Receive a weekly summary and discussion of the top papers of the week by leading researchers in the field.
Implementing cone-beam computed tomography-guided online adaptive radiotherapy in cervical cancer.
In Clinical and translational radiation oncology
BACKGROUND AND PURPOSE :
MATERIAL AND METHODS :
RESULTS :
CONCLUSION :
Shelley Charlotte E, Bolt Matthew A, Hollingdale Rachel, Chadwick Susan J, Barnard Andrew P, Rashid Miriam, Reinlo Selina C, Fazel Nawda, Thorpe Charlotte R, Stewart Alexandra J, South Chris P, Adams Elizabeth J
2023-May
Artificial intelligence, Automated treatment planning, Cervical cancer, Cone-beam computed tomography (CBCT), External beam radiotherapy, Image-guided radiotherapy (IGRT), Online adaptive radiotherapy
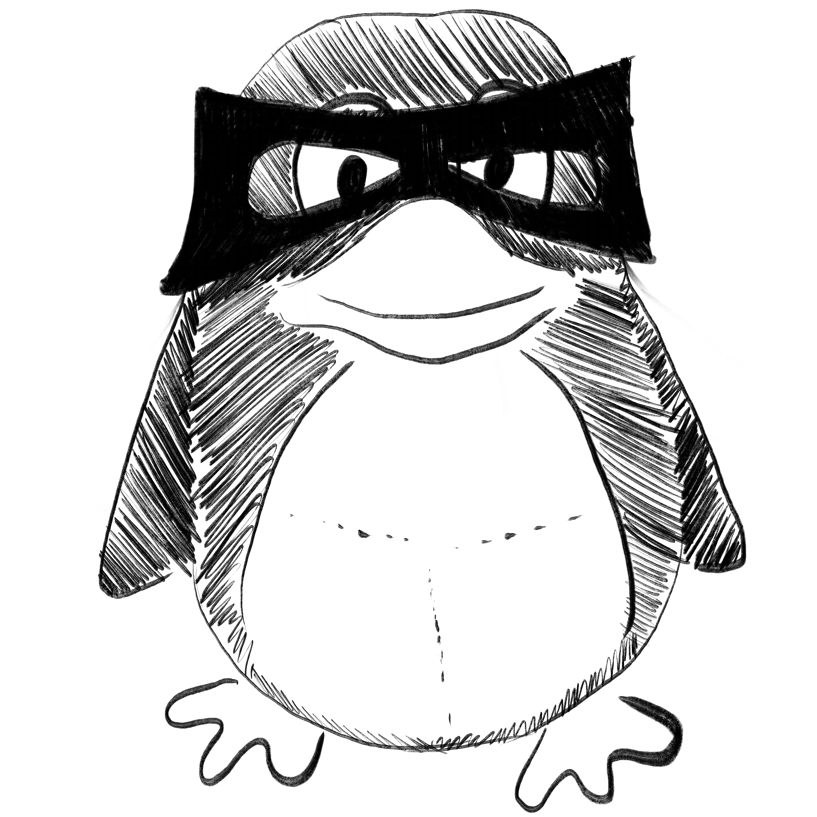
Multi-Ideology, Multiclass Online Extremism Dataset, and Its Evaluation Using Machine Learning.
