Receive a weekly summary and discussion of the top papers of the week by leading researchers in the field.
Deep Learning-Based Computed Tomography Image Standardization to Improve Generalizability of Deep Learning-Based Hepatic Segmentation.
In Korean journal of radiology
OBJECTIVE :
MATERIALS AND METHODS :
RESULTS :
CONCLUSION :
Lee Seul Bi, Hong Youngtaek, Cho Yeon Jin, Jeong Dawun, Lee Jina, Yoon Soon Ho, Lee Seunghyun, Choi Young Hun, Cheon Jung-Eun
2023-Mar-07
Artificial intelligence, Automated segmentation, Image conversion, Quality control, Reproducibility
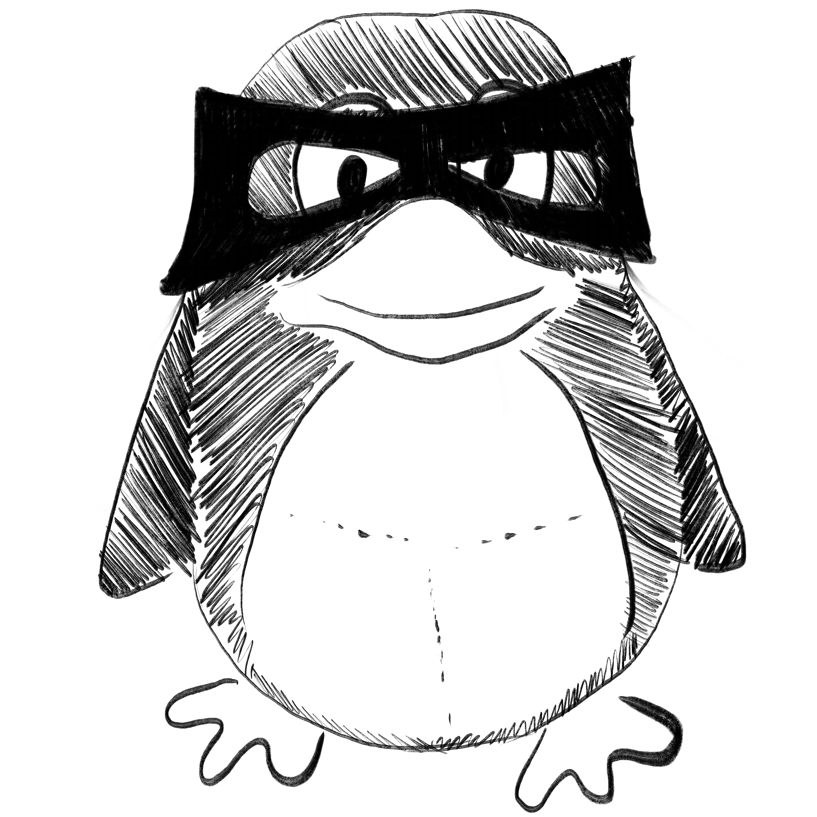
Classification of household microplastics using a multi-model approach based on Raman spectroscopy.
In Chemosphere
Feng Zikang, Zheng Lina, Liu Jia
2023-Mar-10
Classification, Environment stress, Household microplastics, Multi-model approach, Raman spectroscopy
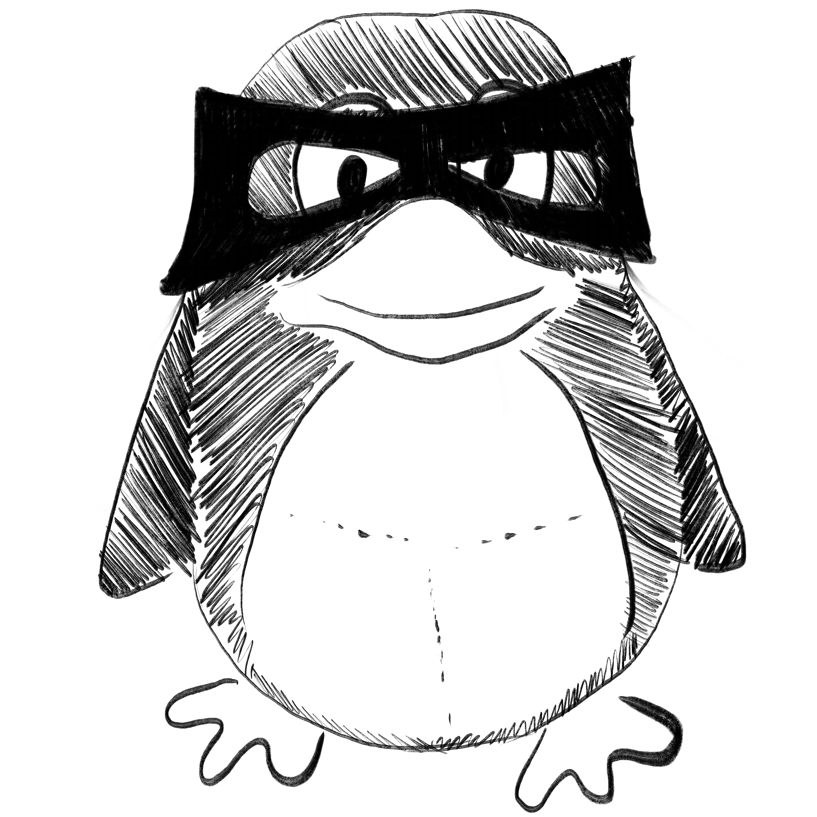
New proposal of viral genome representation applied in the classification of SARS-CoV-2 with deep learning.
