Receive a weekly summary and discussion of the top papers of the week by leading researchers in the field.
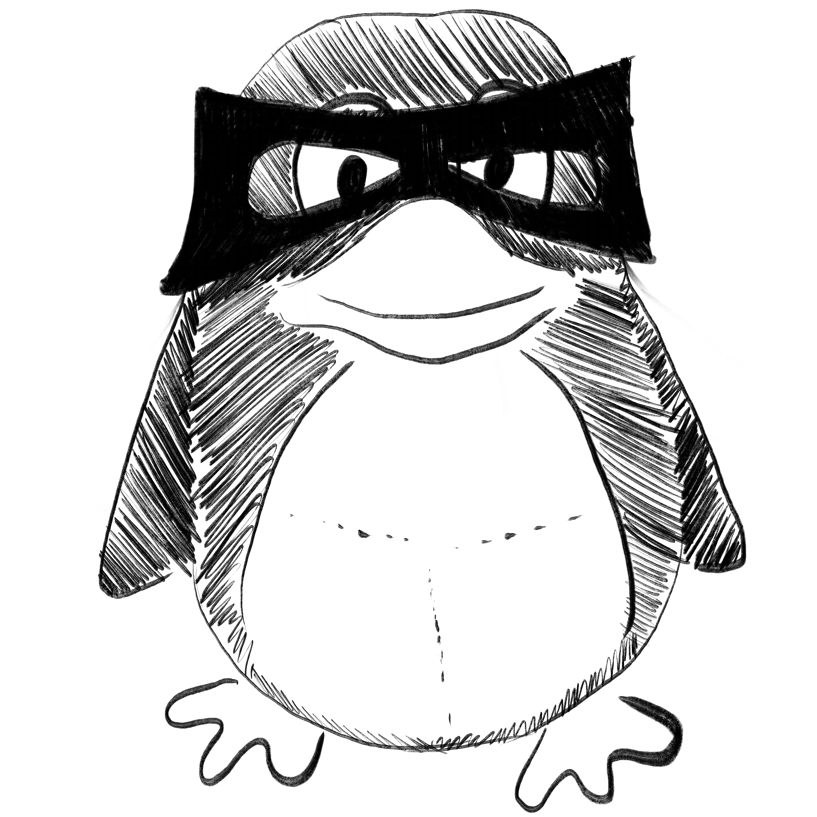
Lifelong Text-Audio Sentiment Analysis learning.
In Neural networks : the official journal of the International Neural Network Society
Lin Yuting, Ji Peng, Chen Xiuyi, He Zhongshi
2023-Feb-17
Cross-modality learning, Lifelong machine learning, Multi-task learning, Text-audio sentiment analysis
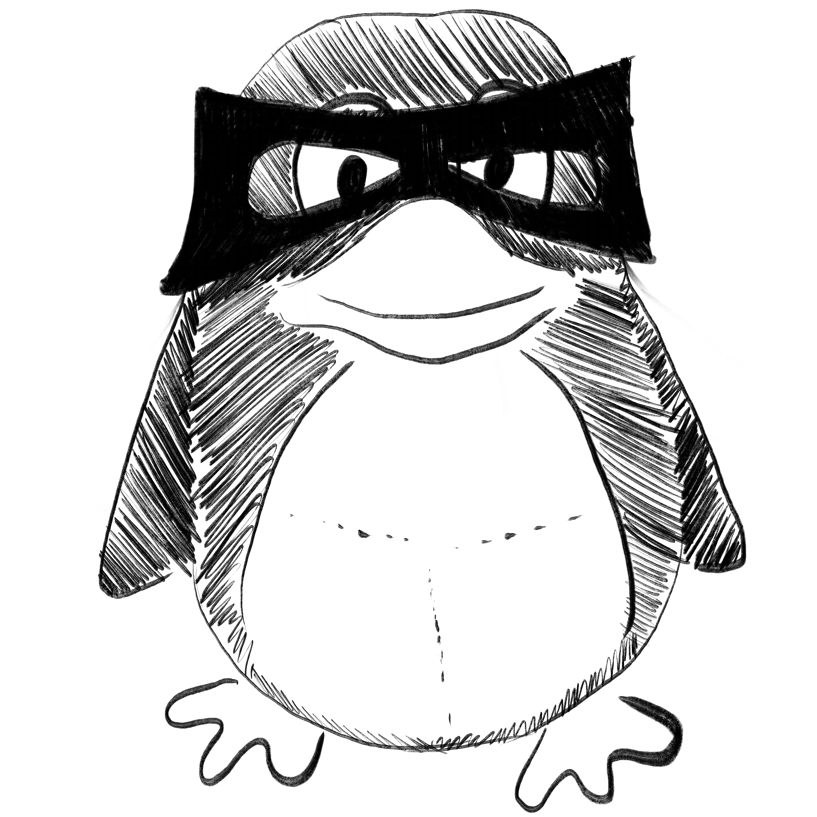
WDMNet: Modeling diverse variations of regional wind speed for multi-step predictions.
