Receive a weekly summary and discussion of the top papers of the week by leading researchers in the field.
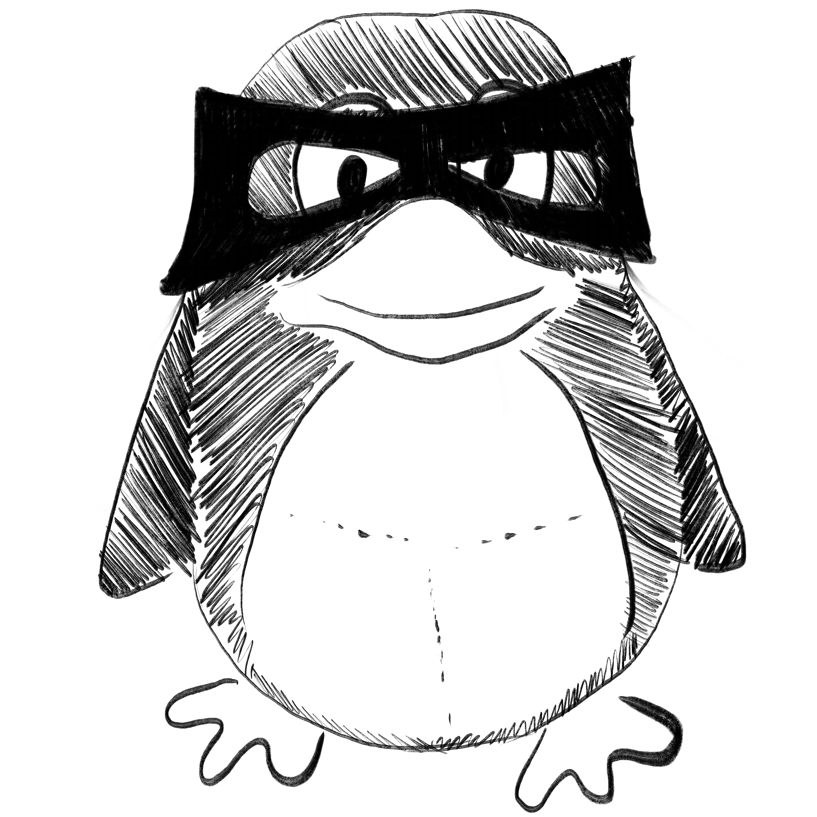
A data-efficient deep learning tool for scRNA-Seq label transfer in neuroscience.
In bioRxiv : the preprint server for biology
Lehrer Julian, Gonzalez-Ferrer Jesus, Haussler David, Teodorescu Mircea, Jonsson Vanessa D, Mostajo-Radji Mohammed A
2023-Mar-01
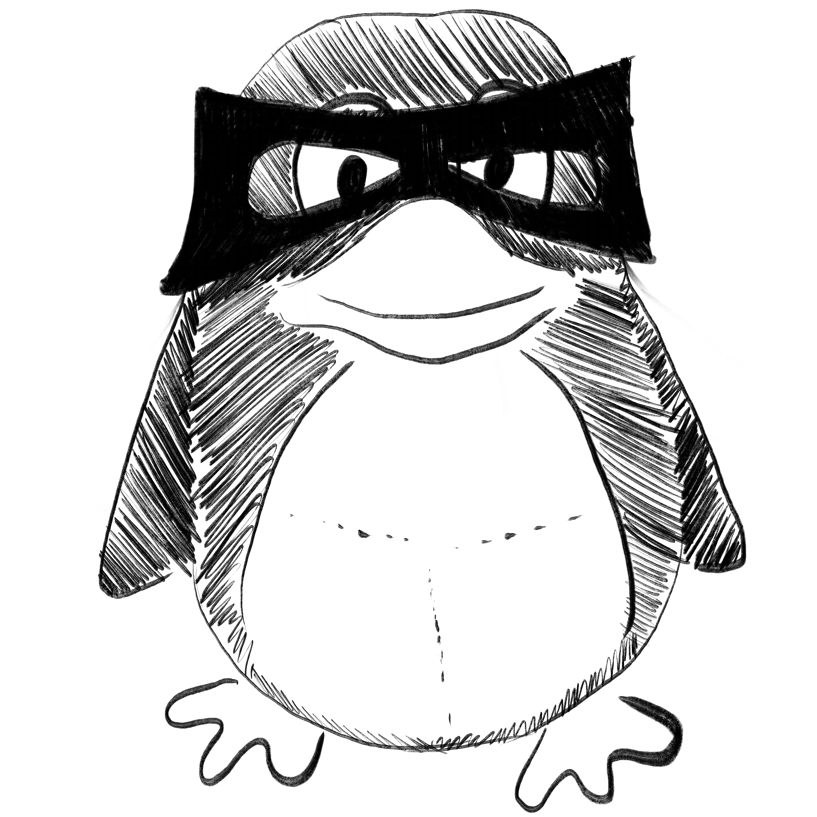
Logic-based mechanistic machine learning on high-content images reveals how drugs differentially regulate cardiac fibroblasts.
