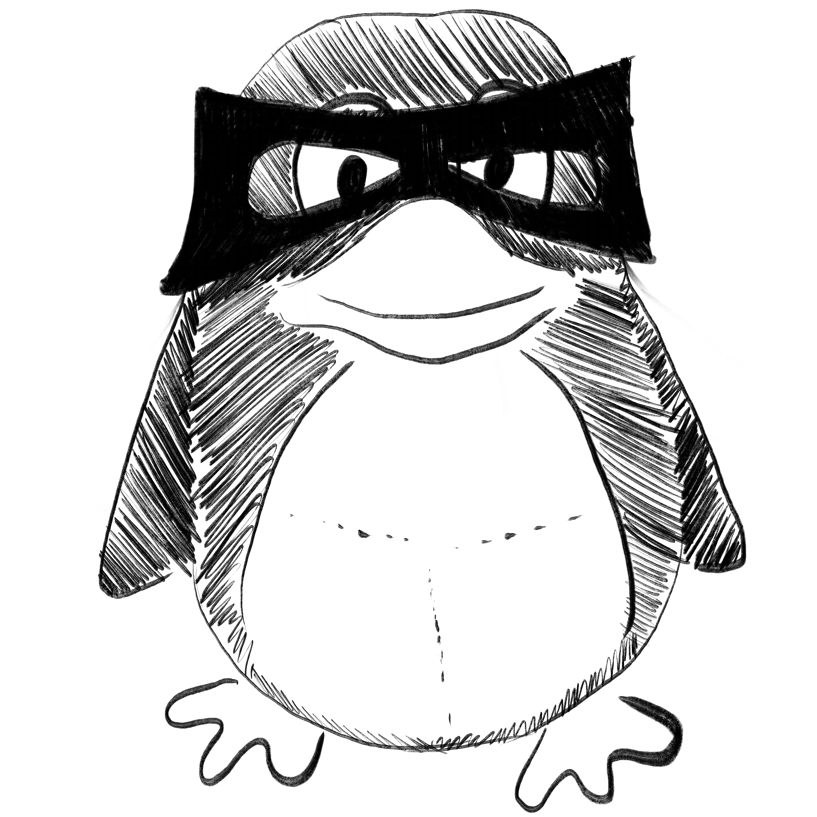
Synthetic computed tomography generation for abdominal adaptive radiotherapy using low-field magnetic resonance imaging.
In Physics and imaging in radiation oncology
BACKGROUND AND PURPOSE :
MATERIALS AND METHODS :
RESULTS :
CONCLUSION :
Garcia Hernandez Armando, Fau Pierre, Wojak Julien, Mailleux Hugues, Benkreira Mohamed, Rapacchi Stanislas, Adel Mouloud
2023-Jan
Deep Learning, Low-field MRI, MR-only treatment planning, Synthetic CT
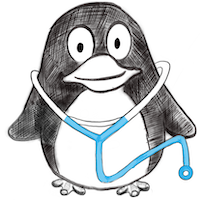
Screening the risk of obstructive sleep apnea by utilizing supervised learning techniques based on anthropometric features and snoring events.
In Digital health
OBJECTIVES :
METHODS :
RESULTS :
CONCLUSIONS :
Tsai Cheng-Yu, Liu Wen-Te, Hsu Wen-Hua, Majumdar Arnab, Stettler Marc, Lee Kang-Yun, Cheng Wun-Hao, Wu Dean, Lee Hsin-Chien, Kuan Yi-Chun, Wu Cheng-Jung, Lin Yi-Chih, Ho Shu-Chuan
2023
Obstructive sleep apnea, Shapley value, anthropometric measure, machine learning, snoring event
Weekly Summary
Receive a weekly summary and discussion of the top papers of the week by leading researchers in the field.