Receive a weekly summary and discussion of the top papers of the week by leading researchers in the field.
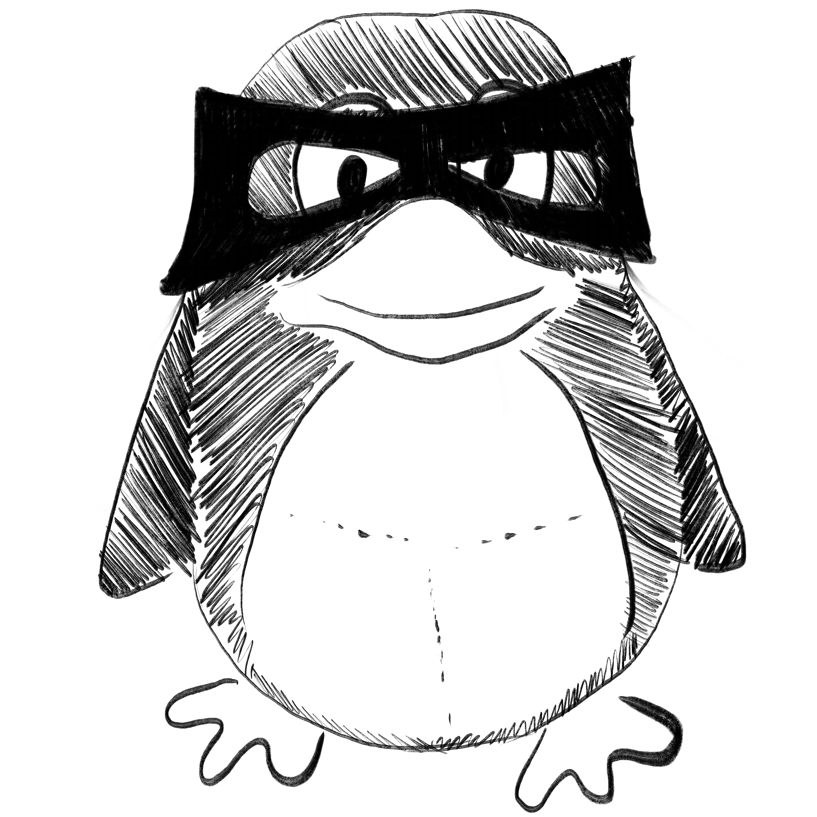
Triadic influence as a proxy for compatibility in social relationships.
In Proceedings of the National Academy of Sciences of the United States of America
Ruiz-García Miguel, Ozaita Juan, Pereda María, Alfonso Antonio, Brañas-Garza Pablo, Cuesta José A, Sánchez Angel
2023-Mar-28
machine learning, relationship prediction, social networks, triadic influence
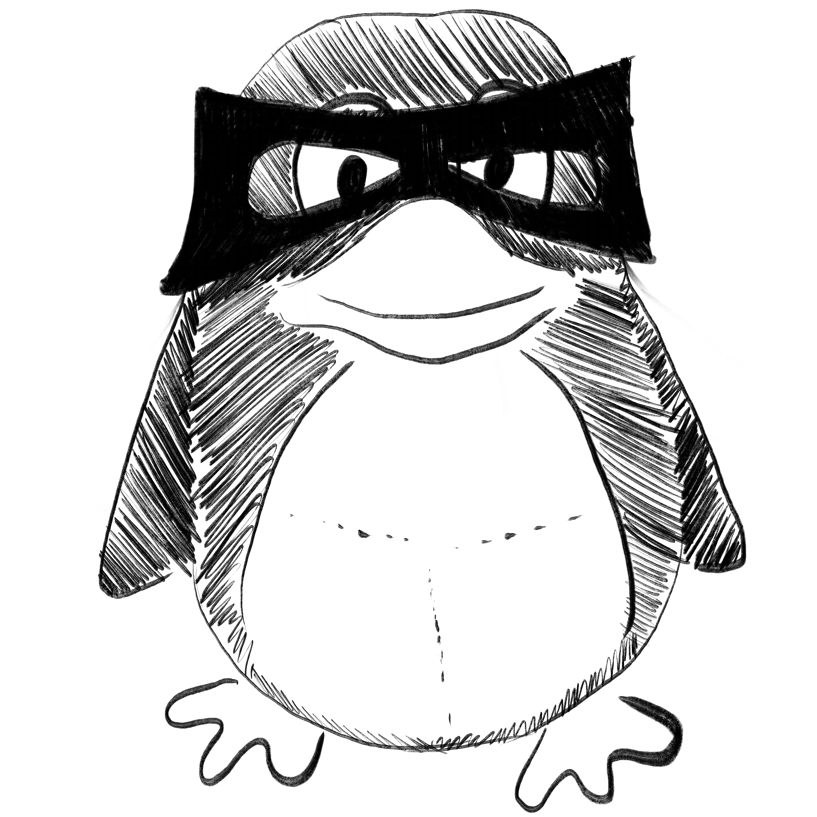
Extreme Gradient Boosting to Predict Atomic Layer Deposition for Platinum Nano-Film Coating.
