Receive a weekly summary and discussion of the top papers of the week by leading researchers in the field.
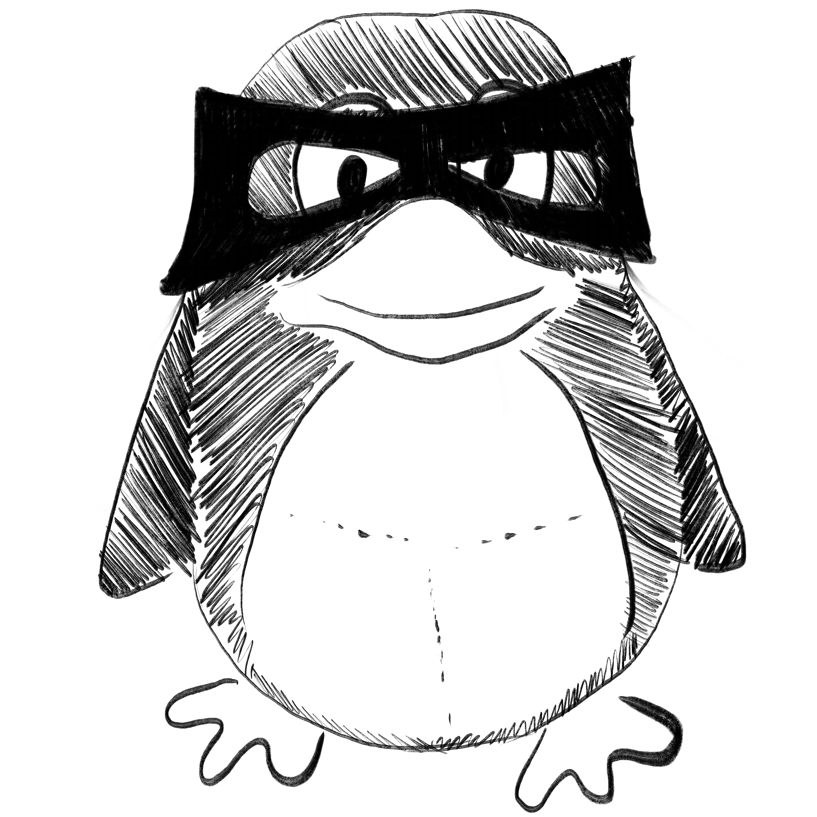
A Comprehensive Survey on Federated Learning Techniques for Healthcare Informatics.
In Computational intelligence and neuroscience
Dasaradharami Reddy K, Gadekallu Thippa Reddy
2023
Next Generation Infectious Diseases Monitoring Gages via Incremental Federated Learning: Current Trends and Future Possibilities.
In Computational intelligence and neuroscience
Javed Iqra, Iqbal Uzair, Bilal Muhammad, Shahzad Basit, Chung Tae-Sun, Attique Muhammad
2023
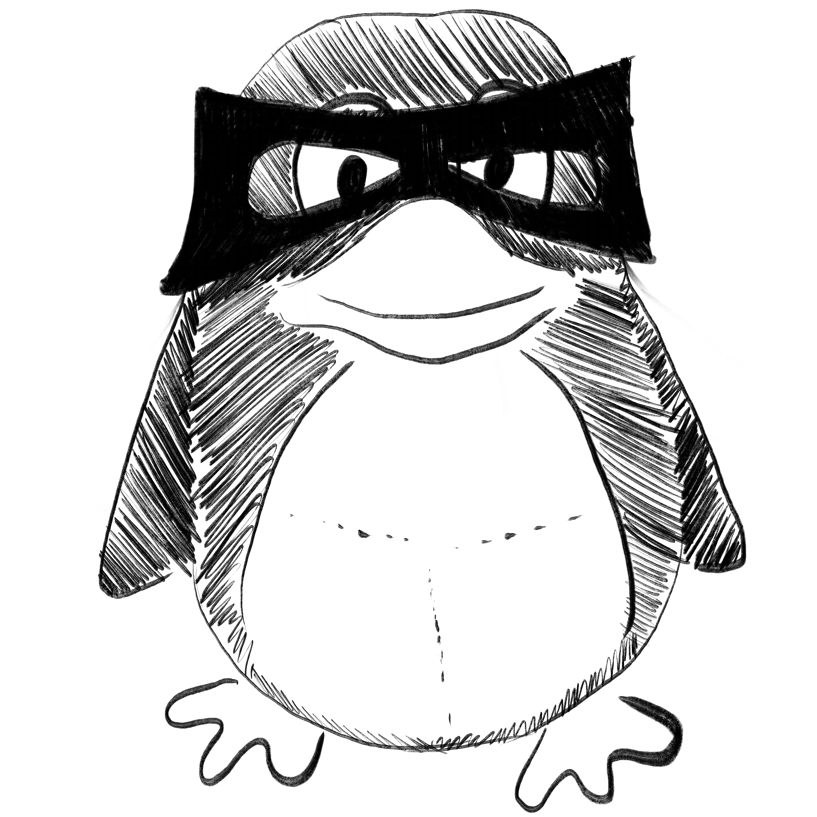
Biometric Authentication and Correlation Analysis Based on CNN-SRU Hybrid Neural Network Model.
