Receive a weekly summary and discussion of the top papers of the week by leading researchers in the field.
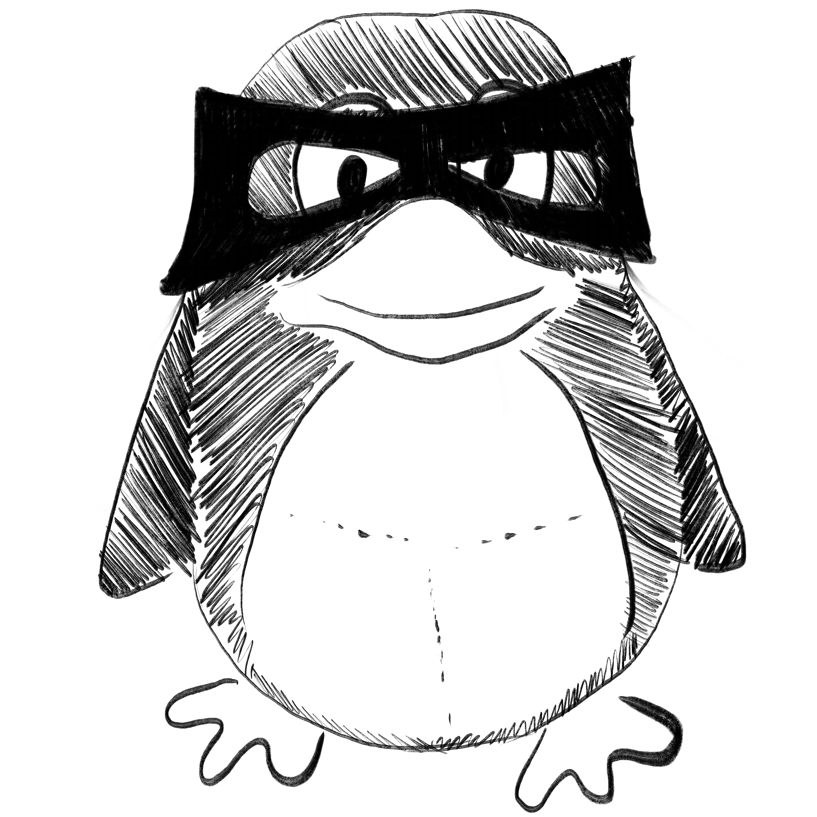
Diagnosis of triple negative breast cancer using expression data with several machine learning tools.
In Bioinformation
Pranaya Sankaranarayanan, Ragunath P K, Venkatesan P
2022
Artificial Neural Network (ANN), Breast Cancer, Logistic Regression, Machine learning, Multi-Layer Perceptron (MLP), Radial Basis Function (RBF), Triple Negative Breast Cancer (TNBC)
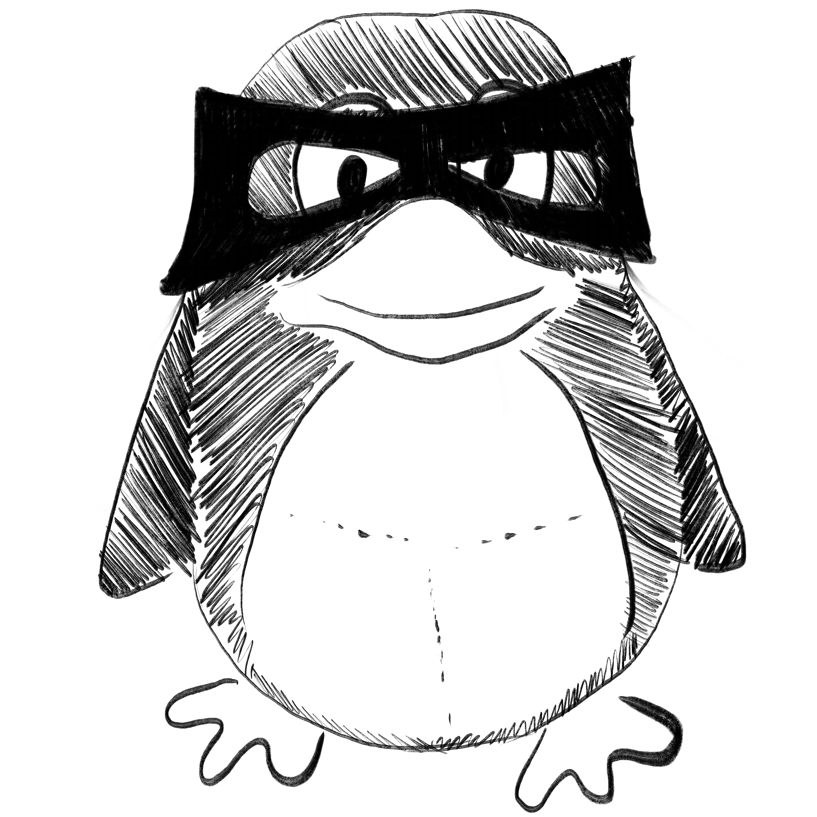
Predictors of Cognitive Decline in Healthy Middle-Aged Individuals with Asymptomatic Alzheimer's Disease.
