Receive a weekly summary and discussion of the top papers of the week by leading researchers in the field.
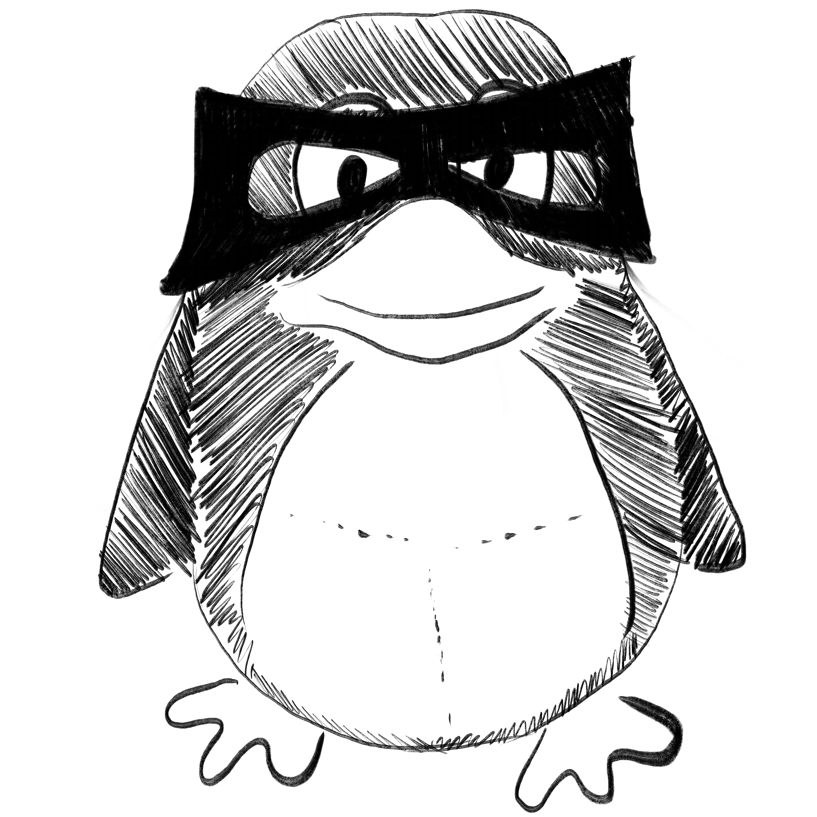
A cross-sectional study: a breathomics based pulmonary tuberculosis detection method.
In BMC infectious diseases ; h5-index 58.0
BACKGROUND :
METHOD :
RESULTS :
CONCLUSIONS :
Fu Liang, Wang Lei, Wang Haibo, Yang Min, Yang Qianting, Lin Yi, Guan Shanyi, Deng Yongcong, Liu Lei, Li Qingyun, He Mengqi, Zhang Peize, Chen Haibin, Deng Guofang
2023-Mar-10
Breathomics, Machine learning, Pulmonary tuberculosis, Volatile organic compounds
Moderation effects of serotype on dengue severity across pregnancy status in Mexico.
