Receive a weekly summary and discussion of the top papers of the week by leading researchers in the field.
Prediction of patient choice tendency in medical decision-making based on machine learning algorithm.
In Frontiers in public health
OBJECTIVE :
METHOD :
RESULTS :
CONCLUSION :
Lyu Yuwen, Xu Qian, Yang Zhenchao, Liu Junrong
2023
assistance systems, machine learning algorithm, medical decision-making, patient choice tendency, prediction
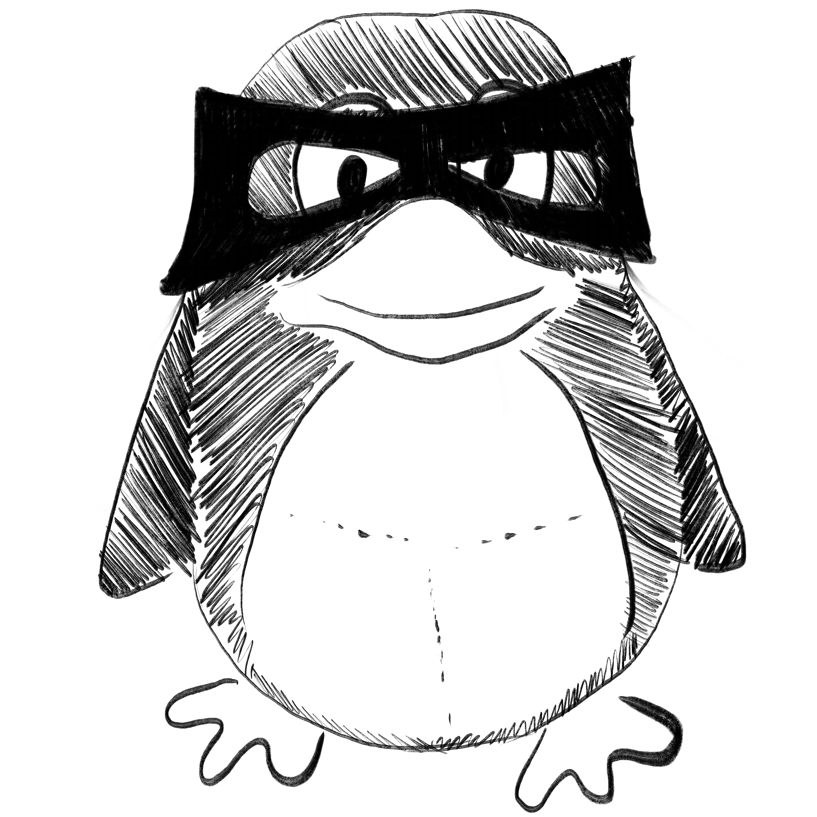
Big data and infectious disease epidemiology: A bibliometric analysis and research agenda.
In Interactive journal of medical research
BACKGROUND :
OBJECTIVE :
METHODS :
RESULTS :
CONCLUSIONS :
Amusa Lateef Babatunde, Twinomurinzi Hossana, Phalane Edith, Phaswana-Mafuya Refilwe Nancy
2022-Nov-29
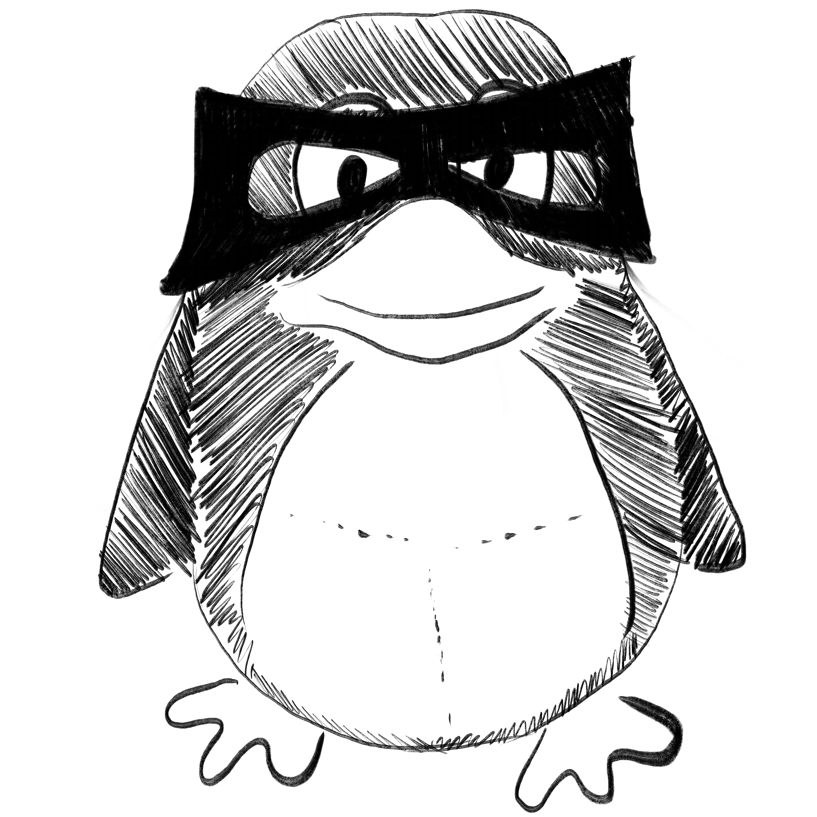
Detecting amyloid-β positivity using regions of interest from structural MRIs.
