Receive a weekly summary and discussion of the top papers of the week by leading researchers in the field.
Depression, cardiometabolic disease, and their co-occurrence after childhood maltreatment: an individual participant data meta-analysis including over 200,000 participants.
In BMC medicine ; h5-index 89.0
BACKGROUND :
METHODS :
RESULTS :
CONCLUSIONS :
Souama Camille, Lamers Femke, Milaneschi Yuri, Vinkers Christiaan H, Defina Serena, Garvert Linda, Stein Frederike, Woofenden Tom, Brosch Katharina, Dannlowski Udo, Galenkamp Henrike, de Graaf Ron, Jaddoe Vincent W V, Lok Anja, van Rijn Bas B, Völzke Henry, Cecil Charlotte A M, Felix Janine F, Grabe Hans J, Kircher Tilo, Lekadir Karim, Have Margreet Ten, Walton Esther, Penninx Brenda W J H
2023-Mar-13
Adverse childhood experiences, Cardiovascular diseases, Child abuse, Childhood maltreatment, Comorbidity, Depression, Depressive disorder, Diabetes mellitus, Meta-analysis
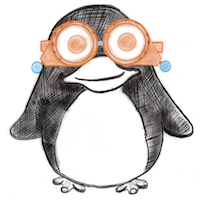
Implementation of deep learning artificial intelligence in vision-threatening disease screenings for an underserved community during COVID-19.
In Journal of telemedicine and telecare ; h5-index 28.0
INTRODUCTION :
METHODS :
RESULTS :
DISCUSSION :
Zhu Aretha, Tailor Priya, Verma Rashika, Zhang Isis, Schott Brian, Ye Catherine, Szirth Bernard, Habiel Miriam, Khouri Albert S
2023-Mar-13
COVID-19, Tele-ophthalmology, deep learning artificial intelligence, vision screenings
Medical Phrase Grounding with Region-Phrase Context Contrastive Alignment
ArXiv Preprint
Zhihao Chen, Yang Zhou, Anh Tran, Junting Zhao, Liang Wan, Gideon Ooi, Lionel Cheng, Choon Hua Thng, Xinxing Xu, Yong Liu, Huazhu Fu
2023-03-14
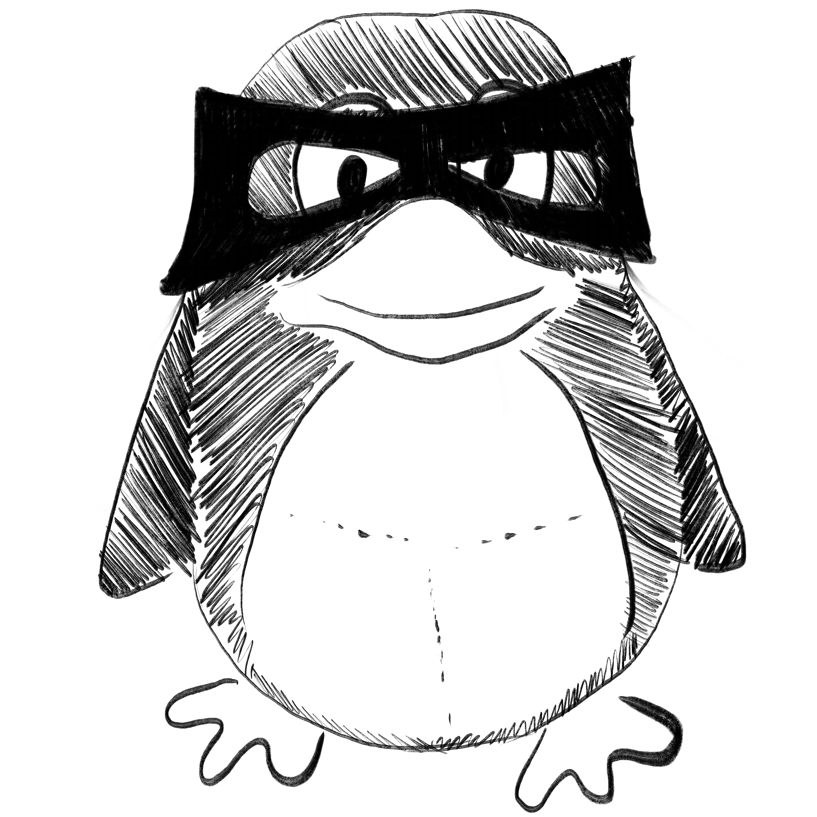
Heating Up: How Early Twitter Marketing Gave Rise to Organic Word-of-Mouth About Heated Tobacco Products.
