Receive a weekly summary and discussion of the top papers of the week by leading researchers in the field.
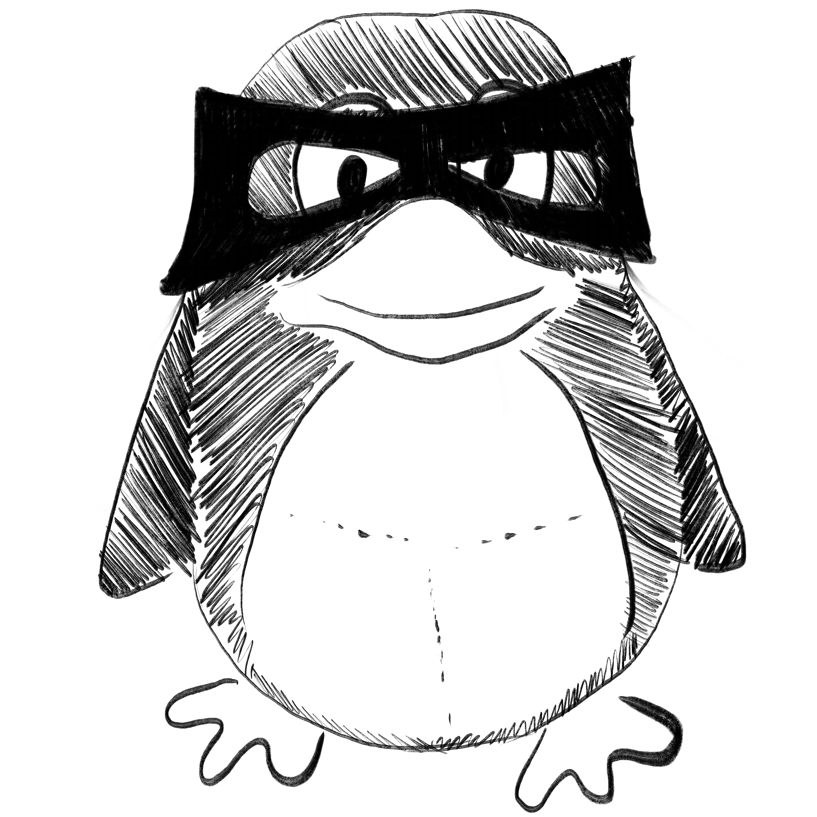
Algal cell viability assessment: The role of environmental factors in phytoplankton population dynamics.
In Marine pollution bulletin
Wang Yanyan, Zhai Wei-Dong, Wu Chi
2023-Mar-08
Cell viability, Deep learning, Digital holography, East China Sea, Harmful algal blooms
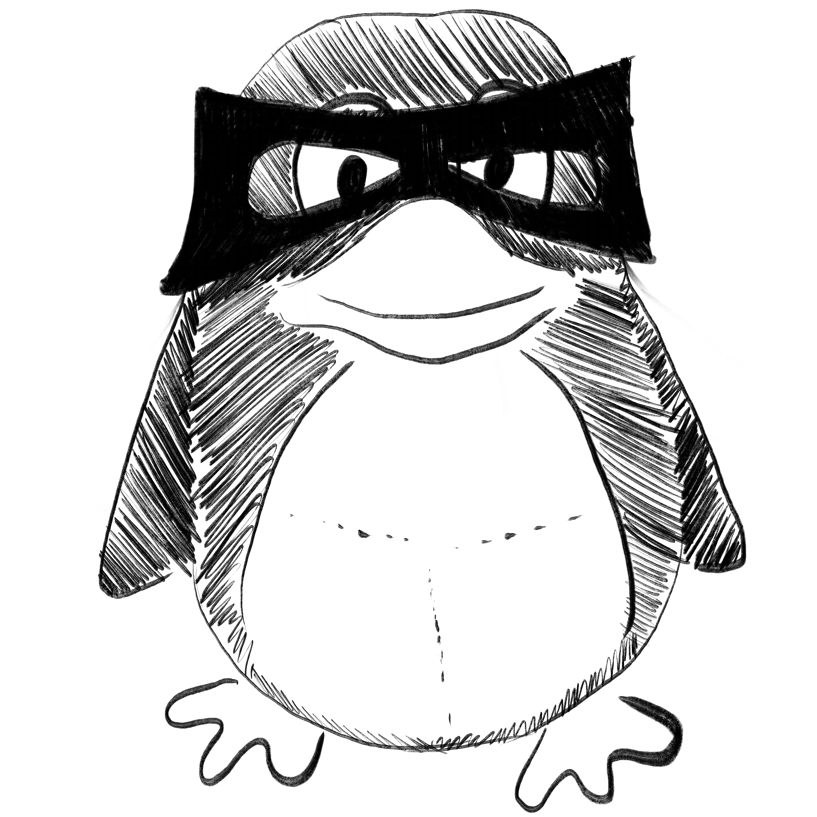
Human-guided deep learning with ante-hoc explainability by convolutional network from non-image data for pregnancy prognostication.
