Receive a weekly summary and discussion of the top papers of the week by leading researchers in the field.
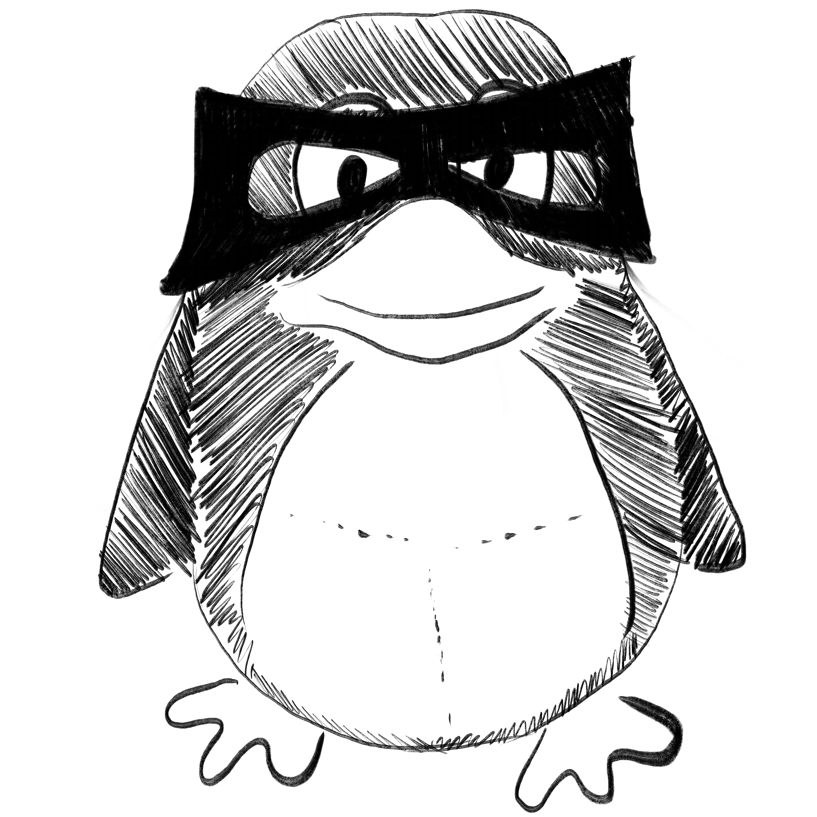
Automated extraction of pod phenotype data from micro-computed tomography.
In Frontiers in plant science
INTRODUCTION :
METHODS :
RESULTS :
DISCUSSION :
Corcoran Evangeline, Siles Laura, Kurup Smita, Ahnert Sebastian
2023
computer vision, machine learning, micro-compute tomography, phenotyping, plant development
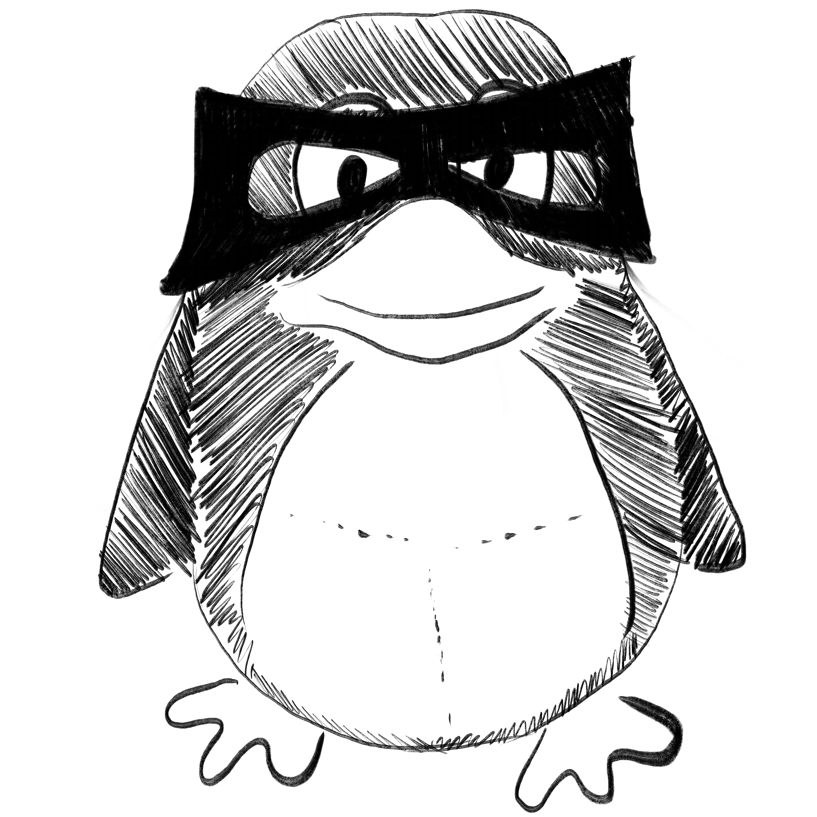
Fungal identification in peanuts seeds through multispectral images: Technological advances to enhance sanitary quality.
