Receive a weekly summary and discussion of the top papers of the week by leading researchers in the field.
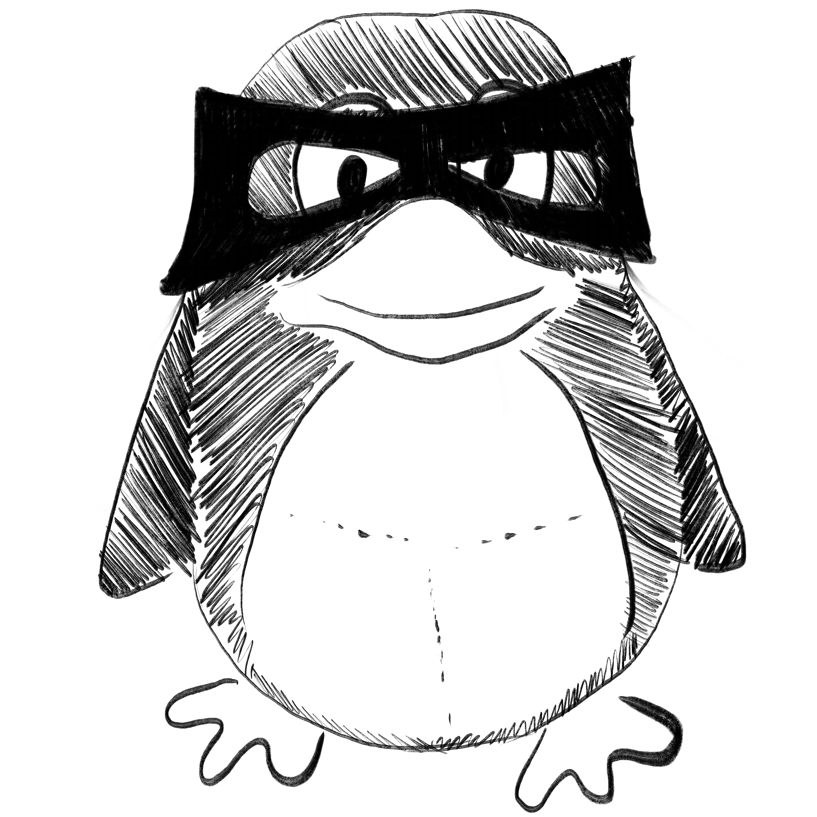
Ascertaining the optimal myoelectric signal recording duration for pattern recognition based prostheses control.
In Frontiers in neuroscience ; h5-index 72.0
INTRODUCTION :
METHOD :
RESULT :
Asogbon Mojisola Grace, Samuel Oluwarotimi Williams, Nsugbe Ejay, Li Yongcheng, Kulwa Frank, Mzurikwao Deogratias, Chen Shixiong, Li Guanglin
2023
electromyogram (EMG), finger gestures, pattern recognition (PR), prostheses, signal recording duration (SRD)
