Receive a weekly summary and discussion of the top papers of the week by leading researchers in the field.
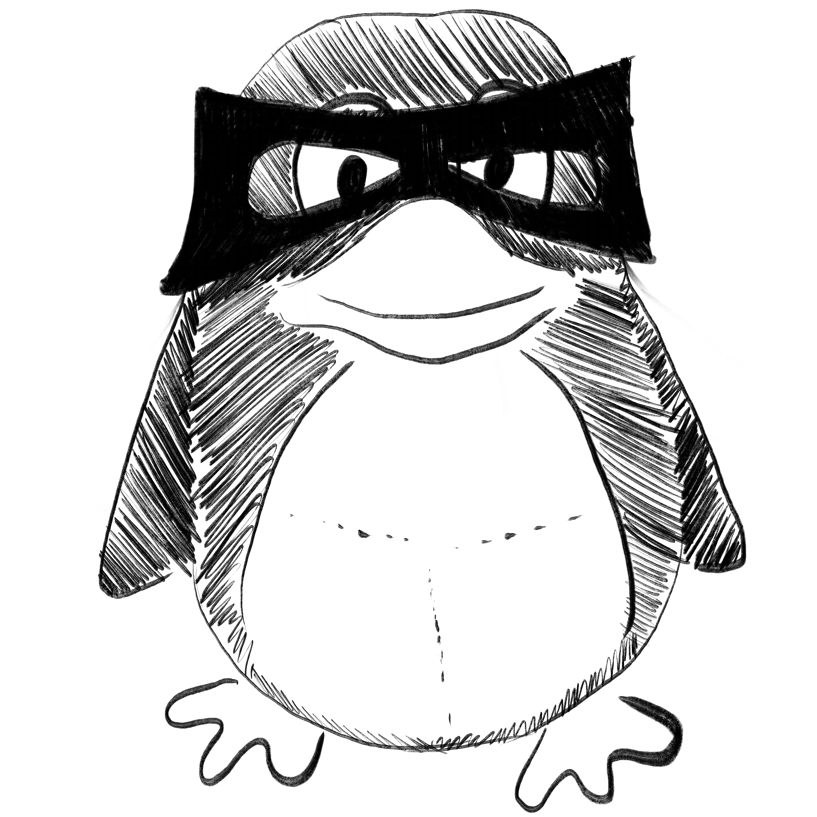
Evaluation of the Morphological and Biological Functions of Vascularized Microphysiological Systems with Supervised Machine Learning.
In Annals of biomedical engineering ; h5-index 52.0
Tronolone James J, Mathur Tanmay, Chaftari Christopher P, Jain Abhishek
2023-Mar-13
Data science, Machine learning, Microphysiological systems, Vascularization
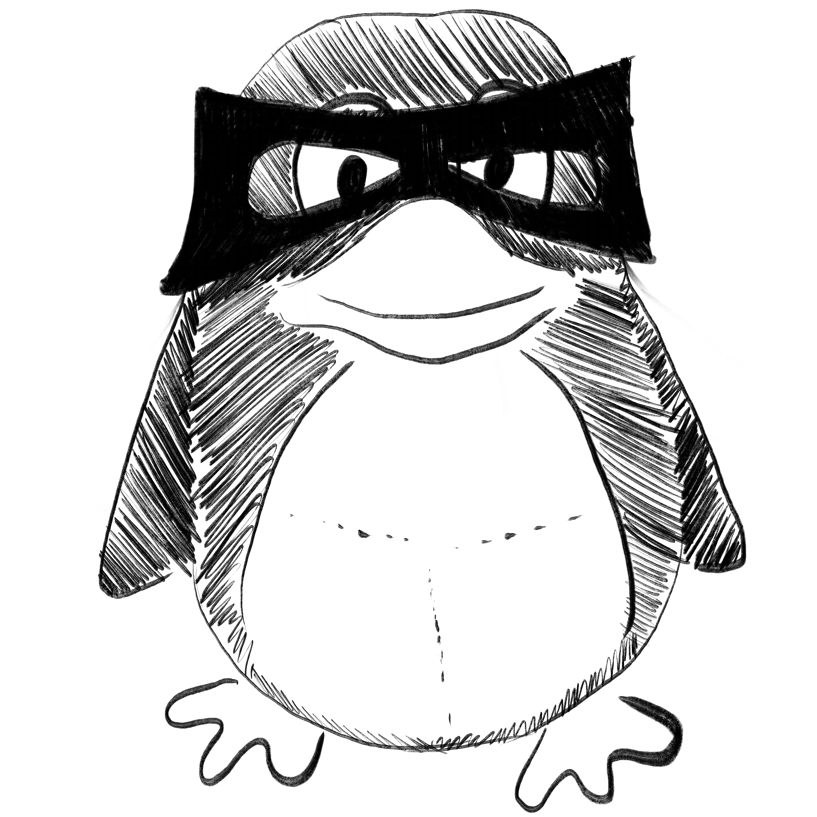
Predicting drug shortages using pharmacy data and machine learning.