In Neural networks : the official journal of the International Neural Network Society
Ye Rui, Feng Shanshan, Li Xutao, Ye Yunming, Zhang Baoquan, Zhu Yan, Sun Yao, Wang Yaowei
2023-Feb-22
Deep learning, Diverse variations, PDEs construction, Regional wind speed prediction
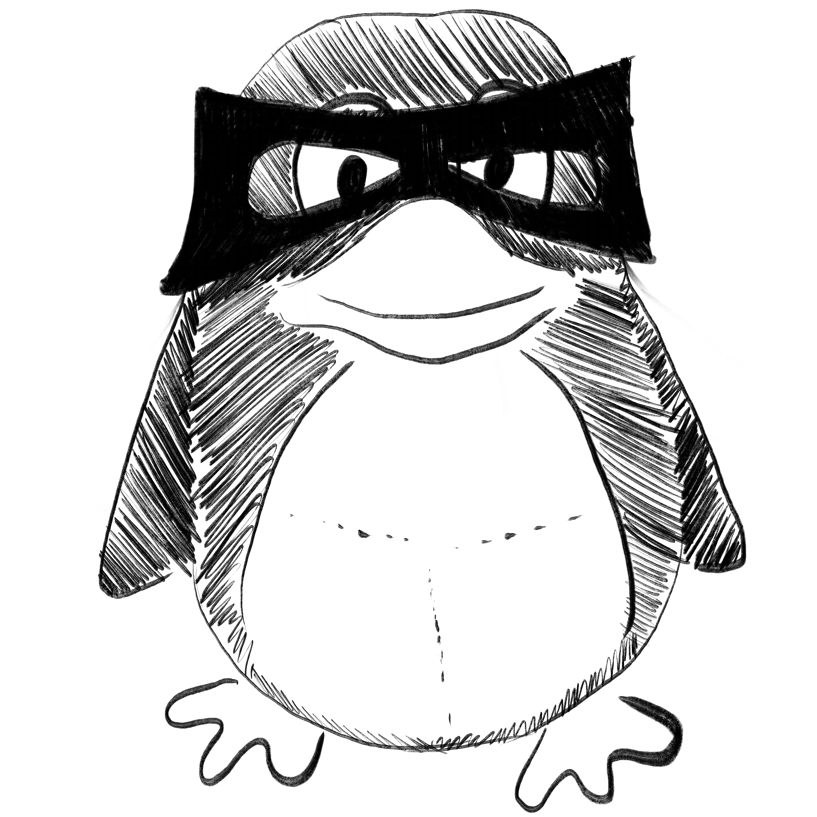
Machine learning for genetic prediction of chemotherapy toxicity in cervical cancer.