In European journal of neurology
BACKGROUND :
METHODS :
RESULTS :
CONCLUSIONS :
Hwang Jeongyoung, Park Hee Kyung, Yoon Hai-Jeon, Jeong Jee Hyang, Lee Hyunju
2023-Mar-13
“Alzheimers disease”, amyloid-β, left entorhinal cortical region, machine learning, sMRI
Assessing the contributions of modifiable risk factors to serious falls and fragility fractures among older persons living with HIV.
In Journal of the American Geriatrics Society ; h5-index 64.0
BACKGROUND :
METHODS :
RESULTS :
CONCLUSIONS :
Womack Julie A, Murphy Terrence E, Leo-Summers Linda, Bates Jonathan, Jarad Samah, Gill Thomas M, Hsieh Evelyn, Rodriguez-Barradas Maria C, Tien Phyllis C, Yin Michael T, Brandt Cynthia A, Justice Amy C
2023-Mar-13
HIV, LE-AAF, falls, fragility fractures
Deep Learning Algorithm Enables Cerebral Venous Thrombosis Detection With Routine Brain Magnetic Resonance Imaging.
In Stroke ; h5-index 83.0
BACKGROUND :
METHODS :
RESULTS :
CONCLUSIONS :
Yang Xiaoxu, Yu Pengxin, Zhang Haoyue, Zhang Rongguo, Liu Yuehong, Li Haoyuan, Sun Penghui, Liu Xin, Wu Yu, Jia Xiuqin, Duan Jiangang, Ji Xunming, Yang Qi
2023-Mar-13
algorithm, area under the curve, brain, cerebral venous thrombosis, magnetic resonance imaging
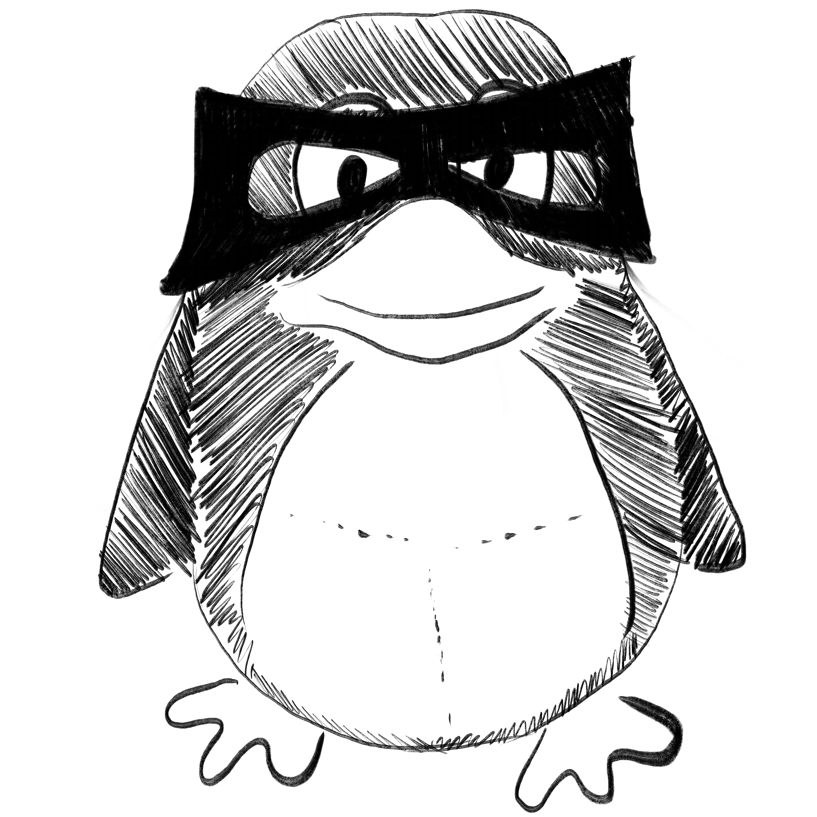
Two Routes to Alzheimer's Disease Based on Differential Structural Changes in Key Brain Regions.
In Journal of Alzheimer's disease : JAD
BACKGROUND :
OBJECTIVE :
METHODS :
RESULTS :
CONCLUSION :
Hollenbenders Yasmin, Pobiruchin Monika, Reichenbach Alexandra
2023-Mar-06
Alzheimer’s Disease Neuroimaging Initiative, Alzheimer’s disease, brain atrophy, clustering, hidden Markov model, longitudinal data, magnetic resonance imaging, patient stratification, subtype
Weekly Summary
Receive a weekly summary and discussion of the top papers of the week by leading researchers in the field.