Receive a weekly summary and discussion of the top papers of the week by leading researchers in the field.
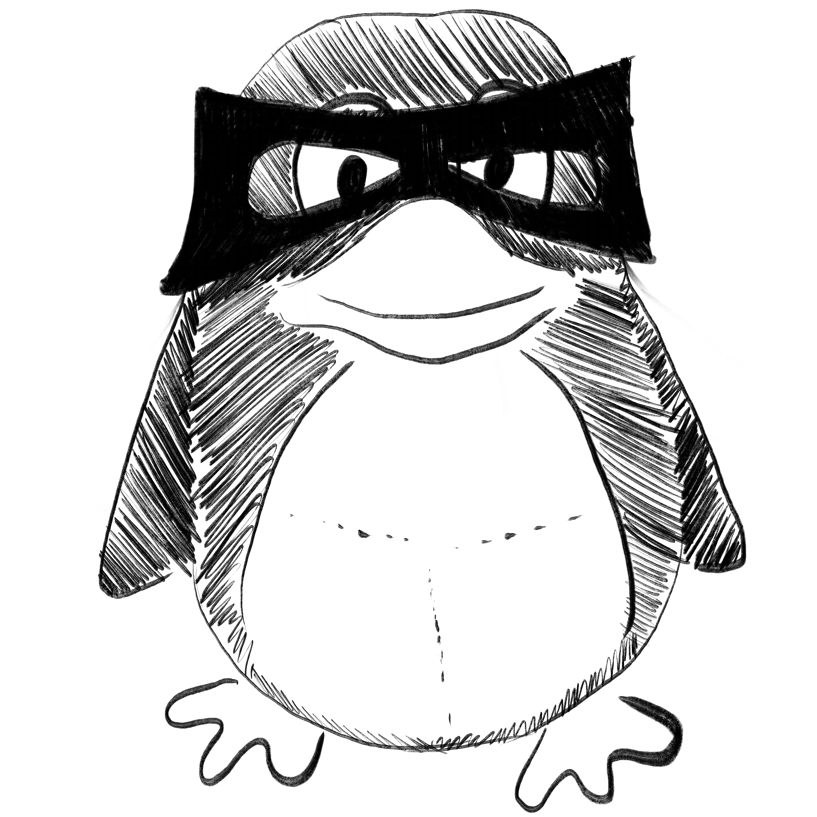
Prediction of brain tumor recurrence location based on multi-modal fusion and nonlinear correlation learning.
In Computerized medical imaging and graphics : the official journal of the Computerized Medical Imaging Society
Zhou Tongxue, Noeuveglise Alexandra, Modzelewski Romain, Ghazouani Fethi, Thureau Sébastien, Fontanilles Maxime, Ruan Su
2023-Mar-16
Brain tumor recurrence, Correlation learning, Deep learning, Location prediction, Multi-modal fusion
BTMF-GAN: A multi-modal MRI fusion generative adversarial network for brain tumors.
In Computers in biology and medicine
Liu Xiao, Chen Hongyi, Yao Chong, Xiang Rui, Zhou Kun, Du Peng, Liu Weifan, Liu Jie, Yu Zekuan
2023-Mar-09
Adaptive transformer, Image fusion, Multi-modal MRI, Salient loss
Intelligent decision support in medical triage: are people robust to biased advice?
In Journal of public health (Oxford, England)
BACKGROUND :
METHODS :
RESULTS :
CONCLUSIONS :
van der Stigchel Birgit, van den Bosch Karel, van Diggelen Jurriaan, Haselager Pim
2023-Mar-20
emergency care, ethics, health intelligence
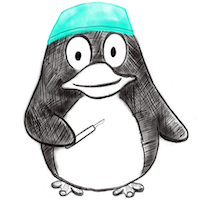
The Role of Scribes in Orthopaedics.
In JBJS reviews
» :
» :
» :
» :
Lam Michelle, Sabharwal Sanjeev
2023-Mar-01
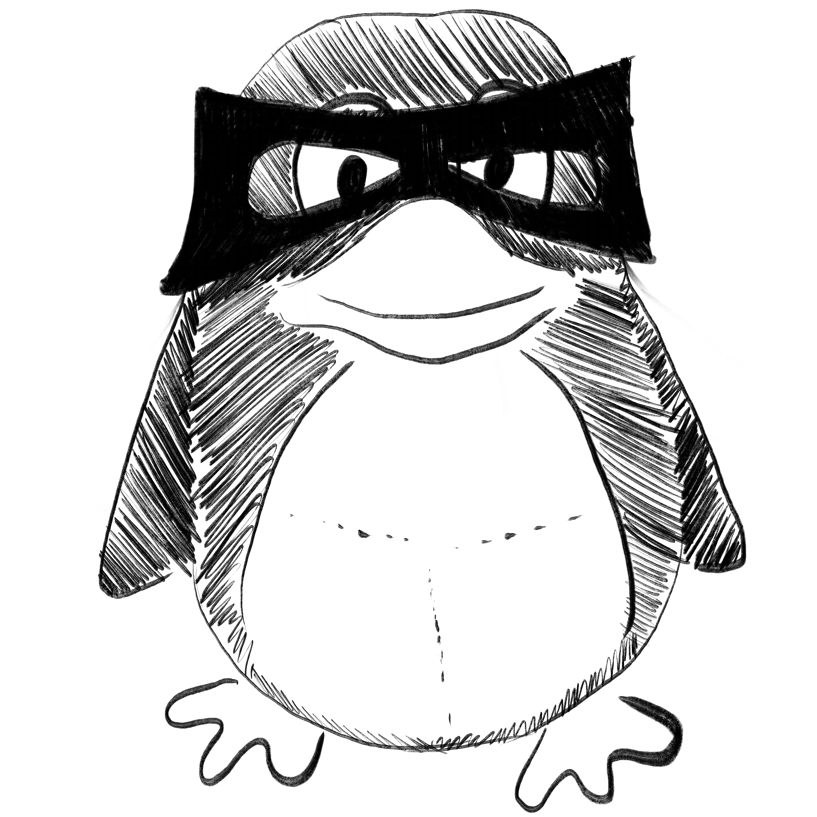
Mechanism of assembly of type 4 filaments: everything you always wanted to know (but were afraid to ask).
In Microbiology (Reading, England)
Pelicic Vladimir
2023-Mar
nanomachines, pili, pilin, type 2 secretion systems, type 4 filaments, type 4 pili
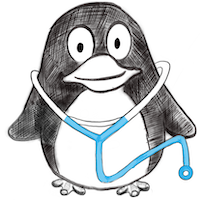
Predicting outcomes of continuous renal replacement therapy using body composition monitoring: a deep-learning approach.
In Scientific reports ; h5-index 158.0
Yoo Kyung Don, Noh Junhyug, Bae Wonho, An Jung Nam, Oh Hyung Jung, Rhee Harin, Seong Eun Young, Baek Seon Ha, Ahn Shin Young, Cho Jang-Hee, Kim Dong Ki, Ryu Dong-Ryeol, Kim Sejoong, Lim Chun Soo, Lee Jung Pyo
2023-Mar-21
Weekly Summary
Receive a weekly summary and discussion of the top papers of the week by leading researchers in the field.