In Frontiers in plant science
Sudki Julia Marconato, Fonseca de Oliveira Gustavo Roberto, de Medeiros André Dantas, Mastrangelo Thiago, Arthur Valter, Amaral da Silva Edvaldo Aparecido, Mastrangelo Clíssia Barboza
2023
Arachis hypogaea L., Aspergillus spp., machine learning, seed health, support vector machine
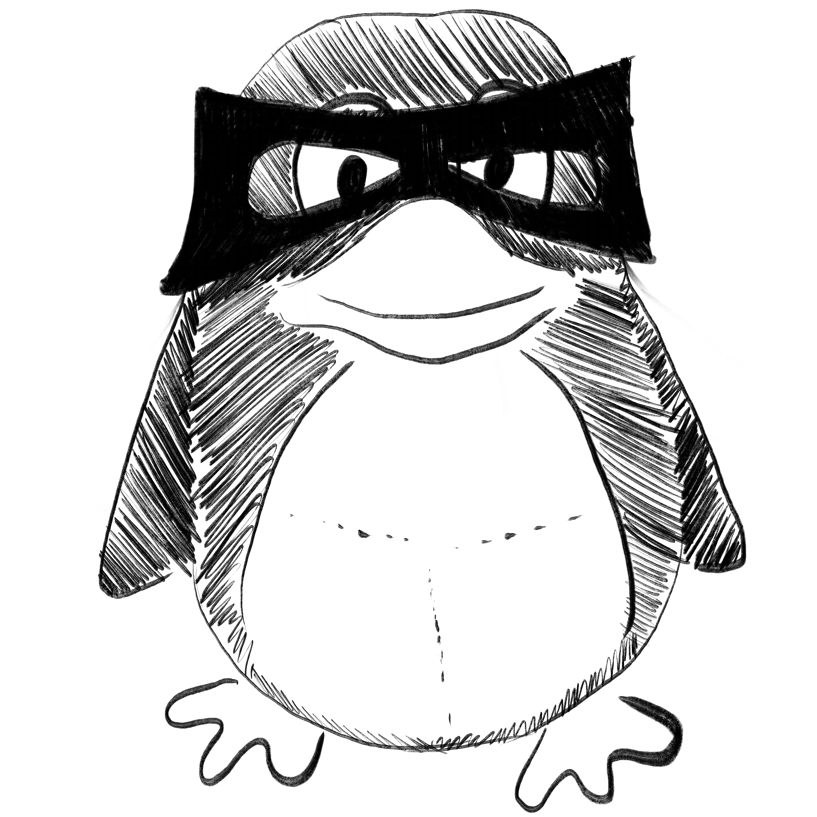
Data Flush.
In Harvard data science review
Shen Xiaotong, Bi Xuan, Shen Rex
2022
Census, data integration, differential privacy, distribution preservation, statistical inference
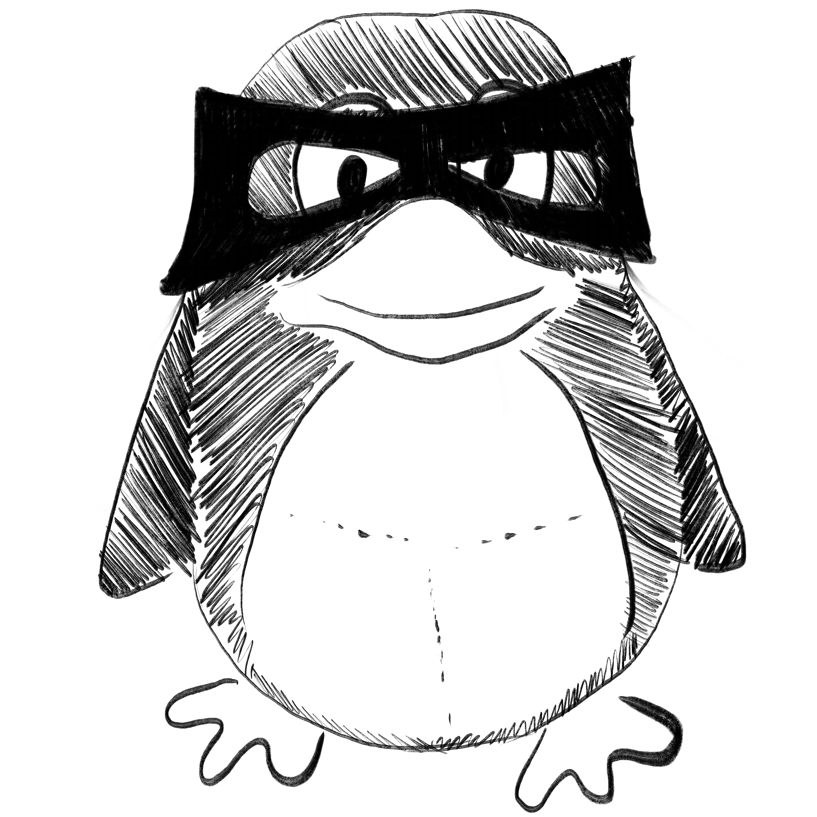
Leveraging explanations in interactive machine learning: An overview.