In BMC bioinformatics
BACKGROUND :
RESULTS :
CONCLUSIONS :
de Souza LuĂsa C, Azevedo Karolayne S, de Souza Jackson G, Barbosa Raquel de M, Fernandes Marcelo A C
2023-Mar-11
CGR DFT, COVID-19, Deep learning, GSP, SARS-CoV-2
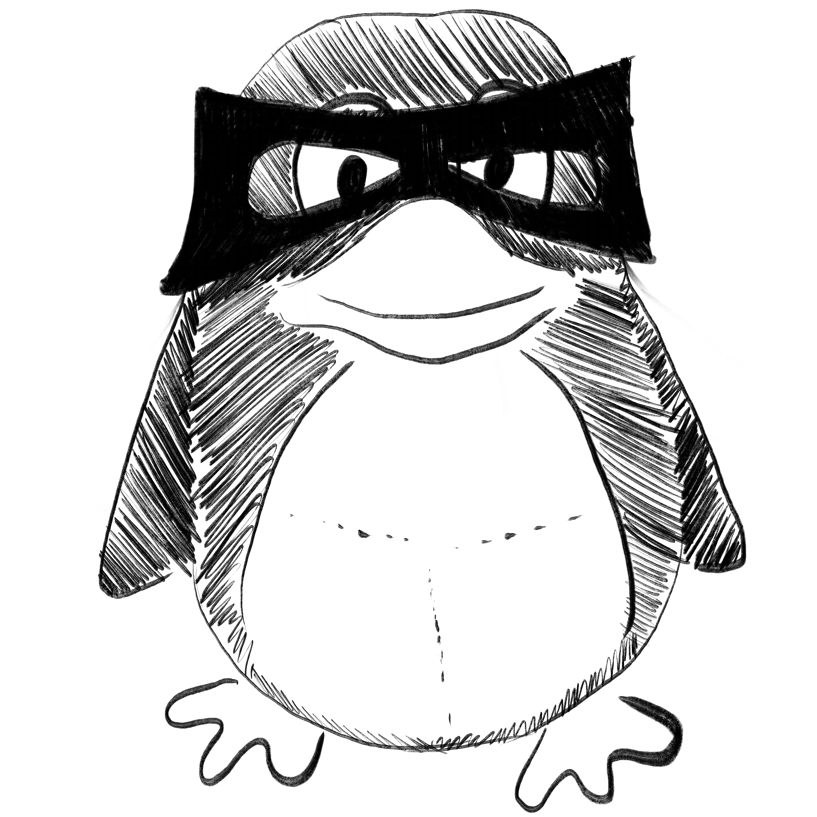
A novel use of an artificially intelligent Chatbot and a live, synchronous virtual question-and answer session for fellowship recruitment.