In Social media + society
Kostygina Ganna, Tran Hy, Kim Yoonsang, Czaplicki Lauren, Kierstead Elexis, Kreslake Jennifer, Emery Sherry, Schillo Barbara
2022
advertising and promotion, social media
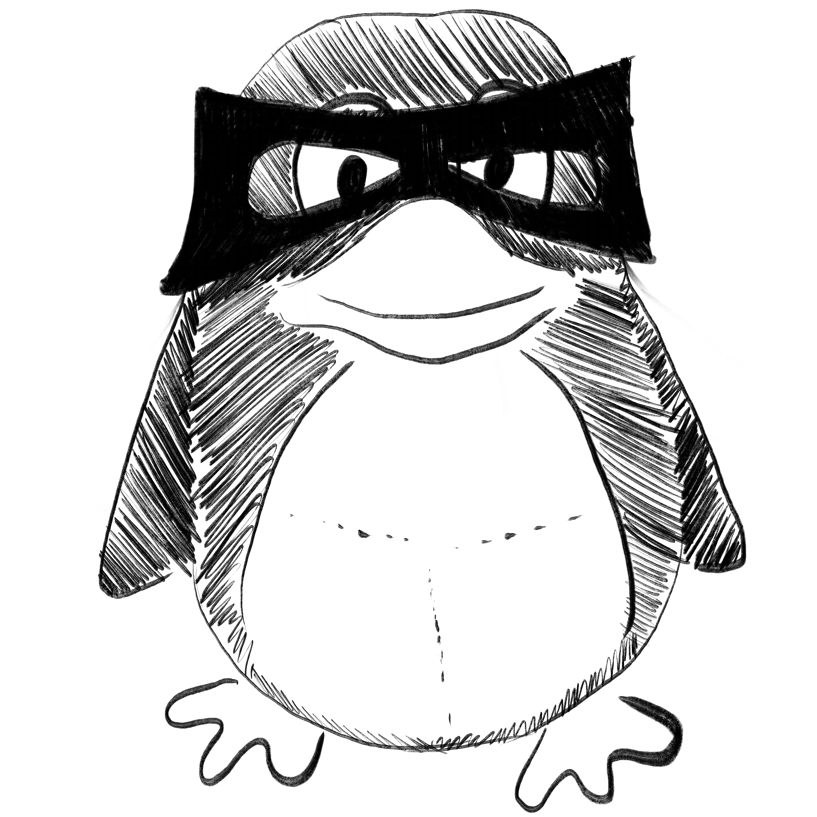
Cognitive rehabilitation in multiple sclerosis: Three digital ingredients to address current and future priorities.
In Frontiers in human neuroscience ; h5-index 79.0
Tacchino Andrea, Podda Jessica, Bergamaschi Valeria, Pedullà Ludovico, Brichetto Giampaolo
2023
cognitive deficit, cognitive disorder, cognitive impairment, digital twin, dual-task, metaverse, multiple sclerosis, telerehabilitation
IPs-GRUAtt: An attention-based bidirectional gated recurrent unit network for predicting phosphorylation sites of SARS-CoV-2 infection.
In Molecular therapy. Nucleic acids
Zhang Guiyang, Tang Qiang, Feng Pengmian, Chen Wei
2023-Jun-13
MT: Bioinformatics, SARS-CoV-2, attention mechanism, bidirectional gated recurrent unit, deep learning, interpretation, phosphorylation
Weekly Summary
Receive a weekly summary and discussion of the top papers of the week by leading researchers in the field.