In Biomedicine & pharmacotherapy = Biomedecine & pharmacotherapie
BACKGROUND :
MATERIALS AND METHODS :
RESULTS :
CONCLUSION :
Guo Lu, Wang Wei, Xie Xiaodong, Wang Shuihua, Zhang Yudong
2023-Mar-10
Chemotherapy toxicity, Locally advanced cervical cancer, Machine learning, Neoadjuvant chemotherapy, PI3K/AKT pathway, Random forest, Single nucleotide polymorphisms
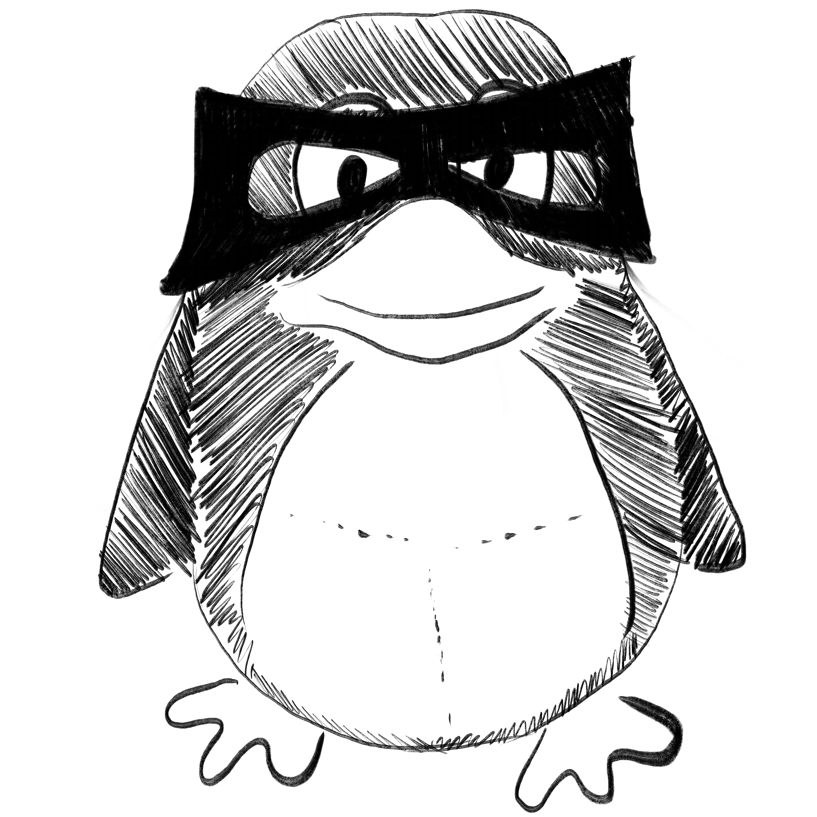
Is ChatGPT a valid author?
In Nurse education in practice ; h5-index 36.0
Teixeira da Silva Jaime A
2023-Mar-07
Artificial intelligence (AI), Authorship principles, COPE, Ethics, ICMJE, Responsibility
Convolutional neural network-based automated maxillary alveolar bone segmentation on cone-beam computed tomography images.
In Clinical oral implants research ; h5-index 55.0
OBJECTIVES :
MATERIAL AND METHODS :
RESULTS :
CONCLUSION :
Fontenele Rocharles Cavalcante, Gerhardt MaurĂcio do Nascimento, Picoli Fernando Fortes, Gerven Adriaan Van, Nomidis Stefanos, Willems Holger, Freitas Deborah Queiroz, Jacobs Reinhilde
2023-Mar-12
alveolar crest, artificial intelligence, cone-beam computed tomography, dental implant, jaw bone, maxilla, neural networks
A transformer architecture for retention time prediction in liquid chromatography mass spectrometry-based proteomics.
In Proteomics
Pham Thang V, Nguyen Vinh V, Vu Duong, Henneman Alex A, Richardson Robin A, Piersma Sander R, Jimenez Connie R
2023-Mar-12
DIA-MS, Deep learning, retention time prediction, spectral library, transformer architecture
Weekly Summary
Receive a weekly summary and discussion of the top papers of the week by leading researchers in the field.