Receive a weekly summary and discussion of the top papers of the week by leading researchers in the field.
IPs-GRUAtt: An attention-based bidirectional gated recurrent unit network for predicting phosphorylation sites of SARS-CoV-2 infection.
In Molecular therapy. Nucleic acids
Zhang Guiyang, Tang Qiang, Feng Pengmian, Chen Wei
2023-Jun-13
MT: Bioinformatics, SARS-CoV-2, attention mechanism, bidirectional gated recurrent unit, deep learning, interpretation, phosphorylation
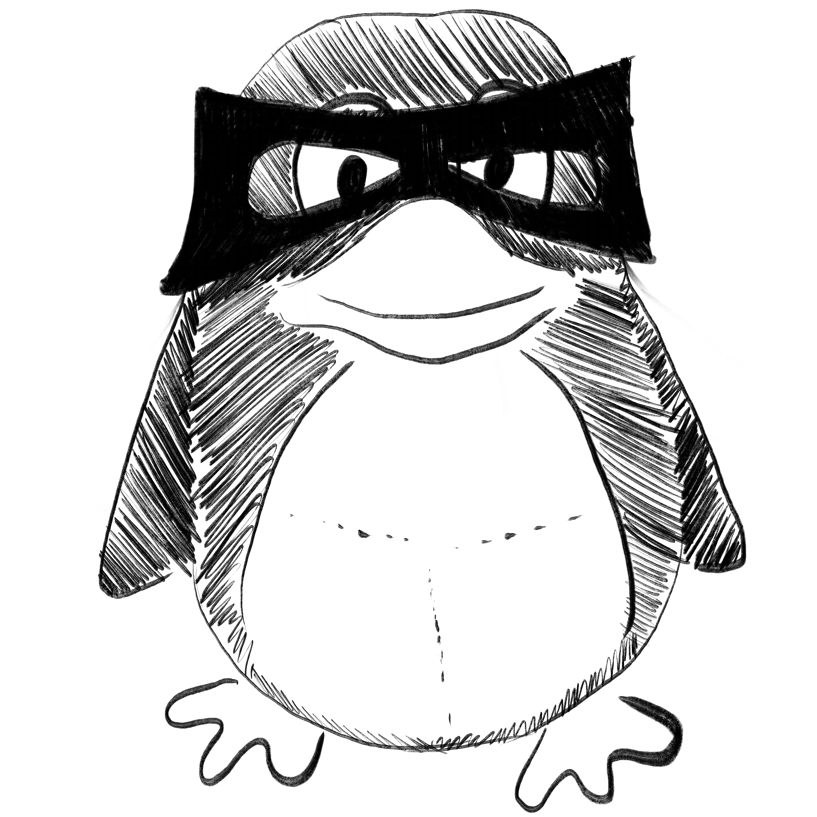
Tensor-based Multimodal Learning for Prediction of Pulmonary Arterial Wedge Pressure from Cardiac MRI
ArXiv Preprint
Prasun C. Tripathi, Mohammod N. I. Suvon, Lawrence Schobs, Shuo Zhou, Samer Alabed, Andrew J. Swift, Haiping Lu
2023-03-14
Preoperative Prediction and Identification of Extracapsular Extension in Head and Neck Cancer Patients: Progress and Potential.
In Cureus
Duggar William N, Vengaloor Thomas Toms, Wang Yibin, Rahman Abdur, Wang Haifeng, Roberts Paul R, Bian Linkan, Gatewood Ronald T, Vijayakumar Srinivasan
2023-Feb
artificial intelligence, deep learning, extracapsular extension, head and neck squamous cell carcinoma, model explainability, preoperative
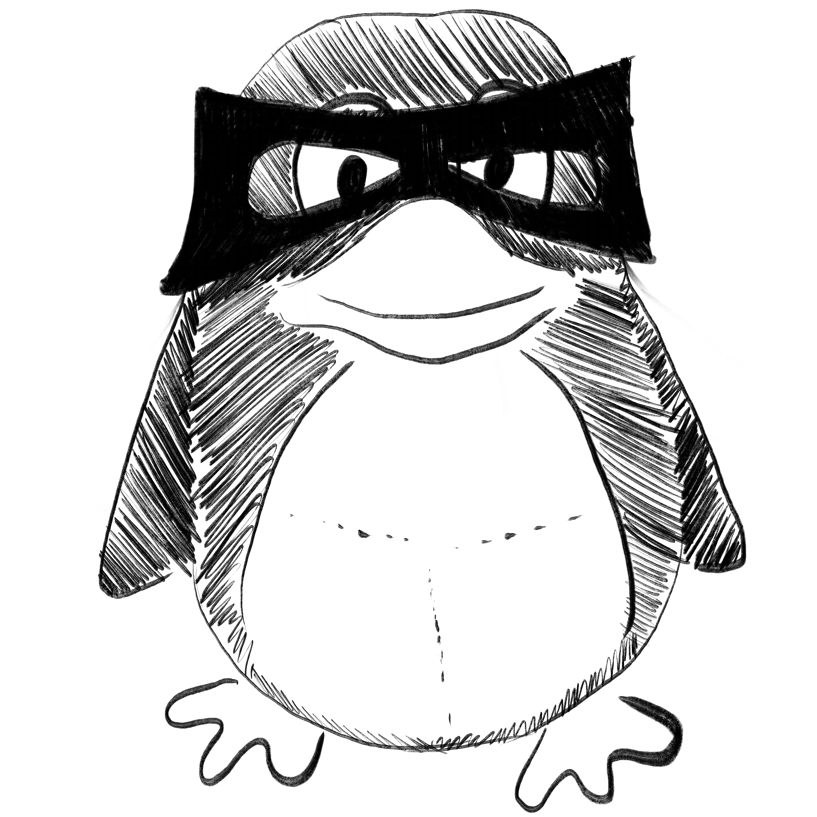
Distributed Caregiving for Cognitively Impaired Individuals: A Case Report.