In BMC infectious diseases ; h5-index 58.0
BACKGROUND :
METHOD :
RESULTS :
CONCLUSION :
Annan Esther, Nguyen Uyen-Sa D T, Treviño Jesús, Wan Yaacob Wan Fairos, Mangla Sherry, Pathak Ashok Kumar, Nandy Rajesh, Haque Ubydul
2023-Mar-10
Dengue, Mexico, Pregnancy, Reproductive age, Serotype, Severe, Severity, Women
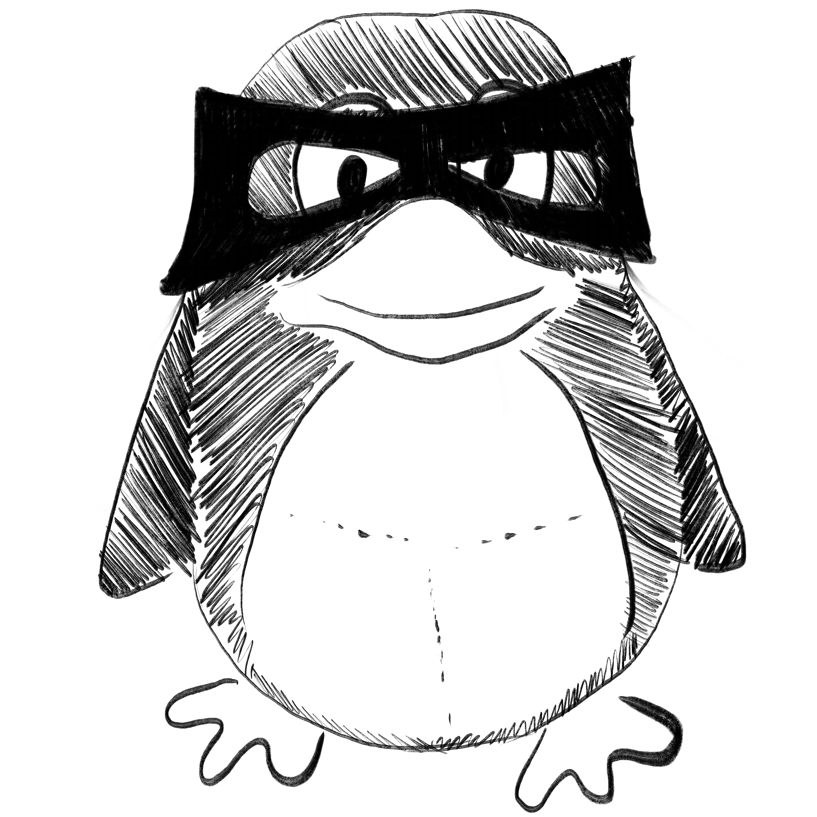
Big Data in Stroke: How to Use Big Data to Make the Next Management Decision.
In Neurotherapeutics : the journal of the American Society for Experimental NeuroTherapeutics
Liu Yuzhe, Luo Yuan, Naidech Andrew M
2023-Mar-10
Artificial intelligence, Big data, Deep learning, Machine learning, Stroke complications, Stroke management
A hybrid deep learning approach for COVID-19 detection based on genomic image processing techniques.
In Scientific reports ; h5-index 158.0
Hammad Muhammed S, Ghoneim Vidan F, Mabrouk Mai S, Al-Atabany Walid I
2023-Mar-10
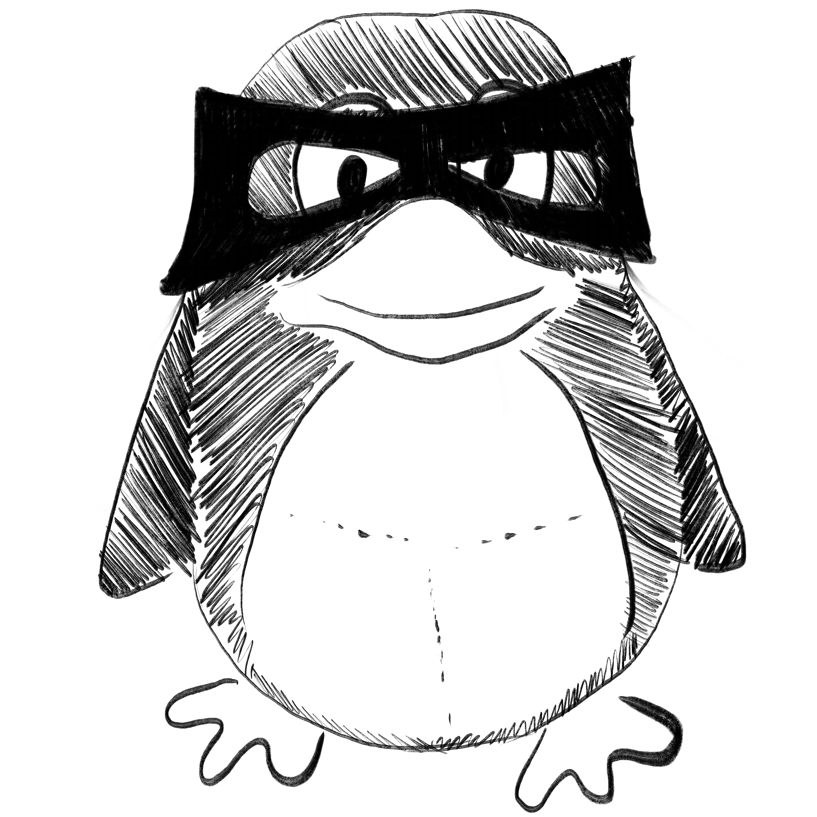
Predicting hospital emergency department visits accurately: A systematic review.