In bioRxiv : the preprint server for biology
UNLABELLED :
SIGNIFICANCE :
Nelson Anders R, Christiansen Steven L, Naegle Kristen M, Saucerman Jeffrey J
2023-Mar-02
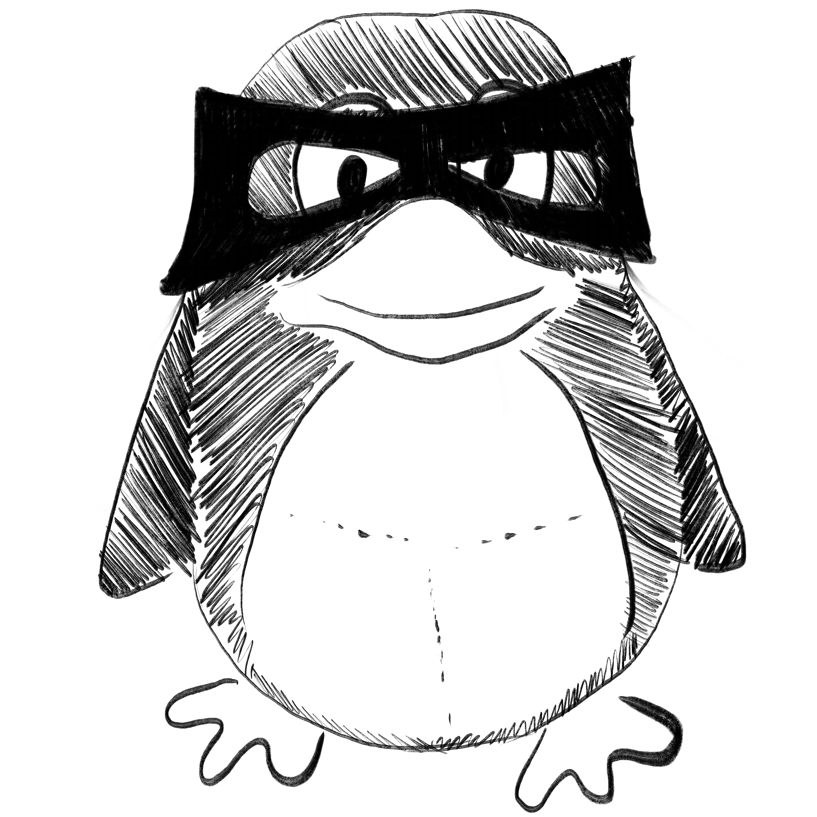
Pretraining strategies for effective promoter-driven gene expression prediction.
In bioRxiv : the preprint server for biology
Reddy Aniketh Janardhan, Herschl Michael H, Kolli Sathvik, Lu Amy X, Geng Xinyang, Kumar Aviral, Hsu Patrick D, Levine Sergey, Ioannidis Nilah M
2023-Feb-27
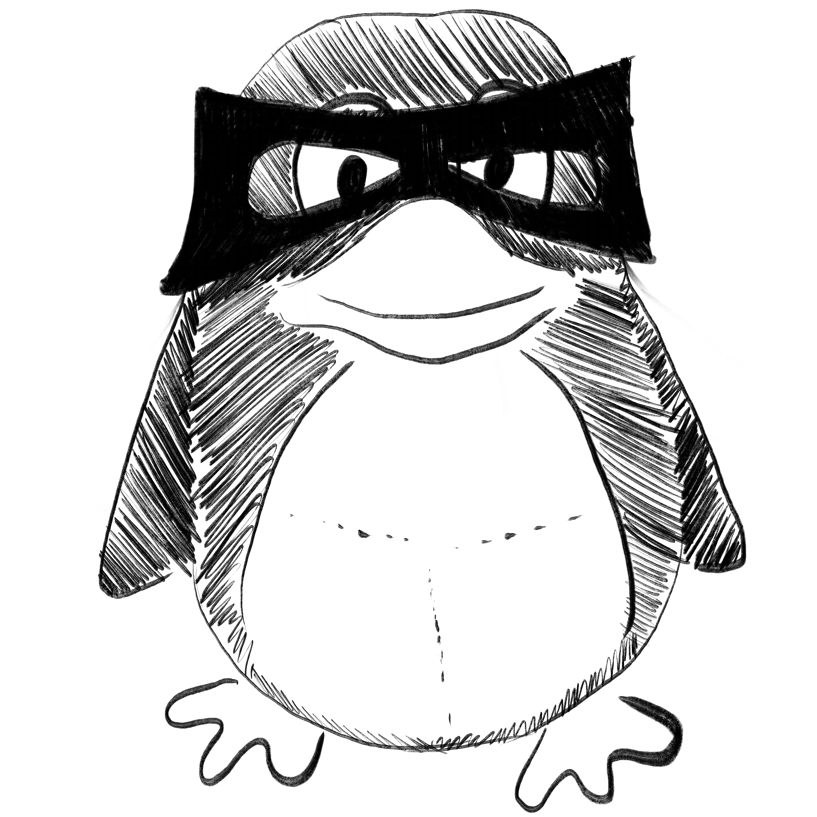
NMRQNet: a deep learning approach for automatic identification and quantification of metabolites using Nuclear Magnetic Resonance (NMR) in human plasma samples.
In bioRxiv : the preprint server for biology
Wang Wanli, Ma Li-Hua, Maletic-Savatic Mirjana, Liu Zhandong
2023-Mar-02
Beyond diagnosis: is there a role for radiomics in prostate cancer management?
In European radiology experimental
Stanzione Arnaldo, Ponsiglione Andrea, Alessandrino Francesco, Brembilla Giorgio, Imbriaco Massimo
2023-Mar-13
Artificial intelligence, Clinical decision-making, Prostatic neoplasms, Radiomics, Reproducibility of results
An anti-infodemic virtual center for the Americas.
In Revista panamericana de salud publica = Pan American journal of public health
Brooks Ian, D’Agostino Marcelo, Marti Myrna, McDowell Kate, Mejia Felipe, Betancourt-Cravioto Miguel, Gatzke Lisa, Hicks Elaine, Kyser Rebecca, Leicht Kevin, Pereira Dos Santos Eliane, Saw Jessica Jia-Wen, Tomio Ailin, Garcia Saiso Sebastian
2023
Americas, COVID-19, Public Health Informatics, artificial intelligence, communication, social media
Weekly Summary
Receive a weekly summary and discussion of the top papers of the week by leading researchers in the field.