In Cureus
Alemi Yara, Loughman Blaise, Uriyo Maria
2023-Feb
care navigation, caregiver burden, case study, cognitive impairment, home platforms
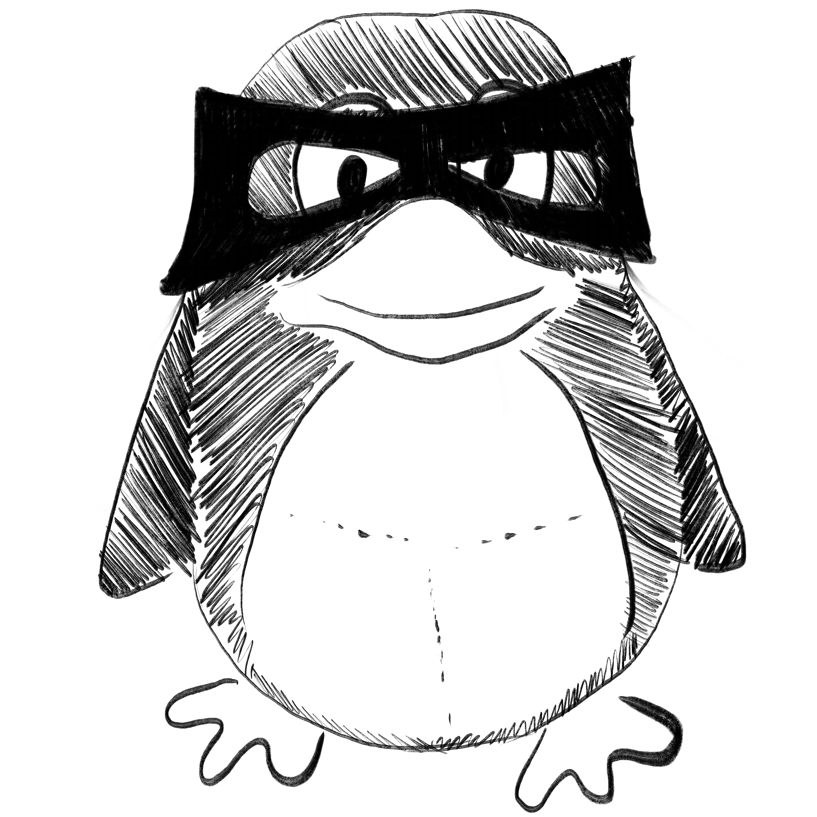
Sprinting performance of individuals with unilateral transfemoral amputation: compensation strategies for lower limb coordination.
In Royal Society open science
Hu Mingyu, Kobayashi Toshiki, Hisano Genki, Murata Hiroto, Ichimura Daisuke, Hobara Hiroaki
2023-Mar
continuous relative phase, gait, prosthetic, run, running-specific prosthesis
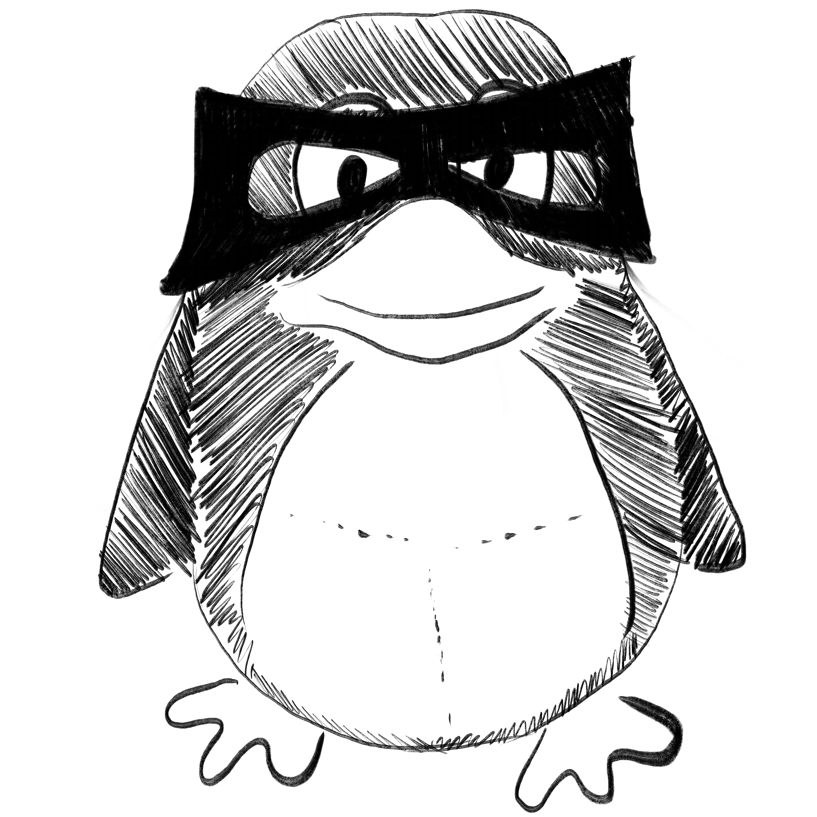
Did AI get more negative recently?
In Royal Society open science
Beese Dominik, Altunbaş Begüm, Güzeler Görkem, Eger Steffen
2023-Mar
SciBERT, citation sentiment, natural language processing, negativity, science-of-science, trend prediction
Weekly Summary
Receive a weekly summary and discussion of the top papers of the week by leading researchers in the field.