Receive a weekly summary and discussion of the top papers of the week by leading researchers in the field.
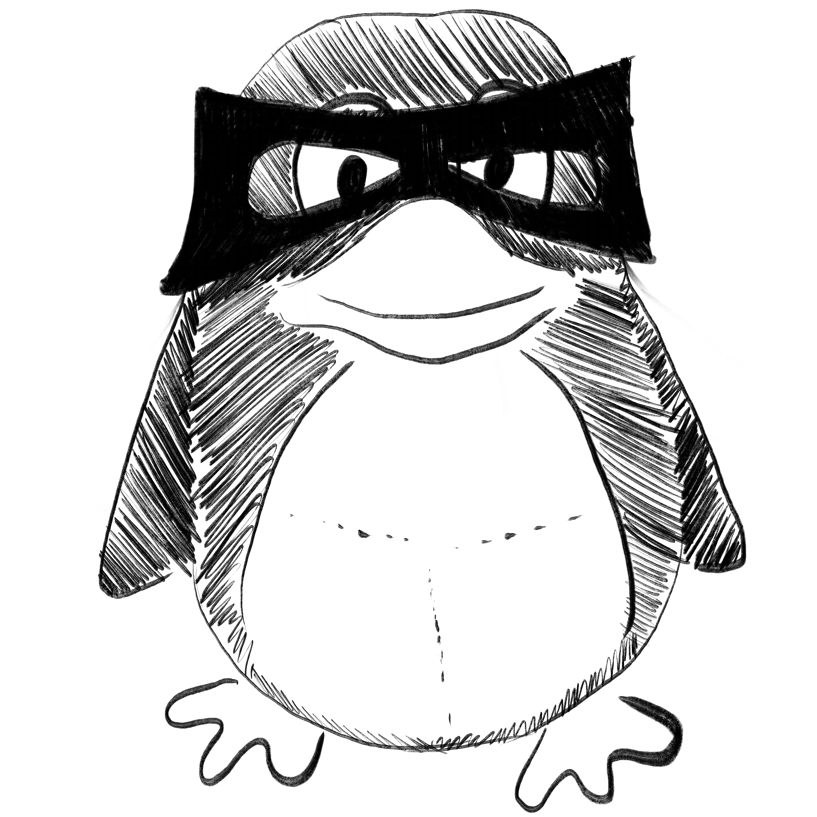
The effect of mechanical feedback on outcome in self-care support tool based on solution-focused brief therapy.
In Psychotherapy research : journal of the Society for Psychotherapy Research
Takagi Gen
2023-Mar-13
goal, machine learning, mechanical feedback, natural language processing, self-care support tool, solution-focused brief therapy
Radiomics and machine learning applied to STIR sequence for prediction of quantitative parameters in facioscapulohumeral disease.
In Frontiers in neurology
PURPOSE :
METHODS :
RESULTS :
CONCLUSION :
Colelli Giulia, Barzaghi Leonardo, Paoletti Matteo, Monforte Mauro, Bergsland Niels, Manco Giulia, Deligianni Xeni, Santini Francesco, Ricci Enzo, Tasca Giorgio, Mira Antonietta, Figini Silvia, Pichiecchio Anna
2023
FSHD, machine learning, muscle MRI, radiomics, stir
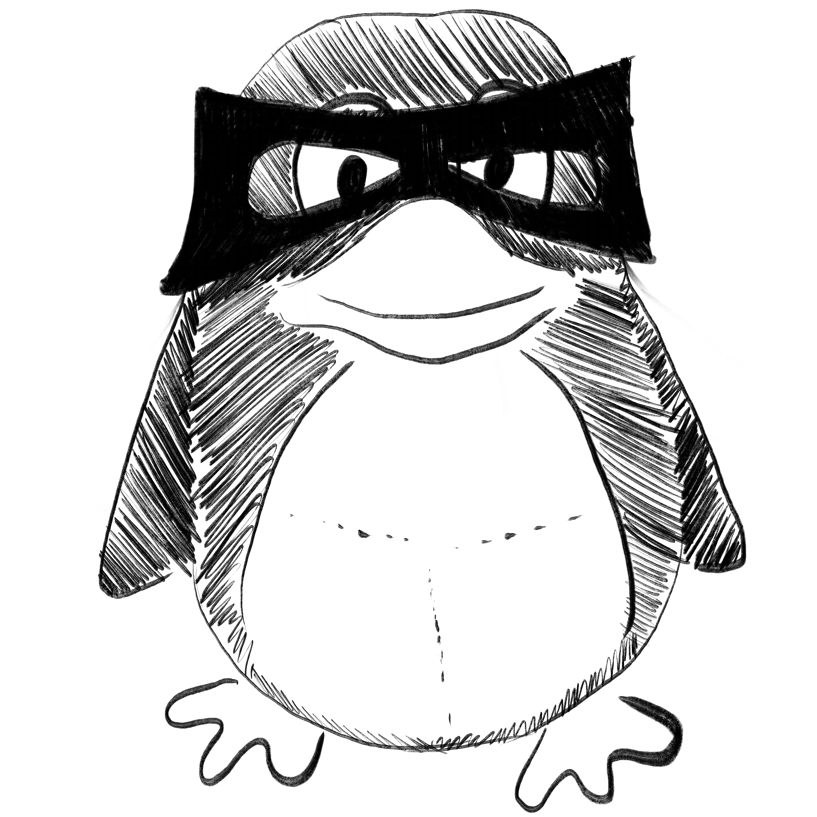
Toward Causal Understanding of Therapist-Client Relationships: A Study of Language Modality and Social Entrainment.