In BMC medical education
INTRODUCTION :
METHODS :
RESULTS :
CONCLUSION :
Yi Peter K, Ray Neil D, Segall Noa
2023-Mar-11
Artificial intelligence, Graduate medical education, Innovation and technology, Recruitment, Social media
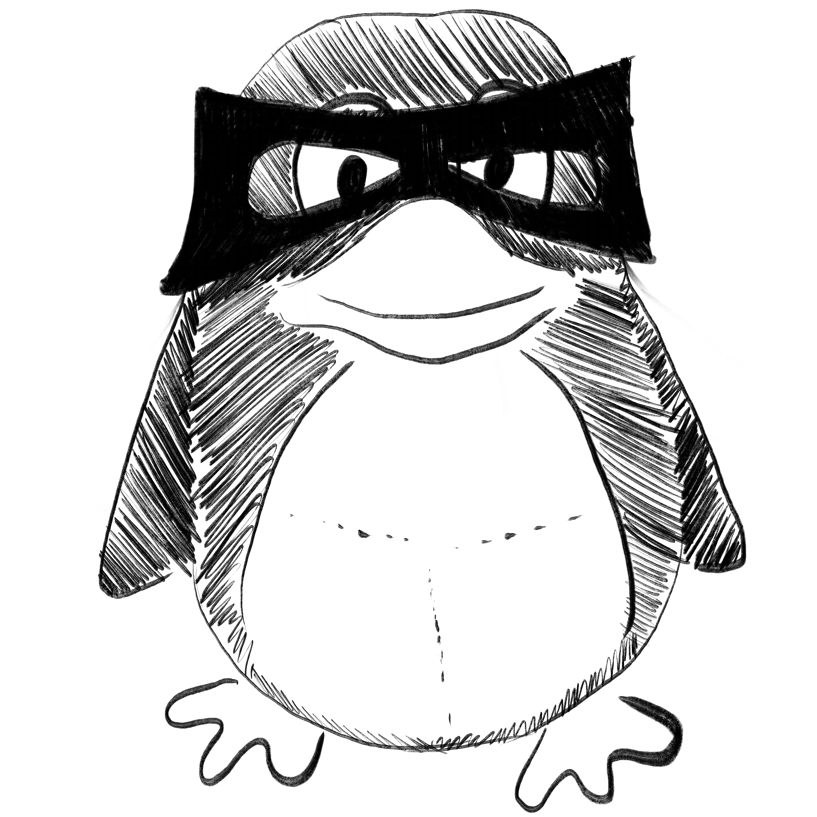
Bandit-supported care planning for older people with complex health and care needs
ArXiv Preprint
Gi-Soo Kim, Young Suh Hong, Tae Hoon Lee, Myunghee Cho Paik, Hongsoo Kim
2023-03-13
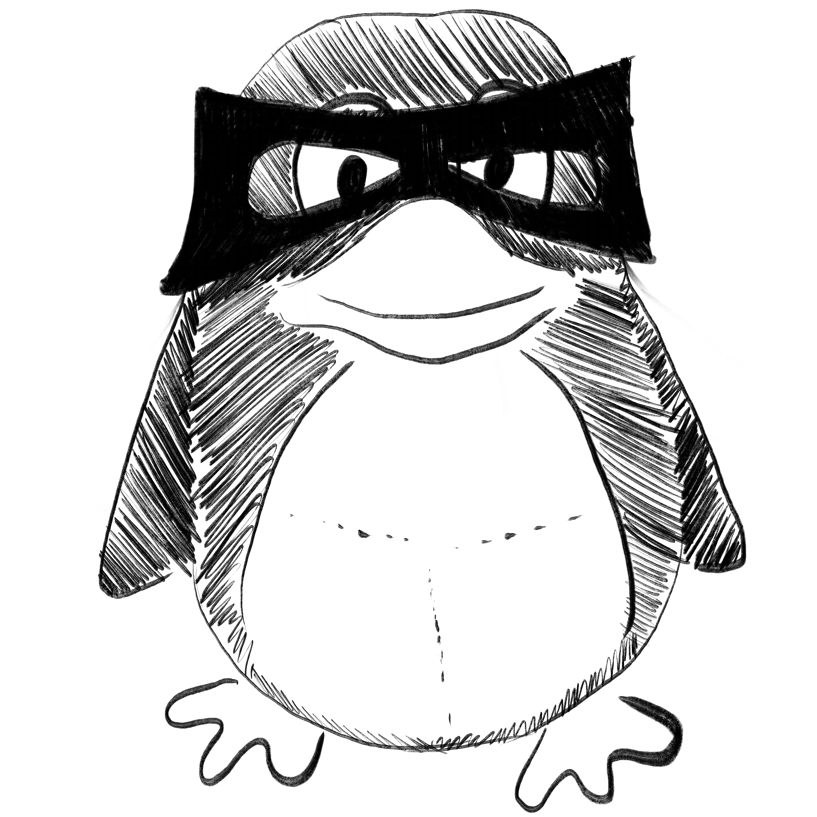
Comparison of photocatalysis and photolysis of 2,2,4,4-tetrabromodiphenyl ether (BDE-47): Operational parameters, kinetic studies, and data validation using three modern machine learning models.
In Chemosphere
Motamedi Mahsa, Yerushalmi Laleh, Haghighat Fariborz, Chen Zhi, Zhuang Yanbin
2023-Mar-10
Direct photolysis, Machine learning, PBDEs, Photocatalysis
Weekly Summary
Receive a weekly summary and discussion of the top papers of the week by leading researchers in the field.