Receive a weekly summary and discussion of the top papers of the week by leading researchers in the field.
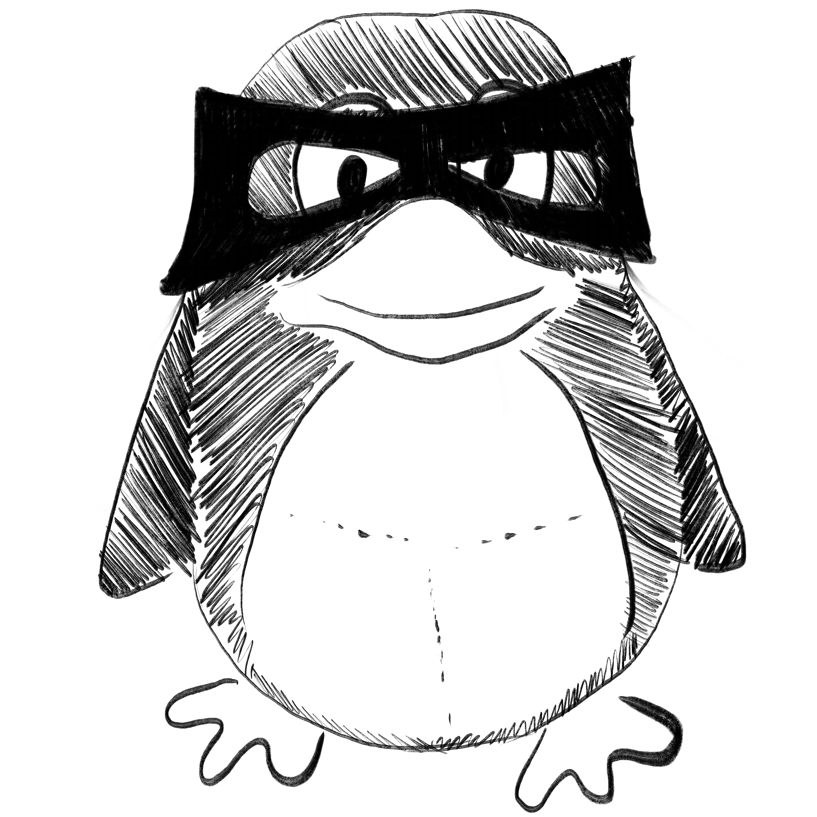
ARTIFICIAL INTELLIGENCE MODELS SHOW POTENTIAL IN RECOGNIZING THE DENTAL IMPLANT TYPE, PREDICTING IMPLANT SUCCESS, AND OPTIMIZING IMPLANT DESIGN.
In The journal of evidence-based dental practice
ARTICLE TITLE AND BIBLIOGRAPHIC INFORMATION :
SOURCE OF FUNDING :
TYPE OF STUDY/DESIGN :
Alqutaibi Ahmed Yaseen
2023-Mar
AI, Artificial intelligence, Dental implant, Implant design, Implant success, Machine learning
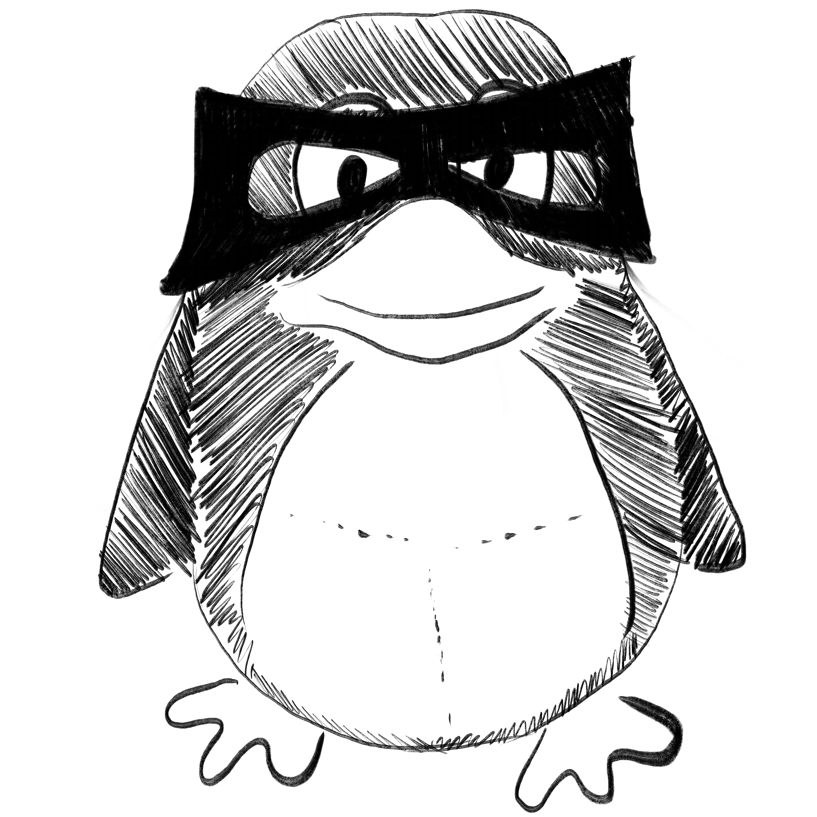
Risk evaluation of carbapenem-induced liver injury based on machine learning analysis.
