Receive a weekly summary and discussion of the top papers of the week by leading researchers in the field.
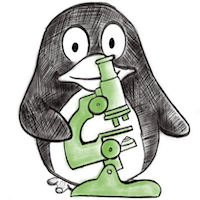
Clinically Relevant Latent Space Embedding of Cancer Histopathology Slides through Variational Autoencoder Based Image Compression
ArXiv Preprint
Mohammad Sadegh Nasr, Amir Hajighasemi, Paul Koomey, Parisa Boodaghi Malidarreh, Michael Robben, Jillur Rahman Saurav, Helen H. Shang, Manfred Huber, Jacob M. Luber
2023-03-23
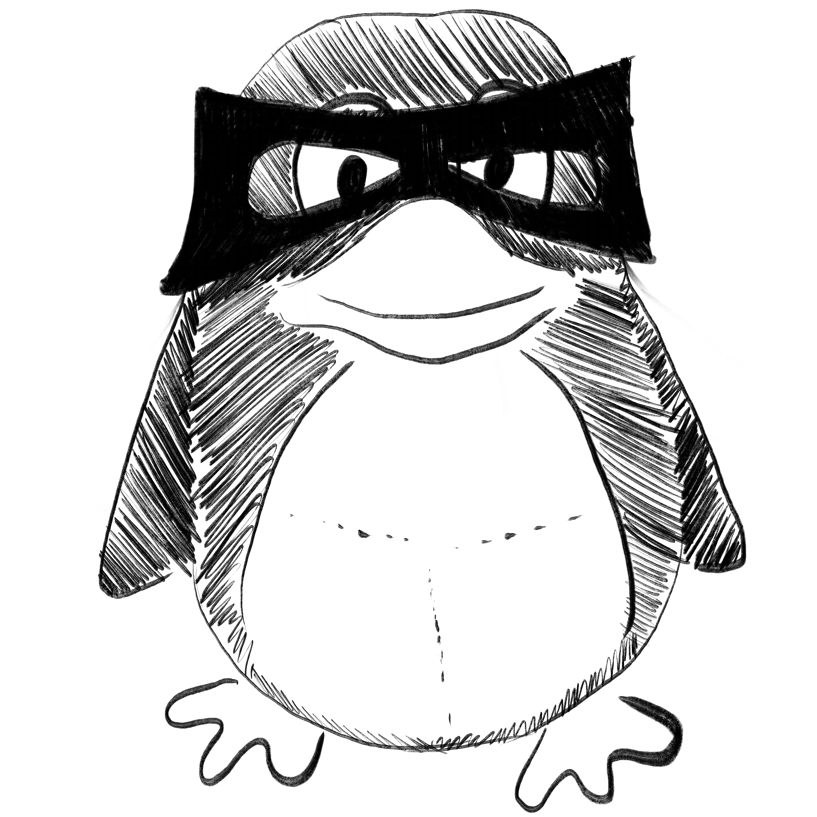
SARS-CoV-2 related adaptation mechanisms of rehabilitation clinics affecting patient-centred care: Qualitative study of online patient reports.
In JMIR rehabilitation and assistive technologies
BACKGROUND :
OBJECTIVE :
METHODS :
RESULTS :
CONCLUSIONS :
CLINICALTRIAL :
Kühn Lukas, Lindert Lara, Kuper Paulina, Choi Kyung-Eun Anna
2023-Mar-05
Predictors of Cyberchondria during the COVID-19 pandemic: A cross-sectional study using supervised machine learning.