In The International journal of health planning and management
OBJECTIVES :
METHODS :
RESULTS :
CONCLUSIONS :
Silva Eduardo, Pereira Margarida F, Vieira Joana T, Ferreira-Coimbra João, Henriques Mariana, Rodrigues Nuno F
2023-Mar-10
emergency department, forecasting, hospital, predictive models, resource management, visits
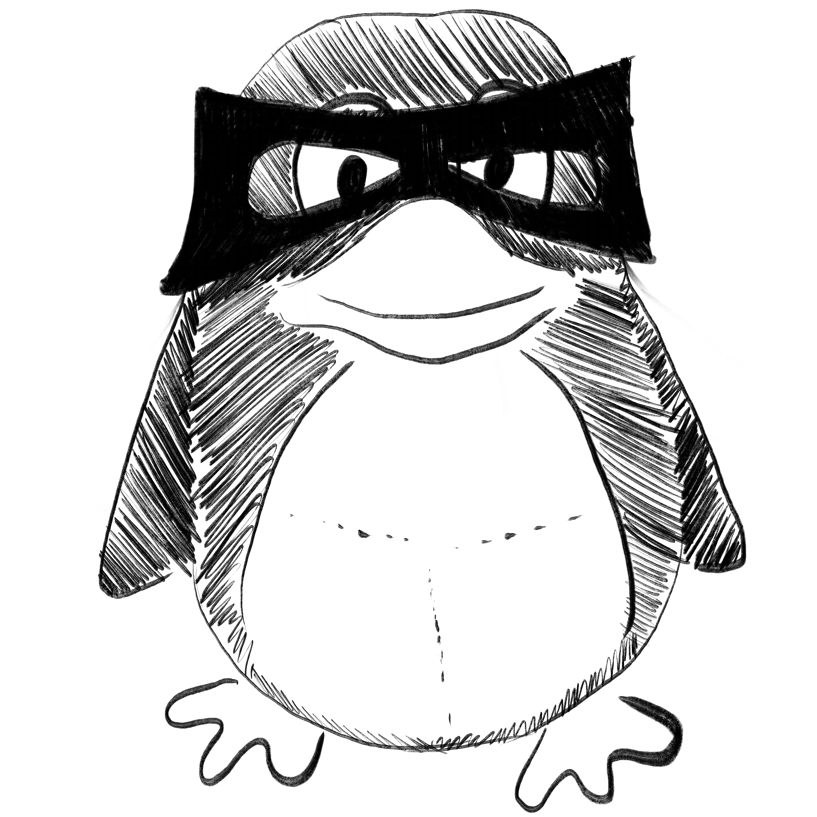
A review of the application of artificial intelligence to nuclear reactors: Where we are and what's next.
In Heliyon
Huang Qingyu, Peng Shinian, Deng Jian, Zeng Hui, Zhang Zhuo, Liu Yu, Yuan Peng
2023-Mar
Artificial intelligence, Causal learning, Nuclear reactors, SciML, XAI
Weekly Summary
Receive a weekly summary and discussion of the top papers of the week by leading researchers in the field.