In Proceedings of the ... ACM International Conference on Multimodal Interaction. ICMI (Conference)
Vail Alexandria K, Girard Jeffrey M, Bylsma Lauren M, Cohn Jeffrey F, Fournier Jay, Swartz Holly A, Morency Louis-Philippe
2022-Nov
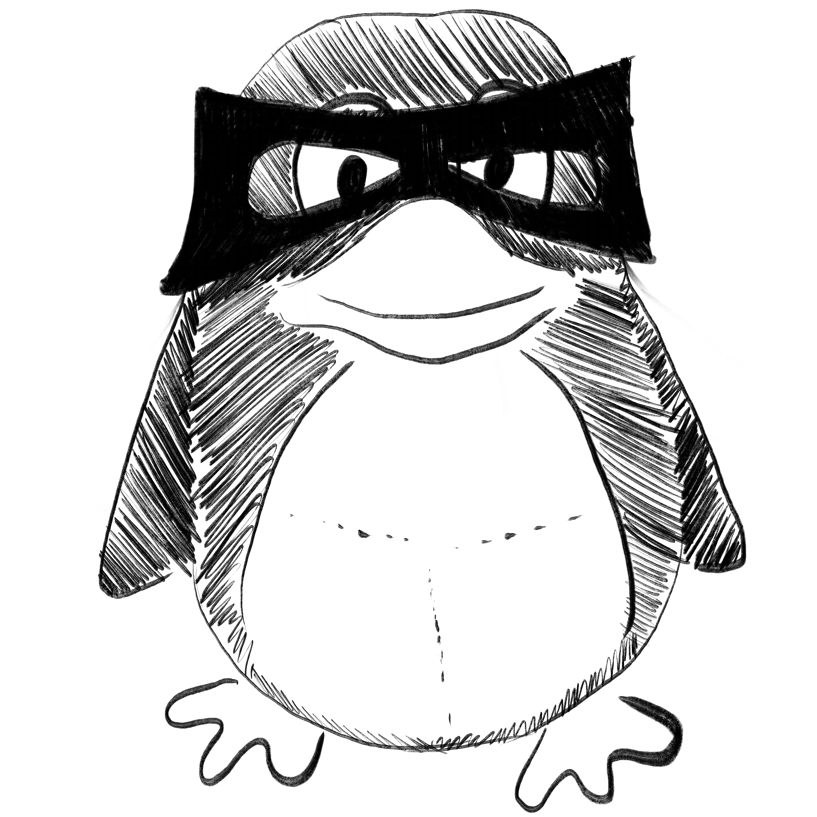
Minimizing Viral Transmission in COVID-19 Like Pandemics: Technologies, Challenges, and Opportunities.
