Receive a weekly summary and discussion of the top papers of the week by leading researchers in the field.
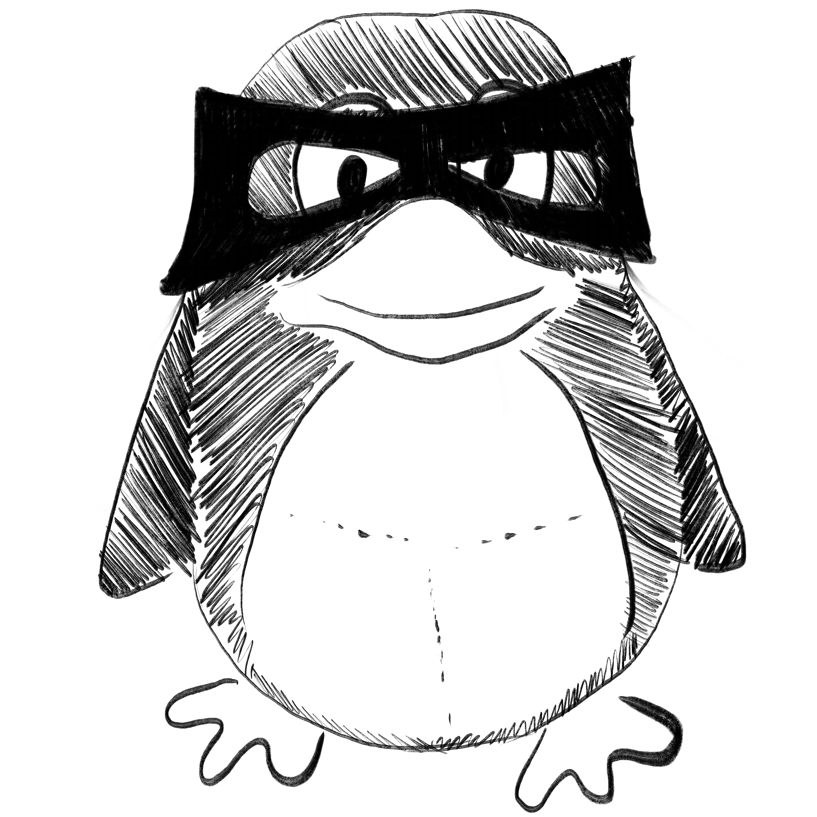
Immersive virtual reality application for intelligent manufacturing: Applications and art design.
In Mathematical biosciences and engineering : MBE
Lei Yu, Su Zhi, He Xiaotong, Cheng Chao
2023-Jan
** immersive Human-machine interaction , intelligent manufacturing , virtual reality **
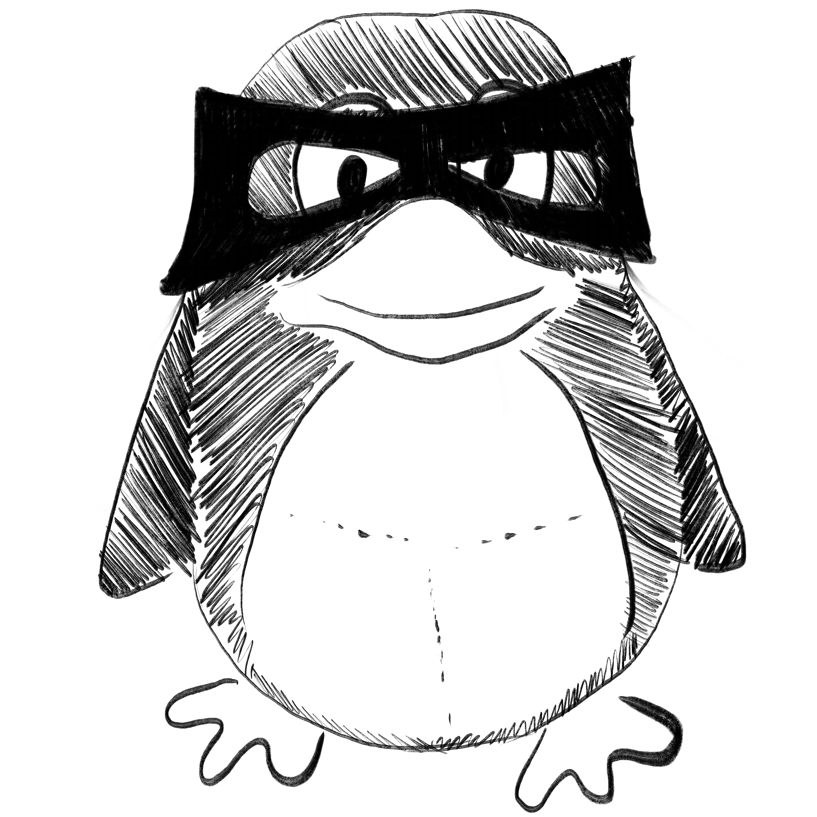
An application of artificial intelligence for investigating the effect of COVID-19 lockdown on three-dimensional temperature variation in equatorial Africa.