In JMIR formative research
BACKGROUND :
OBJECTIVE :
METHODS :
RESULTS :
CONCLUSIONS :
Infanti Alexandre, Starcevic Vladan, Schimmenti Adriano, Khazaal Yasser, Karila Laurent, Giardina Alessandro, Flayelle Maèva, Hedayatzadeh Razavi Seyedeh Boshra, Baggio Stéphanie, Vögele Claus, Billieux Joël
2023-Mar-09
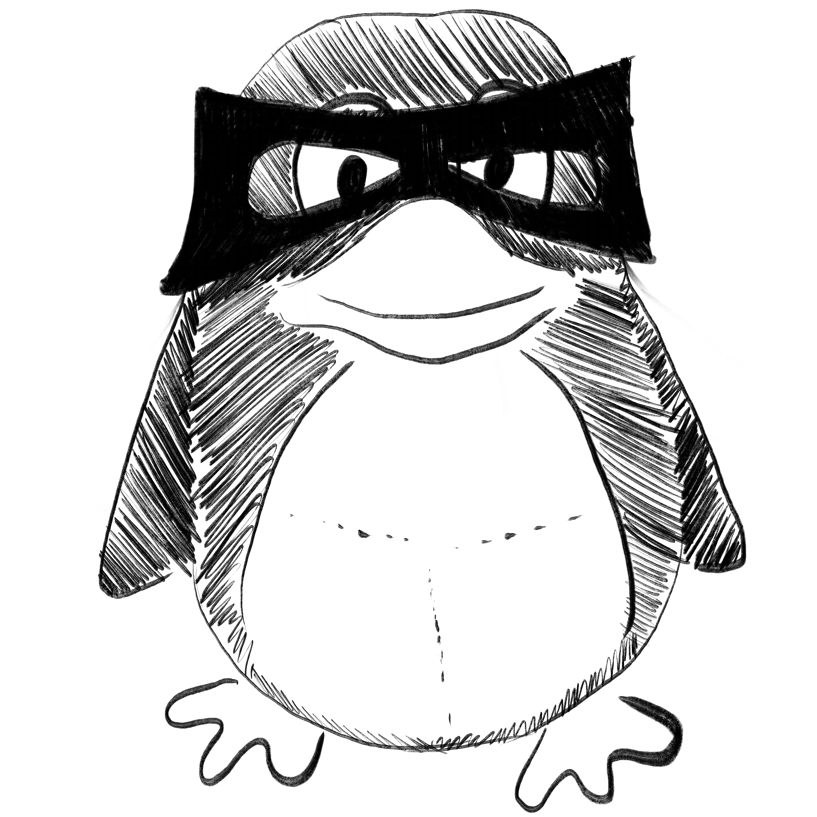
The hair cell analysis toolbox is a precise and fully automated pipeline for whole cochlea hair cell quantification.
In PLoS biology
Buswinka Christopher J, Osgood Richard T, Simikyan Rubina G, Rosenberg David B, Indzhykulian Artur A
2023-Mar-22
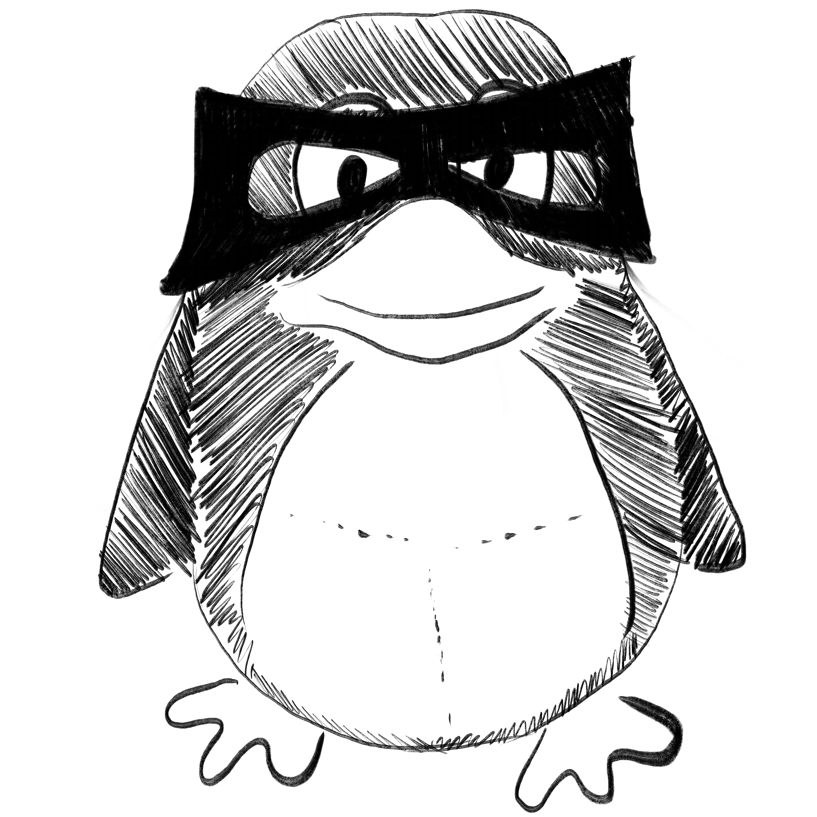
Characterization of RNA polymerase II trigger loop mutations using molecular dynamics simulations and machine learning.