In Neural networks : the official journal of the International Neural Network Society
BACKGROUND AND OBJECTIVE :
METHODS :
RESULTS :
CONCLUSIONS :
Sufriyana Herdiantri, Wu Yu-Wei, Su Emily Chia-Yu
2023-Feb-24
Causal diagram, Deep learning, Electronic health records, Explainable artificial intelligence, Prelabor rupture of membranes
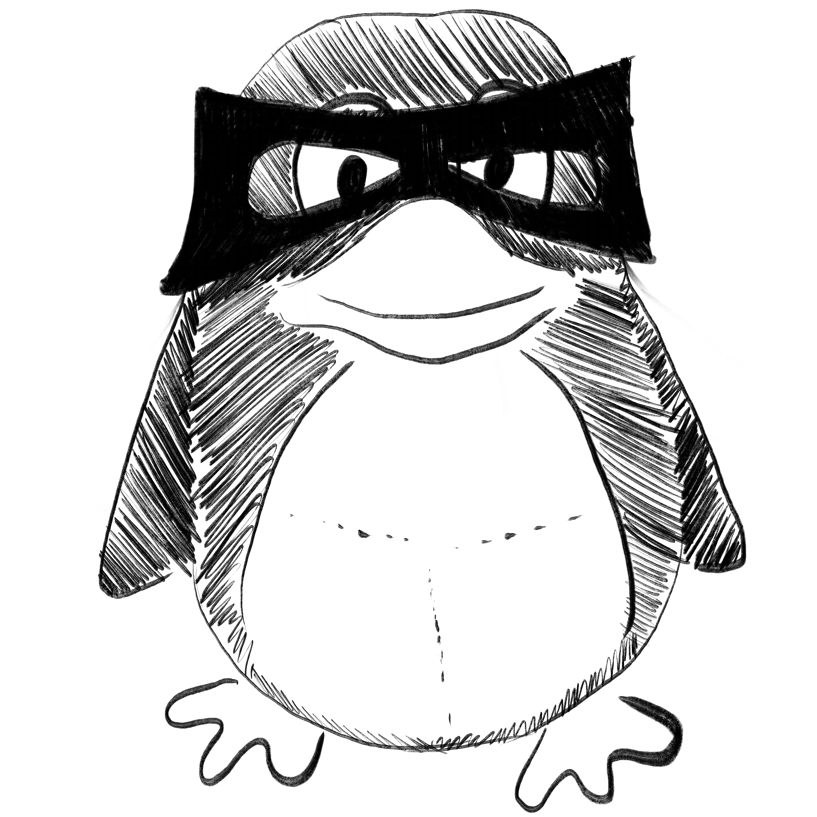
Explore traffic conflict risks considering motion constraint degree in the diverging area of toll plazas.
In Accident; analysis and prevention
Xing Lu, Yu Le, Zheng Ou, Abdel-Aty Mohamed
2023-Mar-08
Driving behavior, Machine learning, Motion constraint degree, Random parameters logit, Toll plaza diverging area, Traffic conflict
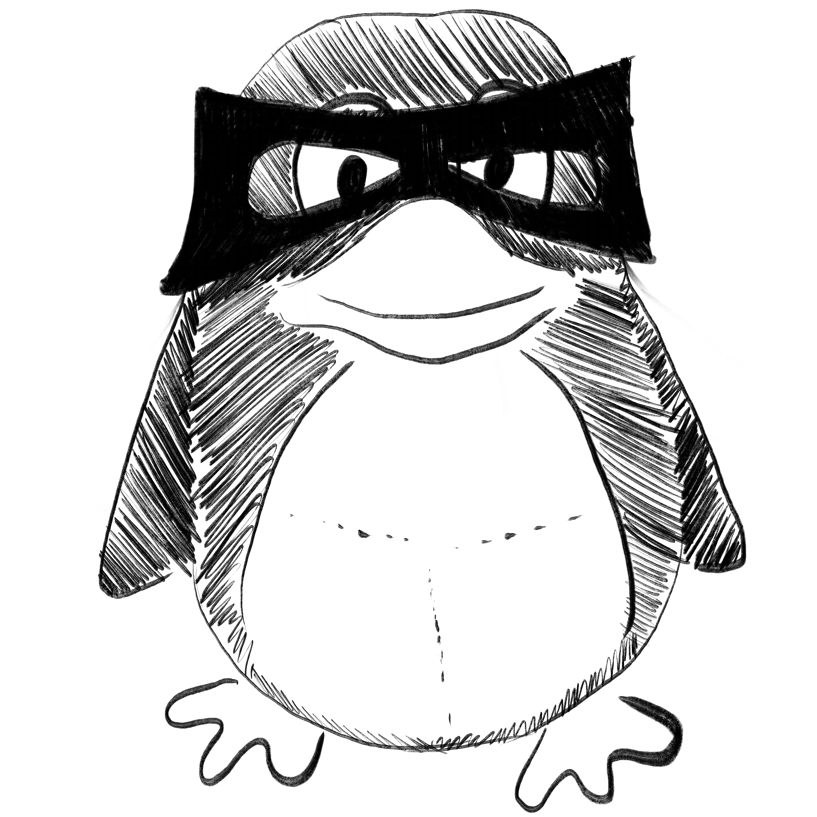
Contributions of various driving factors to air pollution events: Interpretability analysis from Machine learning perspective.