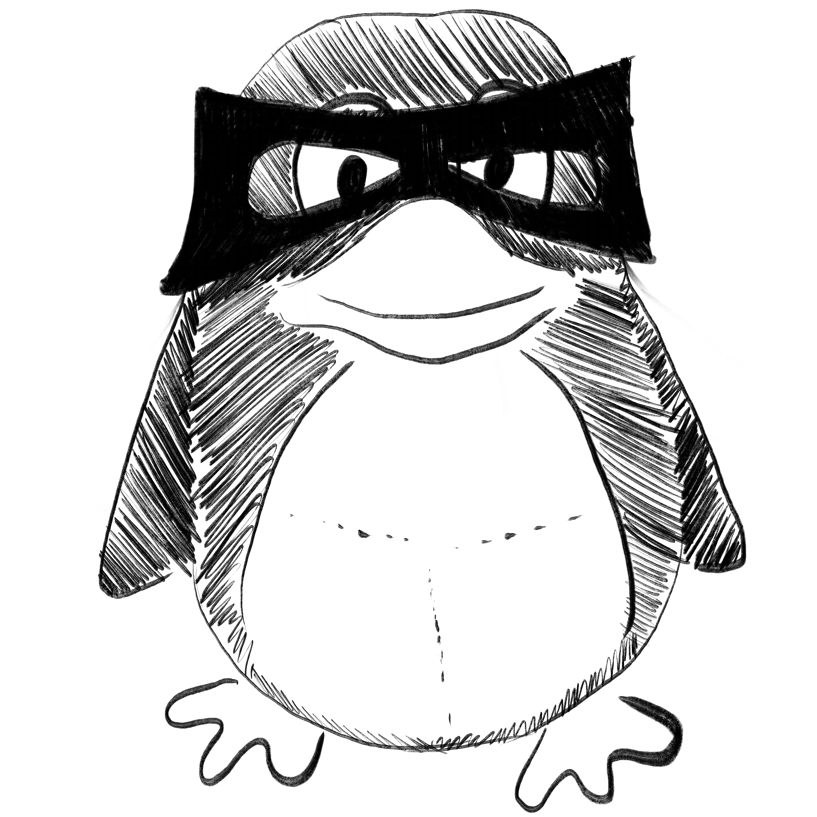
Explainable machine learning for long-term outcome prediction in two-center stroke patients after intravenous thrombolysis.
In Frontiers in neuroscience ; h5-index 72.0
OBJECTIVE :
METHODS :
RESULTS :
CONCLUSION :
Ping Zheng, Huiyu She, Min Li, Qingke Bai, Qiuyun Lu, Xu Chen
2023
NIHSS, cerebral infarction, intravenous thrombolysis, ischemic stroke, machine learning, modified rankin score
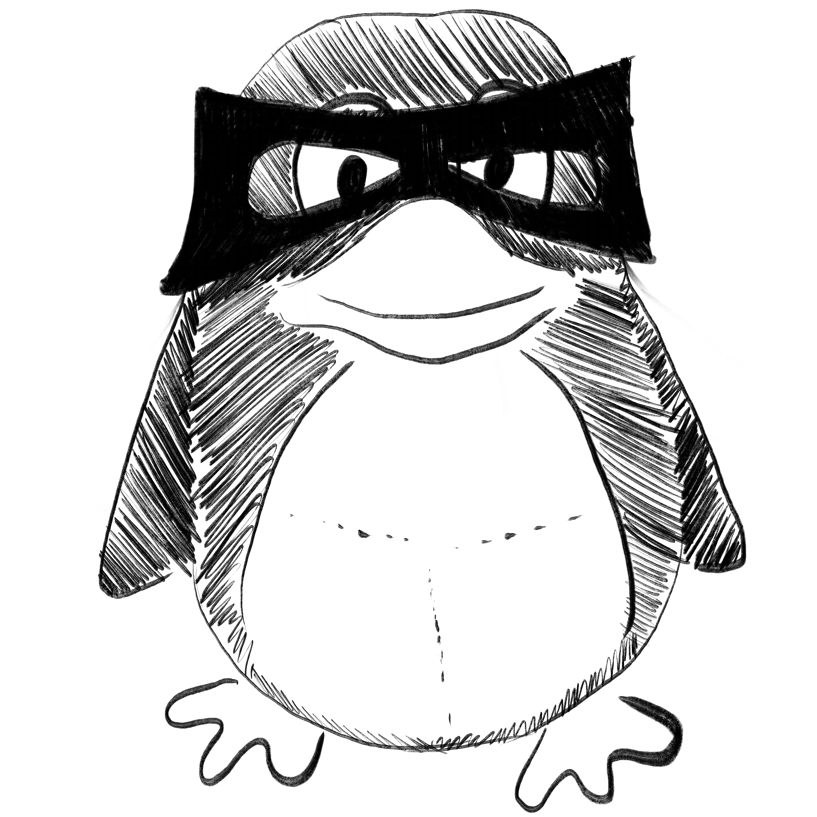
Recognition of single upper limb motor imagery tasks from EEG using multi-branch fusion convolutional neural network.