In Research square
Lah James, Tandon Raghav, Zhao Liping, Watson Caroline, Elmor Morgan, Heilman Craig, Sanders Katherine, Hales Chadwick, Yang Huiying, Loring David, Goldstein Felicia, Hanfelt John, Duong Duc, Johnson Erik C B, Wingo Aliza, Wingo Thomas, Roberts Blaine, Seyfried Nicholas, Levey Allan, Mitchell Cassie
2023-Feb-28
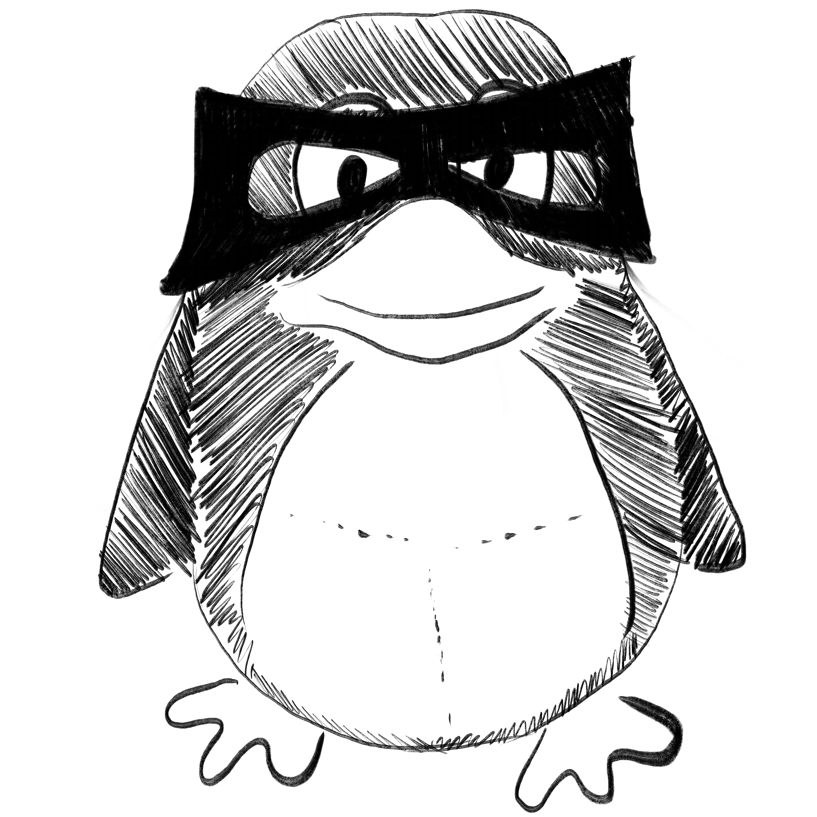
Novel Approach Explains Spatio-Spectral Interactions in Raw Electroencephalogram Deep Learning Classifiers.