In Computational intelligence and neuroscience
Zhang Houding, Yang Zexian
2023
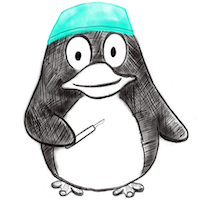
Machine Learning Techniques for Antimicrobial Resistance Prediction of Pseudomonas Aeruginosa from Whole Genome Sequence Data.
In Computational intelligence and neuroscience
AIM :
METHOD :
RESULTS :
CONCLUSION :
Noman Sohail M, Zeeshan Muhammad, Arshad Jehangir, Deressa Amentie Melkamu, Shafiq Muhammad, Yuan Yumeng, Zeng Mi, Li Xin, Xie Qingdong, Jiao Xiaoyang
2023
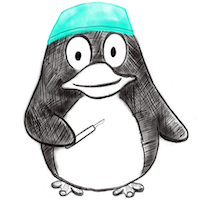
Supervised Machine Learning Based Noninvasive Prediction of Atrial Flutter Mechanism from P-to-P Interval Variability under Imbalanced Dataset Conditions.
In Computational intelligence and neuroscience
Gul Muhammad Usman, Kamarul Azman Muhammad Haziq, Kadir Kushsairy Abdul, Shah Jawad Ali, Hussen Seada
2023
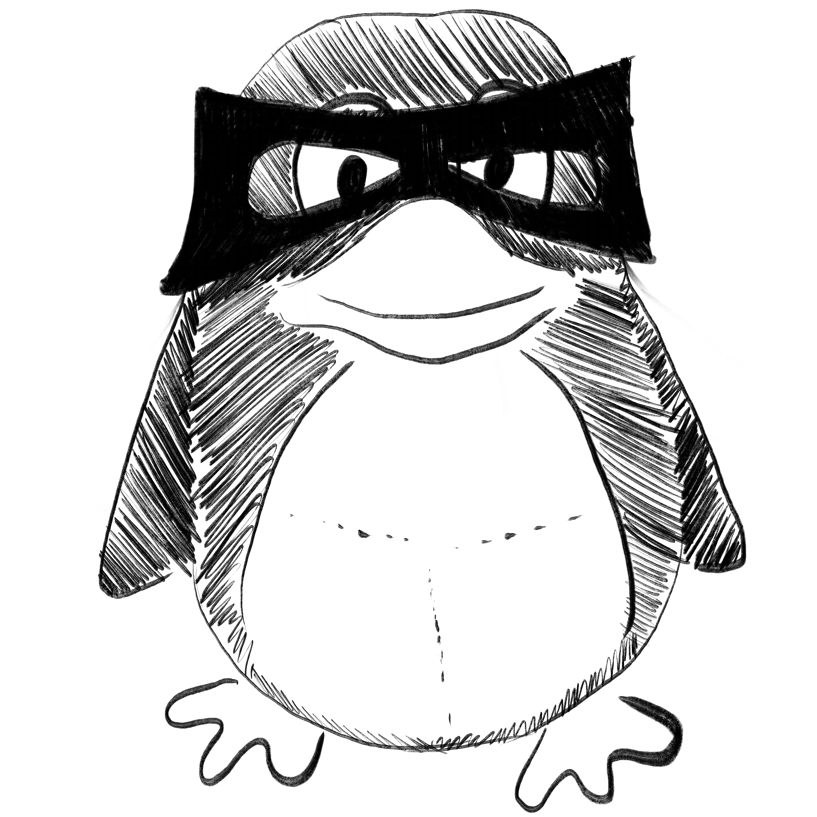
Retracted: Design of Financial Risk Control Model Based on Deep Learning Neural Network.
In Computational intelligence and neuroscience
Neuroscience Computational Intelligence And
2023
Weekly Summary
Receive a weekly summary and discussion of the top papers of the week by leading researchers in the field.