Receive a weekly summary and discussion of the top papers of the week by leading researchers in the field.
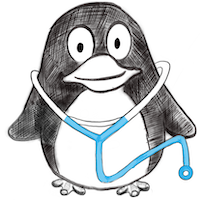
Acute and chronic stress alter behavioral laterality in dogs.
In Scientific reports ; h5-index 158.0
Salgirli Demirbas Yasemin, Isparta Sevim, Saral Begum, Keskin Yılmaz Nevra, Adıay Deniz, Matsui Hiroshi, Töre-Yargın Gülşen, Musa Saad Adam, Atilgan Durmus, Öztürk Hakan, Kul Bengi Cinar, Şafak C Etkin, Ocklenburg Sebastian, Güntürkün Onur
2023-Mar-11
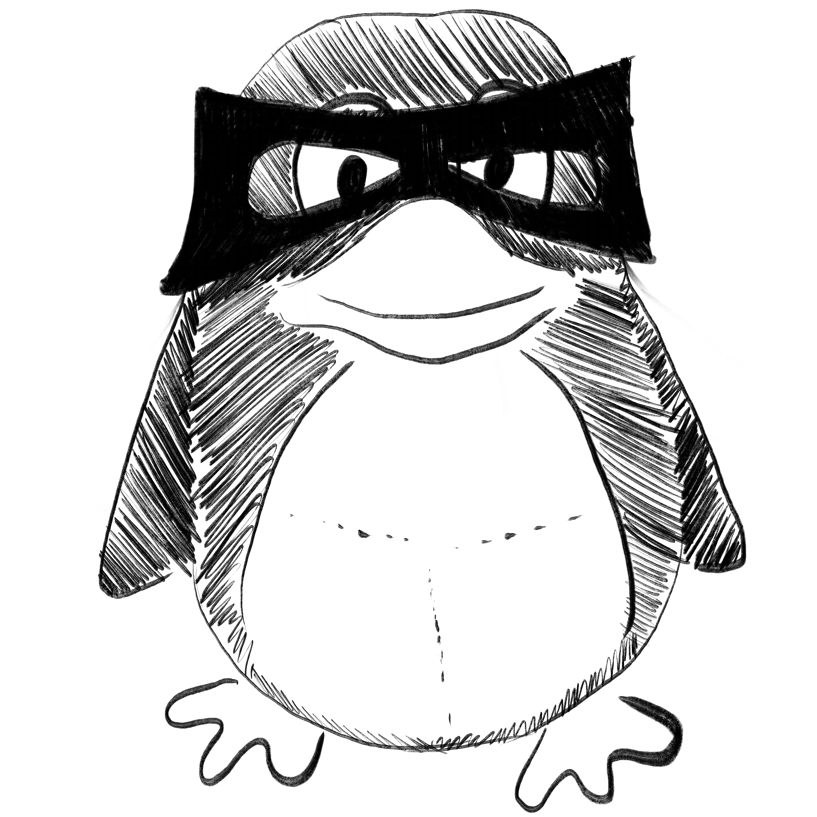
Predicting Potential Drug-Disease Associations Based on Hypergraph Learning with Subgraph Matching.
In Interdisciplinary sciences, computational life sciences
Wang Yuanxu, Song Jinmiao, Wei Mingjie, Duan Xiaodong
2023-Mar-11
Drug–disease associations, Hypergraph Unet, Hypergraph convolutional neural network, Subgraph matching
Optimizing Convolutional Neural Networks for Chronic Obstructive Pulmonary Disease Detection in Clinical Computed Tomography Imaging
ArXiv Preprint