In bioRxiv : the preprint server for biology
Ellis Charles A, Sattiraju Abhinav, Miller Robyn L, Calhoun Vince D
2023-Feb-27
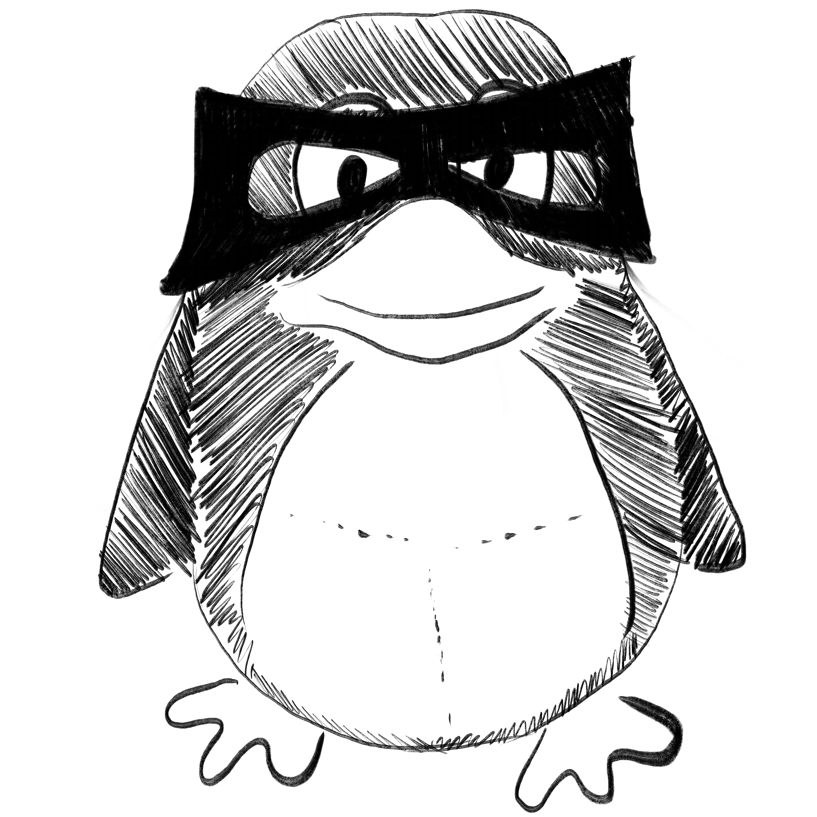
Brain Organoid Computing for Artificial Intelligence.
In bioRxiv : the preprint server for biology
Cai Hongwei, Ao Zheng, Tian Chunhui, Wu Zhuhao, Liu Hongcheng, Tchieu Jason, Gu Mingxia, Mackie Ken, Guo Feng
2023-Mar-01
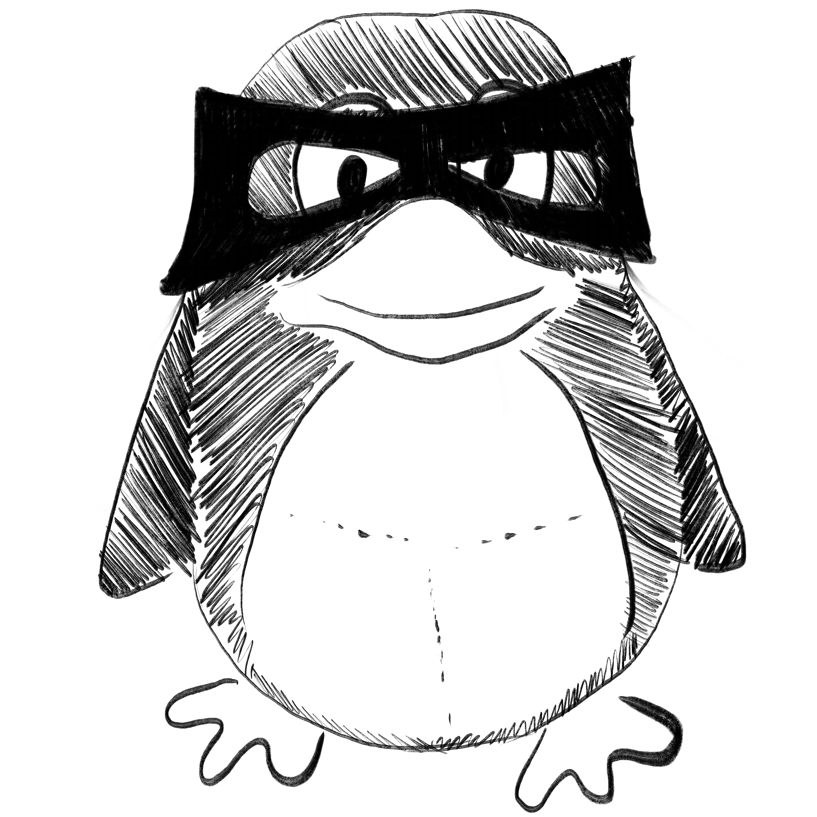
Predicting 'Brainage' in the Developmental Period using Structural MRI, Morphometric Similarity, and Machine Learning.