In Journal of infection and chemotherapy : official journal of the Japan Society of Chemotherapy
INTRODUCTION :
METHODS :
RESULTS :
CONCLUSIONS :
Asai Yuki, Ooi Hayahide, Sato Yoshiharu
2023-Mar-11
Carbapenem, Decision tree analysis, Doripenem, Flowchart, Machine learning, Meropenem
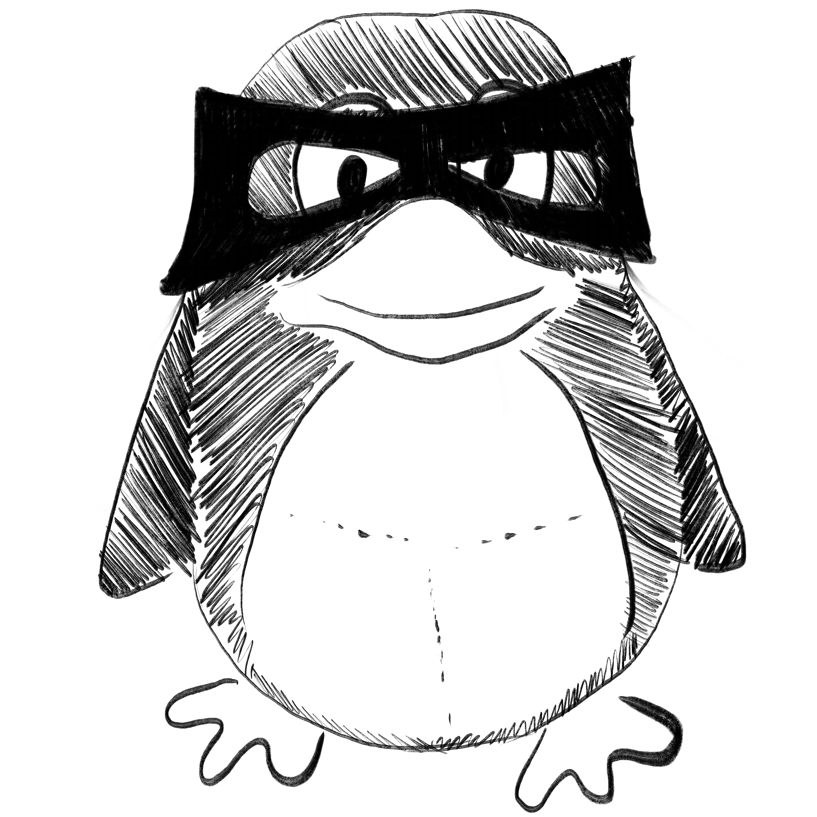
External Validation of an Extreme Gradient Boosting Model for Prediction of Delayed Cerebral Ischemia after Aneurysmal Subarachnoid Hemorrhage.
In World neurosurgery ; h5-index 47.0
BACKGROUND :
METHODS :
RESULTS :
CONCLUSION :
Palmisciano Paolo, Hoz Samer S, Johnson Mark D, Forbes Jonathan A, Prestigiacomo Charles J, Zuccarello Mario, Andaluz Norberto
2023-Mar-11
Aneurysmal subarachnoid hemorrhage, Delayed cerebral ischemia, Machine learning, Predictive Analysis, Vasospasm
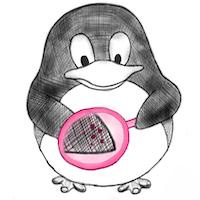
The predictors of death within 1 year in acute ischemic stroke patients based on machine learning.
