Receive a weekly summary and discussion of the top papers of the week by leading researchers in the field.
Emerging Role of Artificial Intelligence in Diagnosis, Classification and Clinical Management of Glioma.
In Seminars in cancer biology
Luo Jiefeng, Pan Mika, Mo Ke, Mao Yingwei, Zou Donghua
2023-Mar-10
artificial intelligence, clinical practice, deep learning, digital pathology, glioma, machine learning, radiology
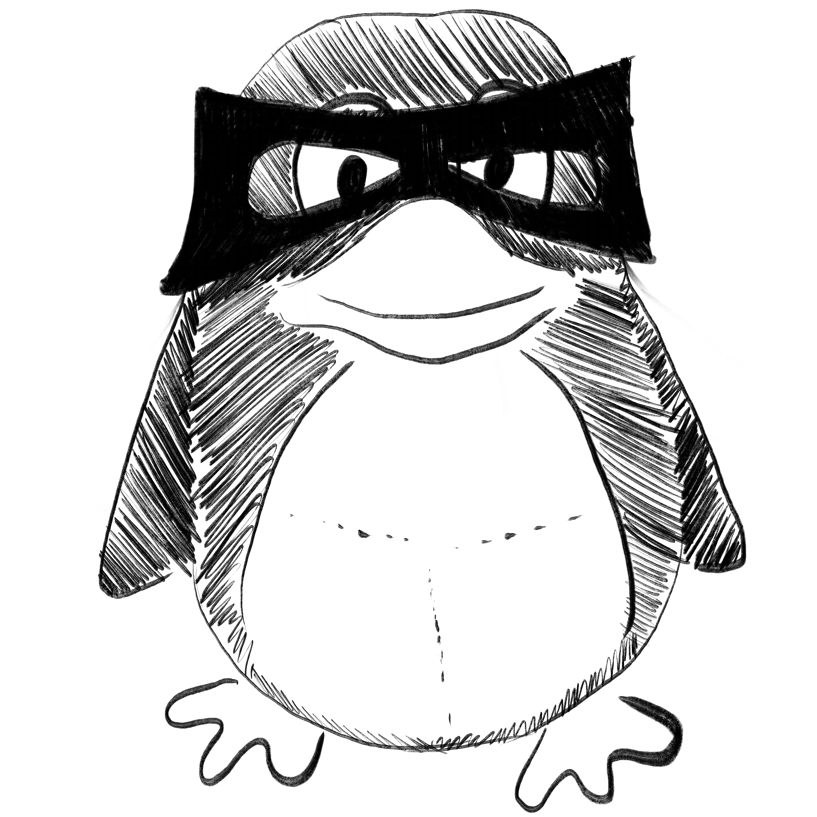
AlphaFold, allosteric, and orthosteric drug discovery: Ways forward.