In Research square
Griffiths-King Daniel J, Wood Amanda G, Novak Jan
2023-Feb-28
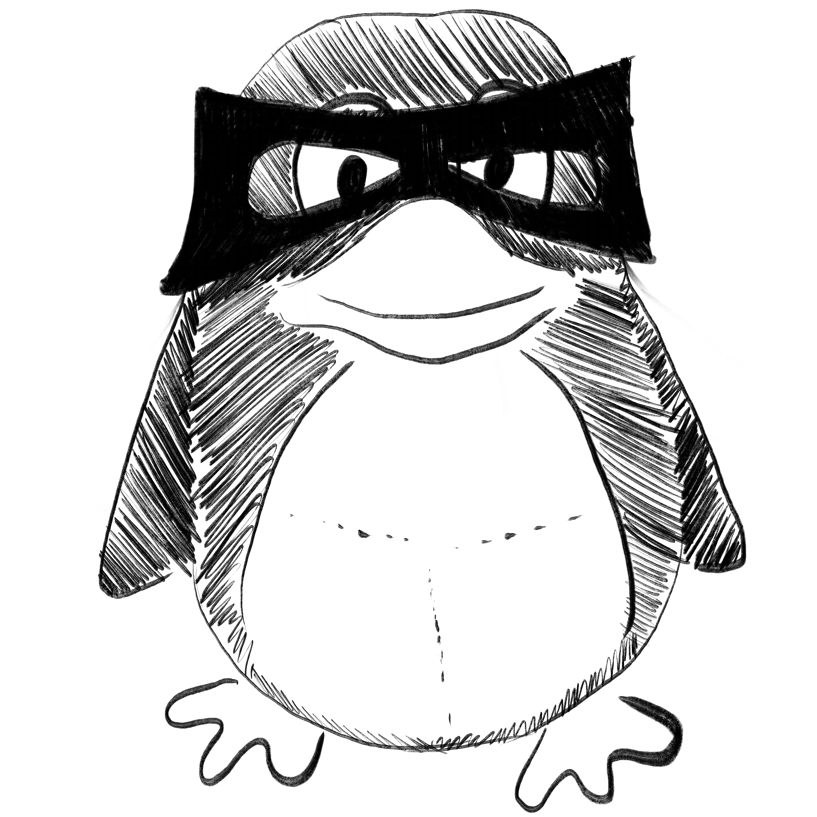
A data-fusion approach to identifying developmental dyslexia from multi-omics datasets.
In bioRxiv : the preprint server for biology
Carrion Jackson, Nandakumar Rohit, Shi Xiaojian, Gu Haiwei, Kim Yookyung, Raskind Wendy H, Peter Beate, Dinu Valentin
2023-Feb-27
Weekly Summary
Receive a weekly summary and discussion of the top papers of the week by leading researchers in the field.