In Environment international
Li Tianshuai, Zhang Qingzhu, Peng Yanbo, Guan Xu, Li Lei, Mu Jiangshan, Wang Xinfeng, Yin Xianwei, Wang Qiao
2023-Mar-04
Air pollution, Machine learning, PDP, PM(2.5), Permutation importance, SHAP
Investigation of radiomics and deep convolutional neural networks approaches for glioma grading.
In Biomedical physics & engineering express
Aouadi Souha, Torfeh Tarraf, Yoganathan S A, Paloor Satheesh, Riyas Mohamed, Hammoud Rabih, Al-Hammadi Noora
2023-Mar-10
Benchmarking, CT, Deep learning, Glioma grading, Multi-contrast MRI, Radiomics
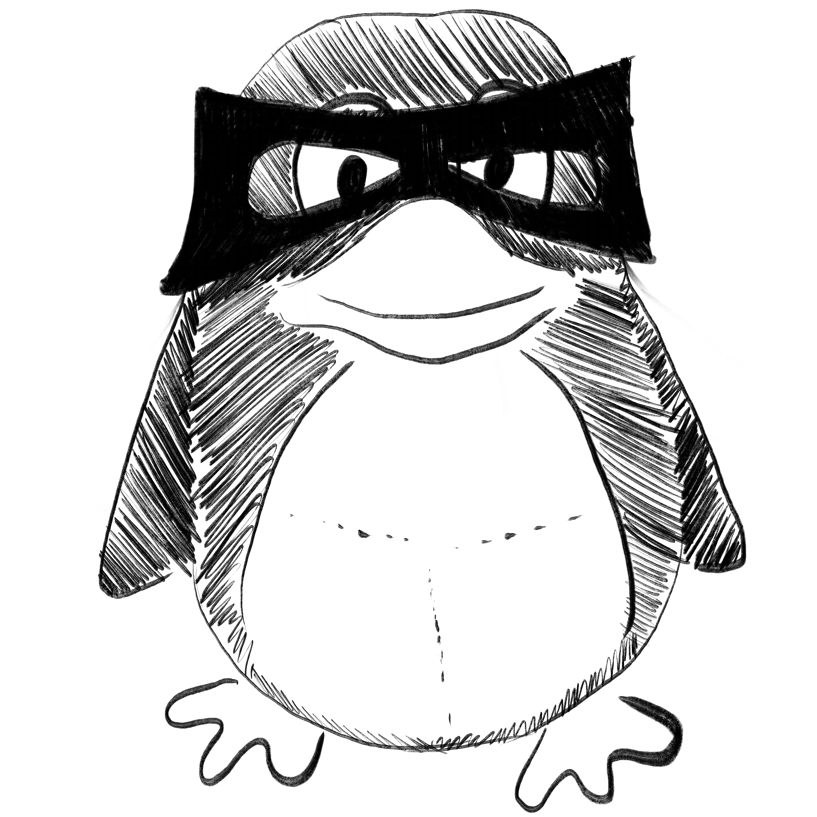
De novo design of small beta barrel proteins.
In Proceedings of the National Academy of Sciences of the United States of America
Kim David E, Jensen Davin R, Feldman David, Tischer Doug, Saleem Ayesha, Chow Cameron M, Li Xinting, Carter Lauren, Milles Lukas, Nguyen Hannah, Kang Alex, Bera Asim K, Peterson Francis C, Volkman Brian F, Ovchinnikov Sergey, Baker David
2023-Mar-14
high-throughput screening, machine learning, protein design, small beta barrels
Weekly Summary
Receive a weekly summary and discussion of the top papers of the week by leading researchers in the field.