In Frontiers in artificial intelligence
Teso Stefano, Alkan Öznur, Stammer Wolfgang, Daly Elizabeth
2023
explainable AI, human-in-the-loop, interactive machine learning, model debugging, model editing
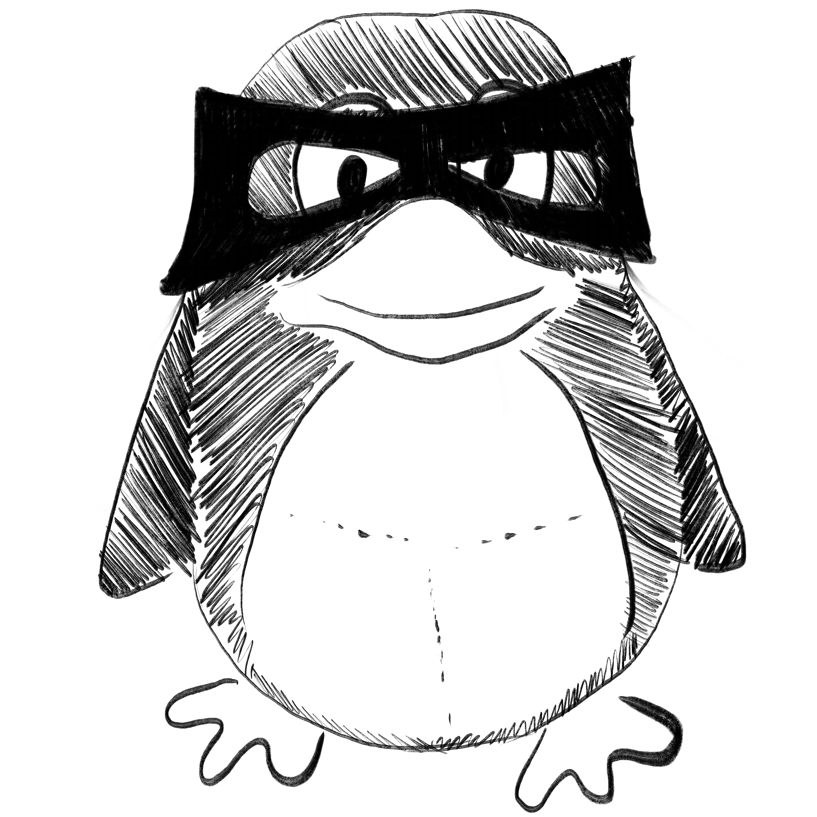
Optimal blending of multiple independent prediction models.
In Frontiers in artificial intelligence
Taraba Peter
2023
Gaussians, blending of independent models, going wider, machine learning with counts, normal distributions
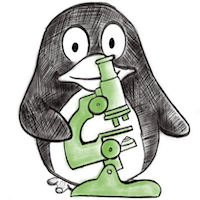
SMPD1 expression profile and mutation landscape help decipher genotype-phenotype association and precision diagnosis for acid sphingomyelinase deficiency.
In Hereditas
BACKGROUND :
RESULTS :
CONCLUSIONS :
Wang Ruisong, Qin Ziyi, Huang Long, Luo Huiling, Peng Han, Zhou Xinyu, Zhao Zhixiang, Liu Mingyao, Yang Pinhong, Shi Tieliu
2023-Mar-13
Acid sphingomyelinase deficiency, Genotype, Niemann-pick disease type a and B, Novel target for the subtypes, Phenotype
Weekly Summary
Receive a weekly summary and discussion of the top papers of the week by leading researchers in the field.