In Langmuir : the ACS journal of surfaces and colloids
Yoon Sung-Ho, Jeon Jun-Hyeok, Cho Seung-Beom, Nacpil Edric John Cruz, Jeon Il, Choi Jae-Boong, Kim Hyeongkeun
2023-Mar-22
Virtual clinical trial based on outcome modeling with iteratively redistributed extrapolation data.
In Radiological physics and technology
Oguma Kohei, Magome Taiki, Someya Masanori, Hasegawa Tomokazu, Sakata Koh-Ichi
2023-Mar-22
Extrapolation data, Outcome modeling, Outcome prediction, Radiotherapy, Virtual clinical trial
QuantImage v2: a comprehensive and integrated physician-centered cloud platform for radiomics and machine learning research.
In European radiology experimental
BACKGROUND :
METHODS :
RESULTS :
CONCLUSIONS :
Abler Daniel, Schaer Roger, Oreiller Valentin, Verma Himanshu, Reichenbach Julien, Aidonopoulos Orfeas, Evéquoz Florian, Jreige Mario, Prior John O, Depeursinge Adrien
2023-Mar-22
Artificial intelligence, Biomarkers, Cloud computing, Decision support techniques, Radiomics
Applications of deep learning to reduce the need for iodinated contrast media for CT imaging: a systematic review.
In International journal of computer assisted radiology and surgery
PURPOSE :
METHODS :
RESULTS :
CONCLUSION :
Azarfar Ghazal, Ko Seok-Bum, Adams Scott J, Babyn Paul S
2023-Mar-22
Computed tomography, Contrast enhancement, Deep learning, Iodinated contrast media reduction
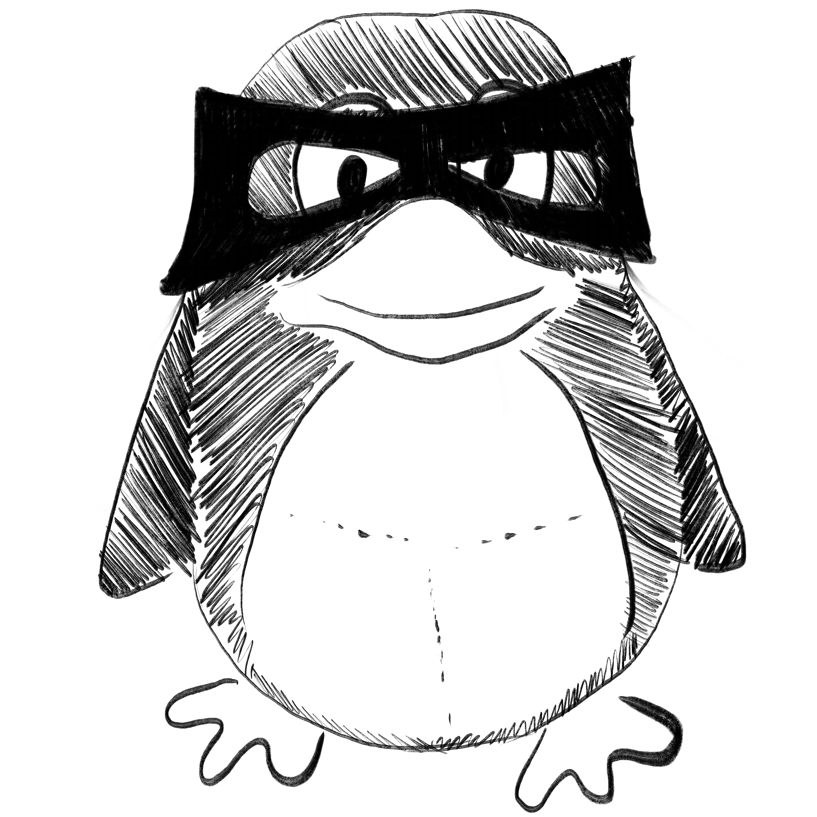
Machine learning based combination of multi-omics data for subgroup identification in non-small cell lung cancer.
In Scientific reports ; h5-index 158.0
Khadirnaikar Seema, Shukla Sudhanshu, Prasanna S R M
2023-Mar-21
Weekly Summary
Receive a weekly summary and discussion of the top papers of the week by leading researchers in the field.