In Frontiers in neurology
OBJECTIVE :
METHODS :
RESULTS :
CONCLUSIONS :
Wang Kai, Gu Longyuan, Liu Wencai, Xu Chan, Yin Chengliang, Liu Haiyan, Rong Liangqun, Li Wenle, Wei Xiu’e
2023
biomarkers, ischemic stroke, machine learning, prediction model, web calculator
Quantitative Morphometry and Machine Learning Model to Explore Duodenal and Rectal Mucosal Tissue of Children with Environmental Enteric Dysfunction.
In The American journal of tropical medicine and hygiene
Khan Marium, Jamil Zehra, Ehsan Lubaina, Zulqarnain Fatima, Srivastava Sanjana, Siddiqui Saman, Fernandes Philip, Raghib Muhammad, Sengupta Saurav, Mujahid Zia, Ahmed Zubair, Idrees Romana, Ahmed Sheraz, Umrani Fayaz, Iqbal Najeeha, Moskaluk Christopher, Raghavan Shyam, Cheng Lin, Moore Sean, Ali Syed Asad, Iqbal Junaid, Syed Sana
2023-Mar-13
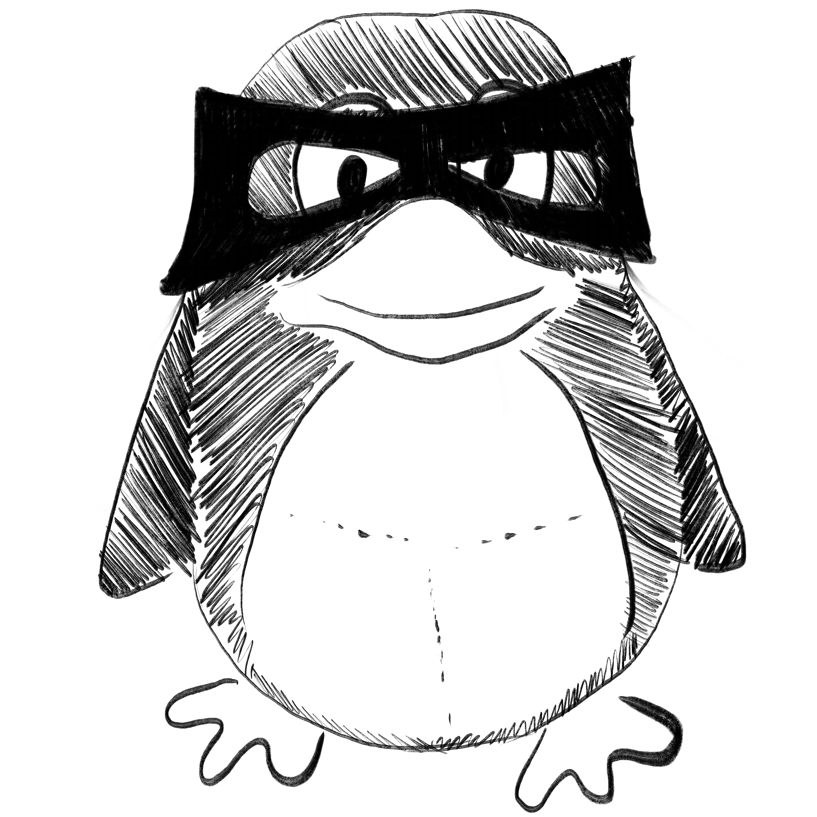
Automatic segmentation of the choroid plexuses: Method and validation in controls and patients with multiple sclerosis.
In NeuroImage. Clinical
Yazdan-Panah Arya, Schmidt-Mengin Marius, Ricigliano Vito A G, Soulier Théodore, Stankoff Bruno, Colliot Olivier
2023-Mar-06
Choroid plexus, Deep learning, Multiple sclerosis, Radiologically isolated syndrome, Segmentation
Weekly Summary
Receive a weekly summary and discussion of the top papers of the week by leading researchers in the field.