In Health care management science
Pall Raman, Gauthier Yvan, Auer Sofia, Mowaswes Walid
2023-Mar-13
Analytics, Drugs, Machine learning, Pharmacies, Shortages, Supply chain, Therapeutics
A survey of ASER members on artificial intelligence in emergency radiology: trends, perceptions, and expectations.
In Emergency radiology
PURPOSE :
METHODS :
RESULTS :
CONCLUSION :
Agrawal Anjali, Khatri Garvit D, Khurana Bharti, Sodickson Aaron D, Liang Yuanyuan, Dreizin David
2023-Mar-13
Artificial intelligence, Computer-aided detection, Emergency, Emergency radiology, Imaging, Machine learning, Radiology, Survey, Trauma
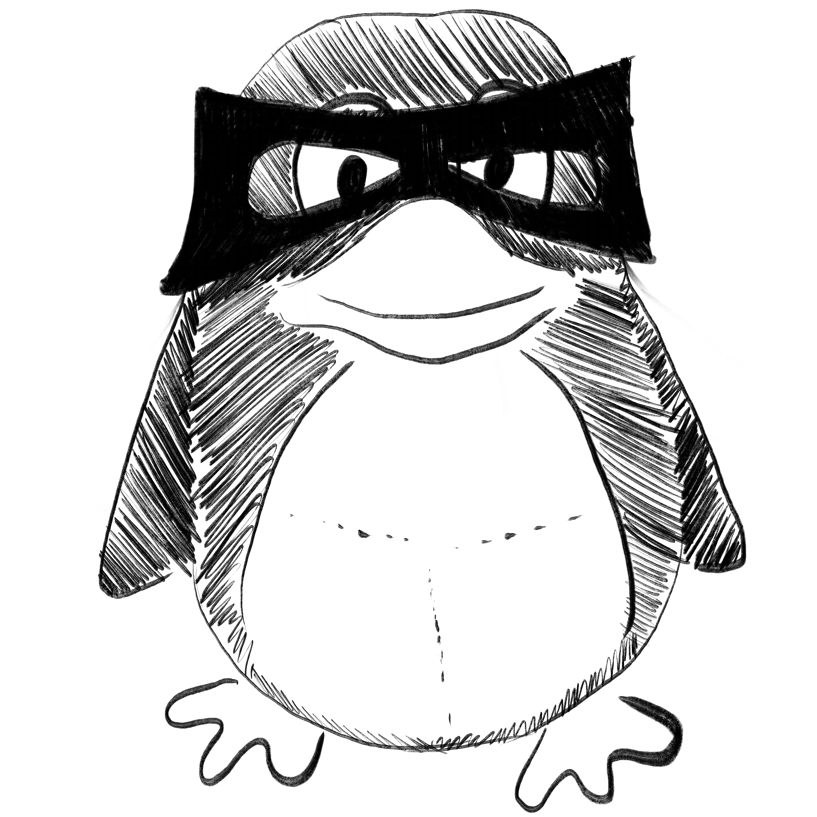
Attitudes of Different Religions Toward Surrogacy: Analysis of 11 Countries' Situation Using Machine Learning Approach and Artificial Neural Networks.
In Journal of religion and health
Yıldız Metin, Felix Ezomo Ojeiru, Ademiju Olugbenga, Noibi Tajudeen Oluwafemi, Gomes Roseline Florence, Tanimowo Abraham, Tayyeb Muhammed, Khadka Ram Bahadur, Rhino Andrianirina, Yildiz Rabia, Ramazanzadegan Kiarash, Yildirim Mehmet Salih, Solmaz Ebru, Haylı Çiğdem Müge, Şengan Aylin
2023-Mar-13
Attitude, Religious belief, Surrogacy
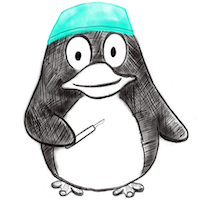
Artificial intelligence applications and ethical challenges in oral and maxillo-facial cosmetic surgery: a narrative review.
In Maxillofacial plastic and reconstructive surgery
Rokhshad Rata, Keyhan Seied Omid, Yousefi Parisa
2023-Mar-13
Artificial intelligence, Deep learning, Machine learning, Orthognathic surgery, Rhinoplasty
Differentiating peritoneal tuberculosis and peritoneal carcinomatosis based on a machine learning model with CT: a multicentre study.
In Abdominal radiology (New York)
PURPOSE :
METHODS :
RESULTS :
CONCLUSION :
Pang Yu, Li Ye, Xu Dong, Sun Xiaoli, Hou Dailun
2023-Mar-13
Computed tomography, Machine learning, Peritoneal carcinomatosis, Peritoneal tuberculosis
Weekly Summary
Receive a weekly summary and discussion of the top papers of the week by leading researchers in the field.