In Geoscience frontiers
Okoh Daniel, Onuorah Loretta, Rabiu Babatunde, Obafaye Aderonke, Audu Dauda, Yusuf Najib, Owolabi Oluwafisayo
2022-Mar
COVID-19 lockdown, Equatorial Africa, Neural network, Sunspot number, Temperature, Time-series
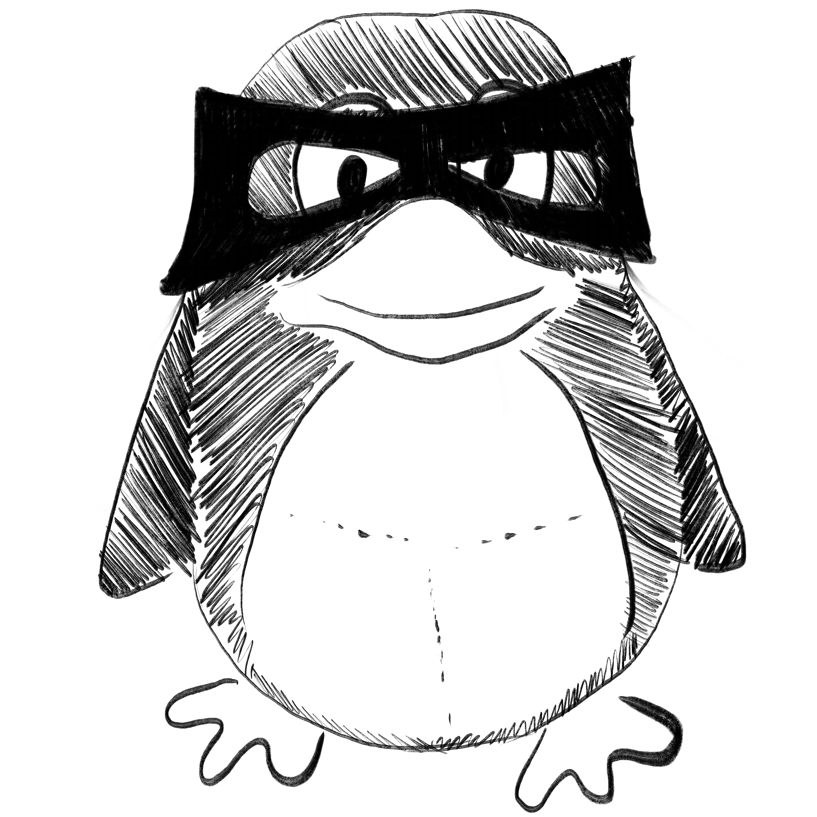
Strengths and limitations of relative wealth indices derived from big data in Indonesia.
In Frontiers in big data
Sartirano Daniele, Kalimeri Kyriaki, Cattuto Ciro, Delamónica Enrique, Garcia-Herranz Manuel, Mockler Anthony, Paolotti Daniela, Schifanella Rossano
2023
index, machine learning, poverty, survey, wealth
Automatic Segmentation of Periapical Radiograph Using Color Histogram and Machine Learning for Osteoporosis Detection.
In International journal of dentistry
Widyaningrum Rini, Sela Enny Itje, Pulungan Reza, Septiarini Anindita
2023
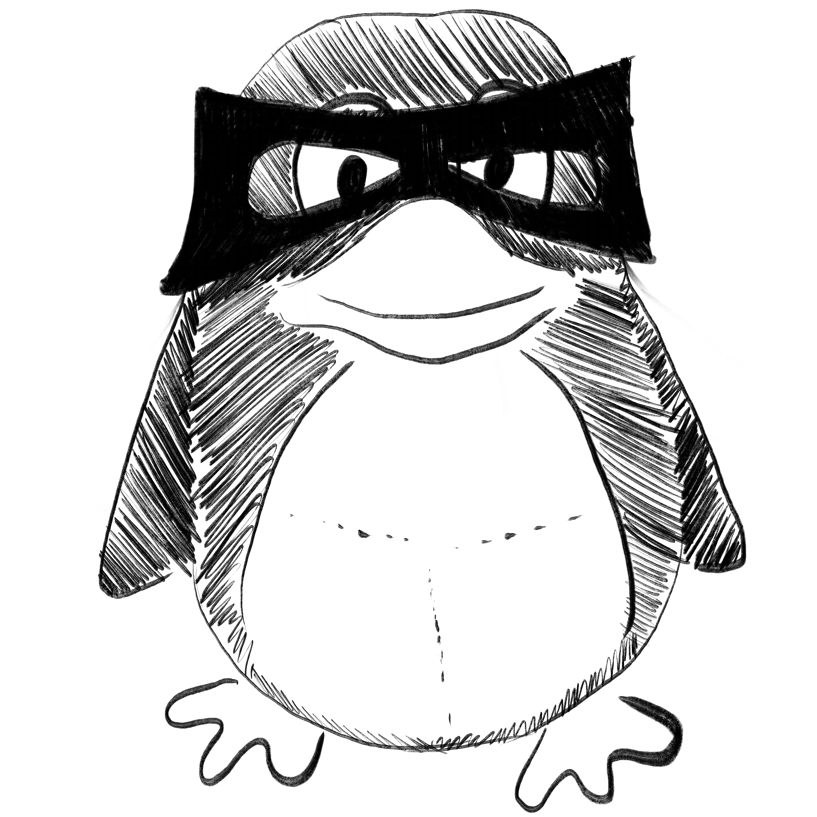
Effects of ultrasound with an automatic vessel detection system using artificial intelligence on the selection of puncture points among ultrasound beginner clinical nurses.
In The journal of vascular access
BACKGROUND :
METHODS :
RESULTS :
CONCLUSION :
Abe-Doi Mari, Murayama Ryoko, Takahashi Toshiaki, Matsumoto Masaru, Tamai Nao, Nakagami Gojiro, Sanada Hiromi
2023-Mar-09
Artificial intelligence, catheter placement, peripheral intravenous catheter, ultrasound, vein assessment
CoviExpert: COVID-19 detection from chest X-ray using CNN.
In Measurement. Sensors
Arivoli A, Golwala Devdatt, Reddy Rayirth
2022-Oct
CNN, COVID-19, CT scan, CoviExpert, Deep learning, X-ray
Weekly Summary
Receive a weekly summary and discussion of the top papers of the week by leading researchers in the field.