Receive a weekly summary and discussion of the top papers of the week by leading researchers in the field.
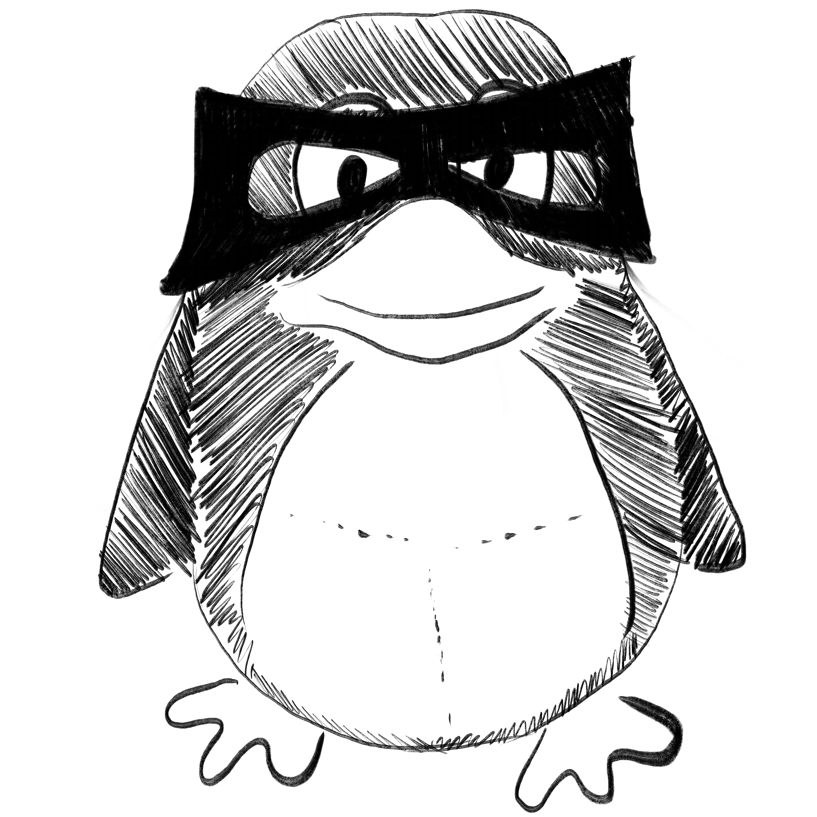
Personalized online ensemble machine learning with applications for dynamic data streams.
In Statistics in medicine
Malenica Ivana, Phillips Rachael V, Chambaz Antoine, Hubbard Alan E, Pirracchio Romain, van der Laan Mark J
2023-Mar-30
machine learning, online learning, personalized medicine, streaming data, time series
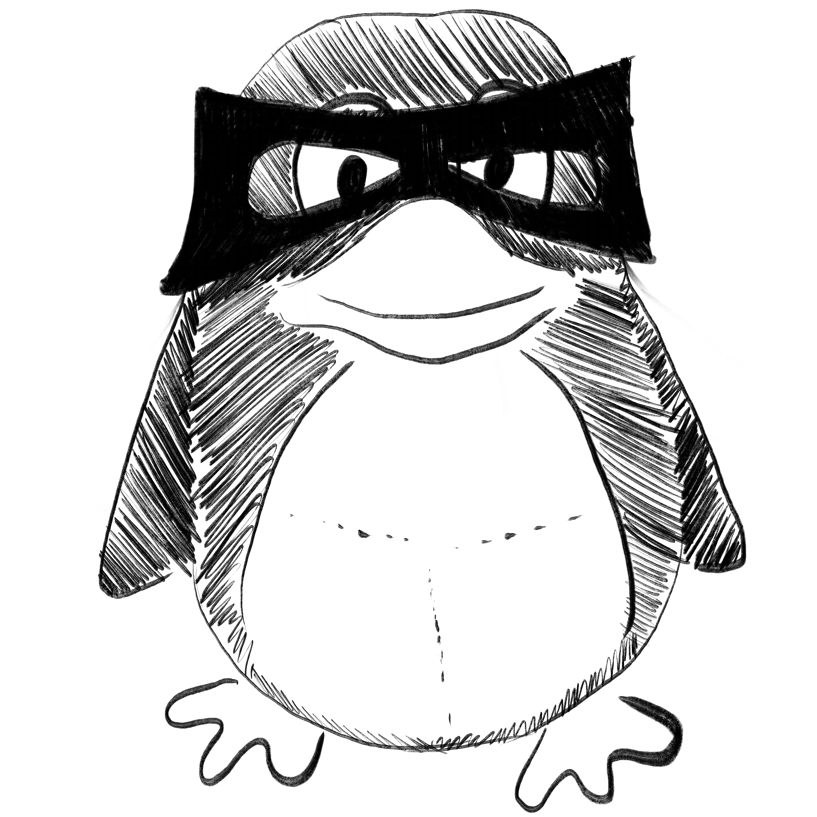
ExamPle: Explainable deep learning framework for the prediction of plant small secreted peptides.