Tina Dorosti, Manuel Schultheiss, Felix Hofmann, Luisa Kirchner, Theresa Urban, Franz Pfeiffer, Johannes Thalhammer, Florian Schaff, Tobias Lasser, Daniela Pfeiffer
2023-03-13
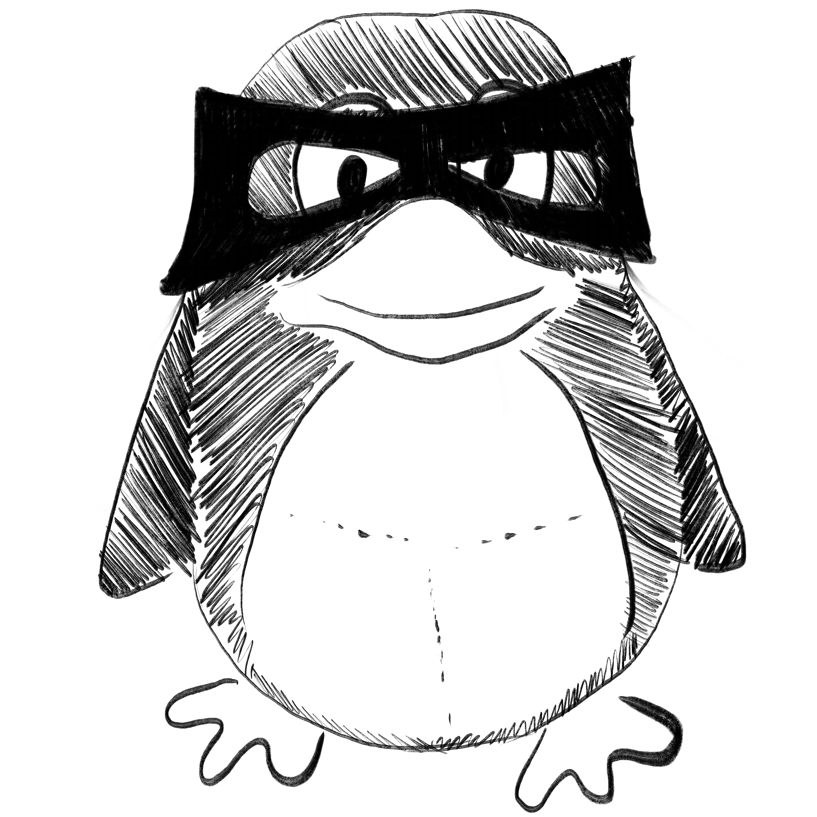
Occupant privacy perception, awareness, and preferences in smart office environments.
In Scientific reports ; h5-index 158.0
Li Beatrice, Tavakoli Arash, Heydarian Arsalan
2023-Mar-11
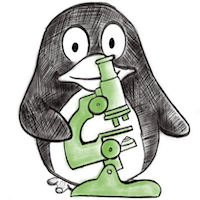
Learning Reduced-Order Models for Cardiovascular Simulations with Graph Neural Networks
ArXiv Preprint
Luca Pegolotti, Martin R. Pfaller, Natalia L. Rubio, Ke Ding, Rita Brugarolas Brufau, Eric Darve, Alison L. Marsden
2023-03-13
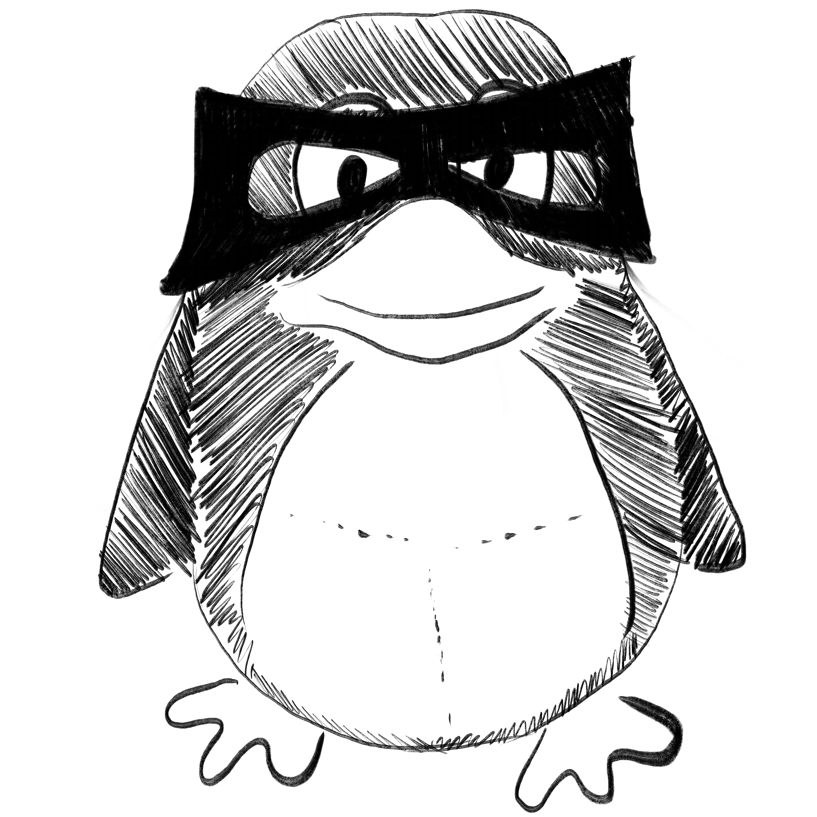
Novel architecture for gated recurrent unit autoencoder trained on time series from electronic health records enables detection of ICU patient subgroups.
In Scientific reports ; h5-index 158.0
Merkelbach Kilian, Schaper Steffen, Diedrich Christian, Fritsch Sebastian Johannes, Schuppert Andreas
2023-Mar-11
Weekly Summary
Receive a weekly summary and discussion of the top papers of the week by leading researchers in the field.