In IEEE sensors journal
Nisar Shibli, Wakeel Abdul, Tahir Wania, Tariq Muhammad
2023-Jan
COVID-19, artificial intelligence, cellular forensics, hidden patterns, pandemic, tracking systems
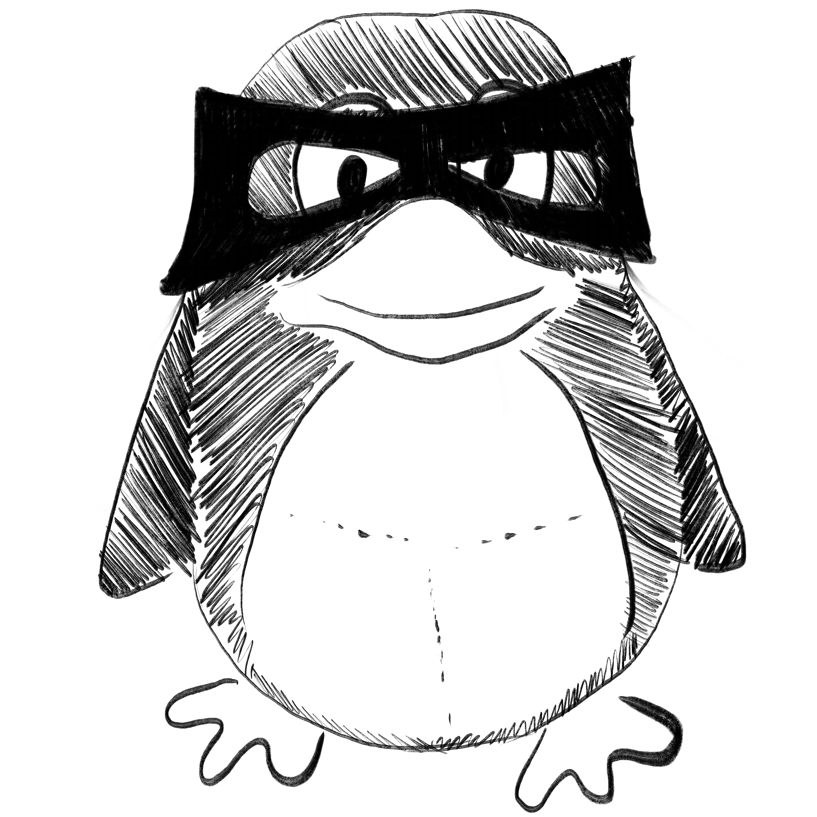
Situation-Aware BDI Reasoning to Detect Early Symptoms of Covid 19 Using Smartwatch.
In IEEE sensors journal
Saleem Kiran, Saleem Misbah, Ahmad Rana Zeeshan, Javed Abdul Rehman, Alazab Mamoun, Gadekallu Thippa Reddy, Suleman Ahmad
2023-Jan
Covid-19, NetLogo, Situation-awareness, ambient intelligence, belief-desire-intention (BDI), healthcare
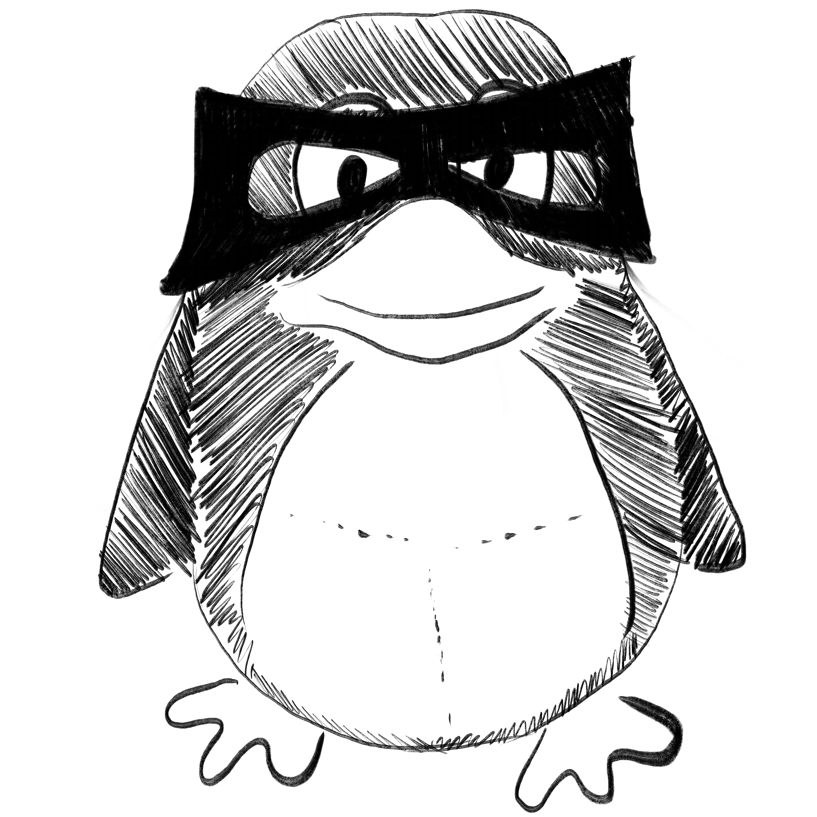
i-Sheet: A Low-Cost Bedsheet Sensor for Remote Diagnosis of Isolated Individuals.
In IEEE sensors journal
Tapwal Riya, Misra Sudip, Deb Pallav Kumar
2023-Jan
Artificial intelligence, COVID-19, remote monitoring, sensors, smart bedsheet
Weekly Summary
Receive a weekly summary and discussion of the top papers of the week by leading researchers in the field.