In Bioinformatics (Oxford, England)
MOTIVATION :
RESULTS :
AVAILABILITY :
SUPPLEMENTARY INFORMATION :
Li Zhongshen, Jin Junru, Wang Yu, Long Wentao, Ding Yuanhao, Hu Haiyan, Wei Leyi
2023-Mar-10
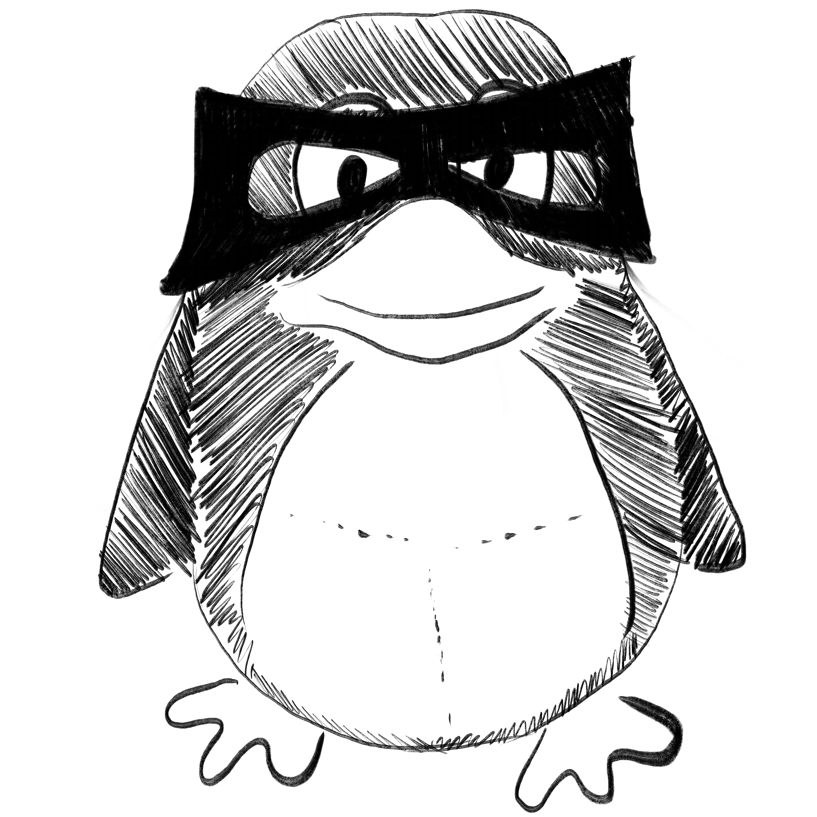
A machine learning-derived neuroanatomical pattern predicts delayed reward discounting in the Human Connectome Project Young Adult sample.
In Journal of neuroscience research
Xu Hui, MacKillop James, Owens Max M
2023-Mar-10
delayed reward discounting, elastic net regression, machine learning, morphometry, neuroanatomy
Deep Learning Radiomics for the Assessment of Telomerase Reverse Transcriptase Promoter Mutation Status in Patients With Glioblastoma Using Multiparametric MRI.
In Journal of magnetic resonance imaging : JMRI
BACKGROUND :
PURPOSE :
STUDY TYPE :
POPULATION :
FIELD STRENGTH/SEQUENCE :
ASSESSMENT :
STATISTICAL TESTS :
RESULTS :
DATA CONCLUSION :
LEVEL OF EVIDENCE :
Zhang Hongbo, Zhang Hanwen, Zhang Yuze, Zhou Beibei, Wu Lei, Lei Yi, Huang Biao
2023-Mar-10
deep learning radiomics, glioblastoma, multiparametric magnetic resonance imaging, telomerase reverse transcriptase
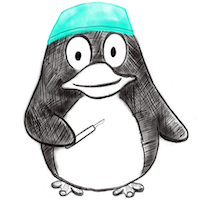
Lymph Node Metastases in Papillary Thyroid Carcinoma can be Predicted by a Convolutional Neural Network: a Multi-Institution Study.
In The Annals of otology, rhinology, and laryngology
OBJECTIVES :
METHODS :
RESULTS :
CONCLUSION :
Esce Antoinette, Redemann Jordan P, Olson Garth T, Hanson Joshua A, Agarwal Shweta, Yenwongfai Leonard, Ferreira Juanita, Boyd Nathan H, Bocklage Thèrése, Martin David R
2023-Mar-10
head and neck and endocrine pathology, head and neck surgery, histopathology, lymphatic metastasis, papillary thyroid cancer
Development of multiple AI pipelines that predict neoadjuvant chemotherapy response of breast cancer using H&E-stained tissues.
In The journal of pathology. Clinical research
Shen Bin, Saito Akira, Ueda Ai, Fujita Koji, Nagamatsu Yui, Hashimoto Mikihiro, Kobayashi Masaharu, Mirza Aashiq H, Graf Hans Peter, Cosatto Eric, Hazama Shoichi, Nagano Hiroaki, Sato Eiichi, Matsubayashi Jun, Nagao Toshitaka, Cheng Esther, Hoda Syed Af, Ishikawa Takashi, Kuroda Masahiko
2023-Mar-10
artificial intelligence, breast cancer, digital pathology, neoadjuvant chemotherapy
Weekly Summary
Receive a weekly summary and discussion of the top papers of the week by leading researchers in the field.