In Drug discovery today ; h5-index 68.0
Nussinov Ruth, Zhang Mingzhen, Liu Yonglan, Jang Hyunbum
2023-Mar-10
ESMfold, activating mutations, artificial intelligence, inhibitors, machine learning, molecular dynamics simulations, orthosteric drugs
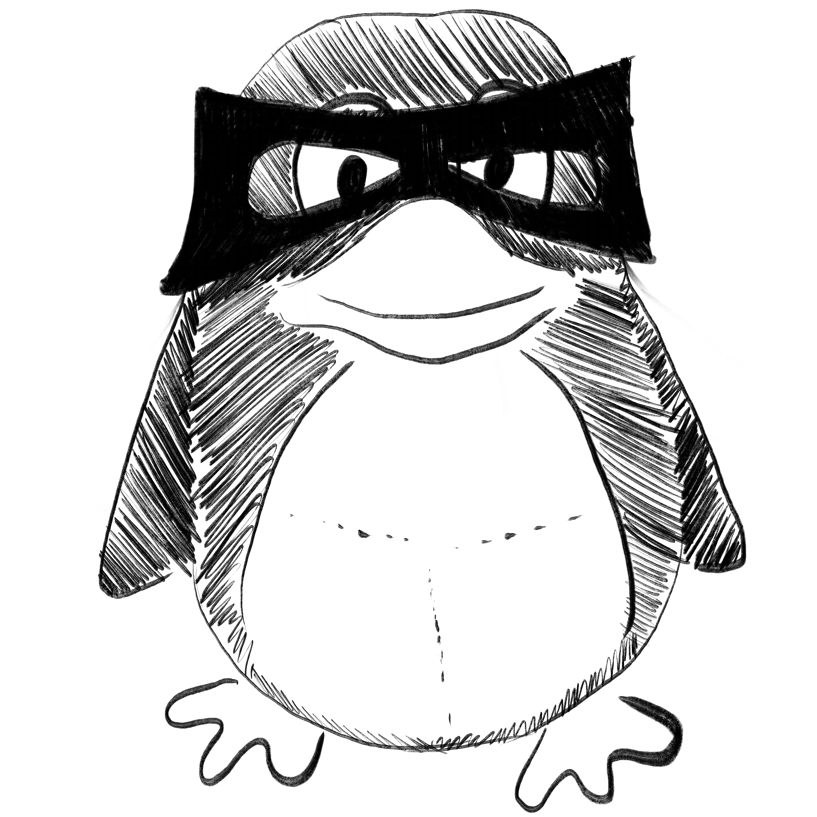
Machine learning in additive manufacturing & Microfluidics for smarter and safer drug delivery systems.
In International journal of pharmaceutics ; h5-index 67.0
Dedeloudi Aikaterini, Weaver Edward, Lamprou Dimitrios A
2023-Mar-10
3D printing, Additive manufacturing, Algorithms, Machine learning, Microfluidics, Quality by design
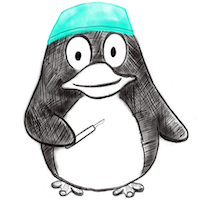
A Machine Learning-Based Prediction of Diabetes Insipidus in Patients Undergoing Endoscopic Transsphenoidal Surgery for Pituitary Adenoma.
In World neurosurgery ; h5-index 47.0
BACKGROUND :
METHODS :
RESULTS :
CONCLUSIONS :
Hou Siyuan, Li Xiaomin, Meng Fanyue, Liu Shaokun, Wang Zhenlin
2023-Mar-10
diabetes insipidus, machine learning, pituitary adenoma, prediction model, transsphenoidal surgery
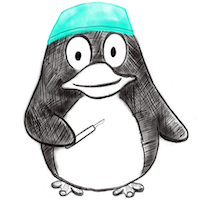
The imitation game: a review of the use of artificial intelligence in colonoscopy, and endoscopists' perceptions thereof.
In Annals of coloproctology
Tham Sarah, Koh Frederick H, Ladlad Jasmine, Chue Koy-Min, Lin Cui-Li, Teo Eng-Kiong, Foo Fung-Joon
2023-Mar-10
Adenoma, Artificial intelligence, Biodmedical technology assessment, Colonic polyps, Colonoscopy
Predicting muscle invasion in bladder cancer based on MRI: A comparison of radiomics, and single-task and multi-task deep learning.
In Computer methods and programs in biomedicine
BACKGROUND AND OBJECTIVES :
METHODS :
RESULTS :
CONCLUSIONS :
Li Jianpeng, Qiu Zhengxuan, Cao Kangyang, Deng Lei, Zhang Weijing, Xie Chuanmiao, Yang Shuiqing, Yue Peiyan, Zhong Jian, Lyu Jiegeng, Huang Xiang, Zhang Kunlin, Zou Yujian, Huang Bingsheng
2023-Mar-05
Bladder cancer, Deep learning, Magnetic resonance imaging, Multi-task learning, Muscle invasion, Radiomics
Weekly Summary
Receive a weekly summary and discussion of the top papers of the week by leading researchers in the field.