In Frontiers in neuroscience ; h5-index 72.0
Zhang Rui, Chen Yadi, Xu Zongxin, Zhang Lipeng, Hu Yuxia, Chen Mingming
2023
brain-computer interface (BCI), convolutional neural network (CNN), deep learning, feature fusion, single upper limb motor imagery
Population level multimodal neuroimaging correlates of attention-deficit hyperactivity disorder among children.
In Frontiers in neuroscience ; h5-index 72.0
OBJECTIVES :
METHODS :
RESULTS :
CONCLUSION :
Lin Huang, Haider Stefan P, Kaltenhauser Simone, Mozayan Ali, Malhotra Ajay, Constable R Todd, Scheinost Dustin, Ment Laura R, Konrad Kerstin, Payabvash Seyedmehdi
2023
attention-deficient hyperactivity disorder, brain connectivity, cortex morphology, machine learning, white matter microstructure
Ischemic stroke prediction of patients with carotid atherosclerotic stenosis via multi-modality fused network.
In Frontiers in neuroscience ; h5-index 72.0
Lv Peng, Yang Jing, Wang Jiacheng, Guo Yi, Tang Qiying, Magnier Baptiste, Lin Jiang, Zhou Jianjun
2023
brain MRI, carotid atherosclerotic stenosis, deep learning, diagnostic classification, ischemic stroke
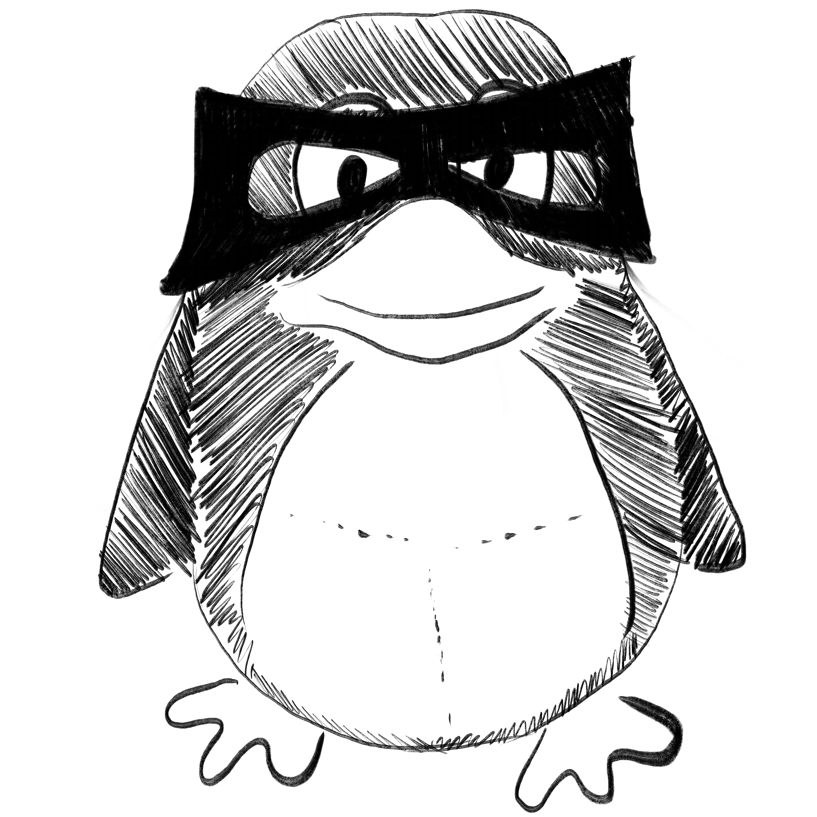
Kohonen neural network and symbiotic-organism search algorithm for intrusion detection of network viruses.
In Frontiers in computational neuroscience
INTRODUCTION :
METHODS :
RESULTS :
DISCUSSION :
Zhou Guo, Miao Fahui, Tang Zhonghua, Zhou Yongquan, Luo Qifang
2023
Kohonen neural network, detection rate, false alarm rate, intrusion detection, symbiotic-organism search algorithm
Weekly Summary
Receive a weekly summary and discussion of the top papers of the week by leading researchers in the field.