In PLoS computational biology
Dutagaci Bercem, Duan Bingbing, Qiu Chenxi, Kaplan Craig D, Feig Michael
2023-Mar-22
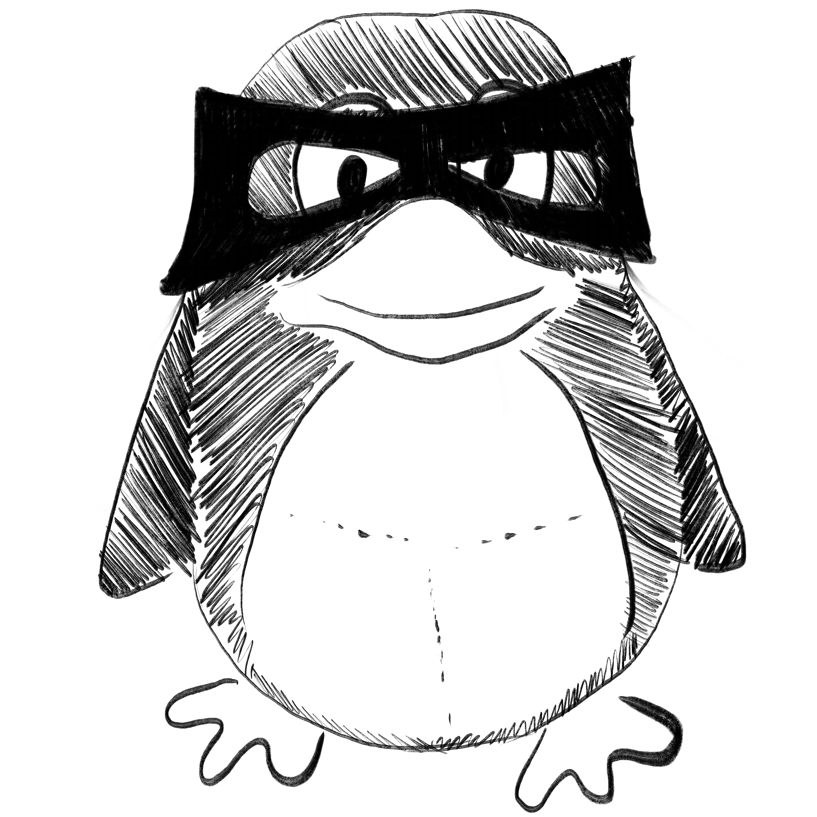
Classification of truck-involved crash severity: Dealing with missing, imbalanced, and high dimensional safety data.
In PloS one ; h5-index 176.0
Mohammadpour Seyed Iman, Khedmati Majid, Zada Mohammad Javad Hassan
2023
Weekly Summary
Receive a weekly summary and discussion of the top papers of the week by leading researchers in the field.