In Computational intelligence and neuroscience
Gaikwad Mayur, Ahirrao Swati, Phansalkar Shraddha, Kotecha Ketan, Rani Shalli
2023
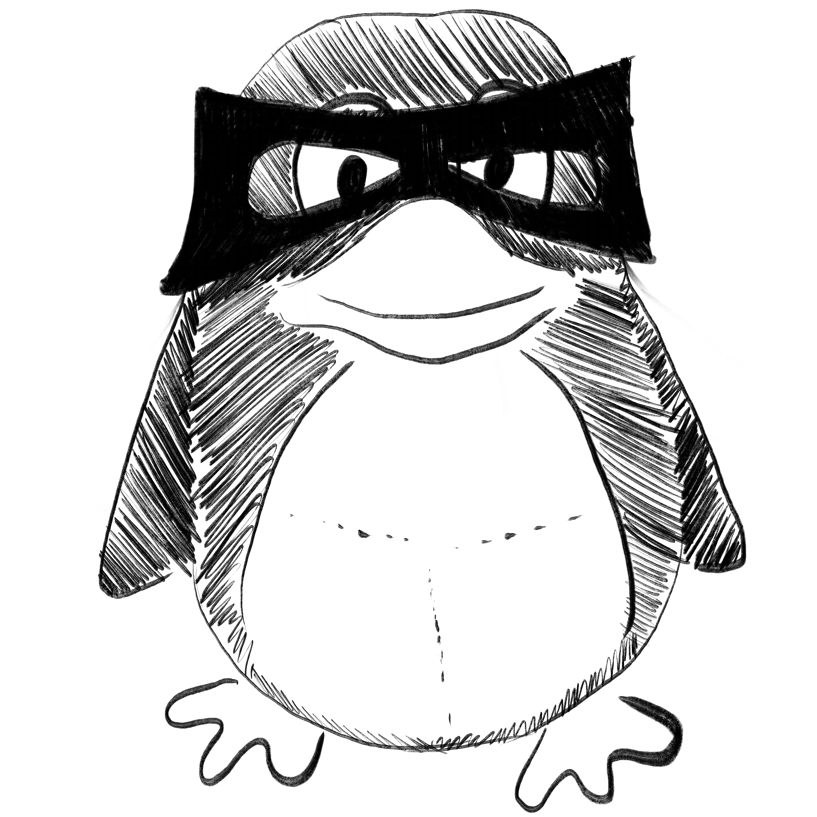
Prediction of protein-protein interactions using sequences of intrinsically disordered regions.
In Proteins
Kibar Gözde, Vingron Martin
2023-Mar-13
intrinsic disorder, intrinsically disordered proteins, machine learning, prediction, protein-protein interactions
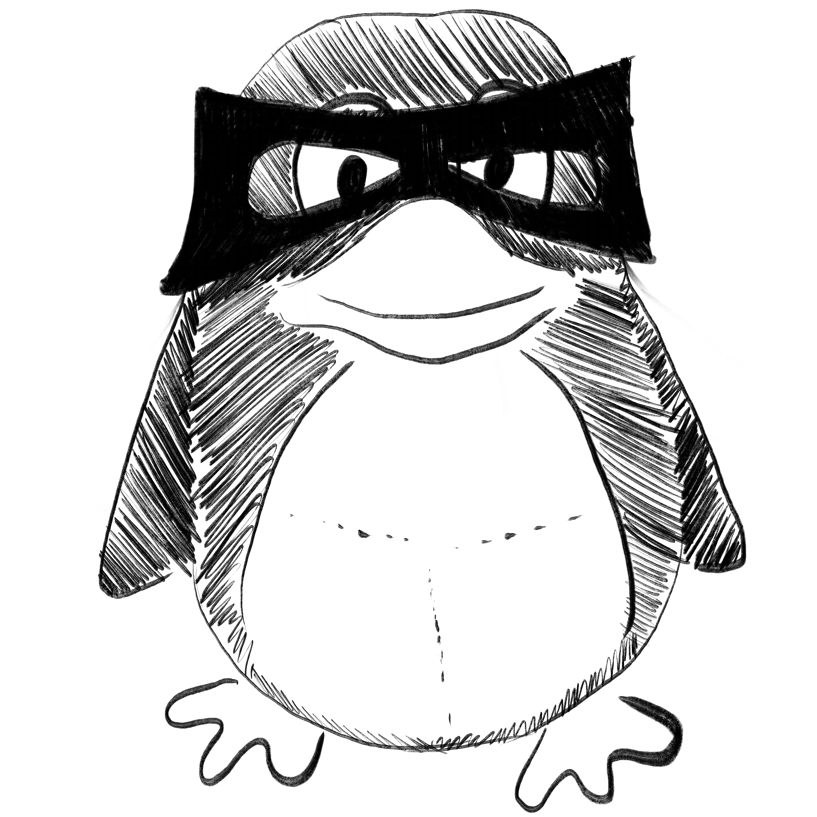
Pathogen-driven cancers from a structural perspective: Targeting host-pathogen protein-protein interactions.
In Frontiers in oncology
Ozdemir Emine Sila, Nussinov Ruth
2023
artificial intelligence, cancer therapeutics, drug discovery, host-pathogen interactions, machine learning, protein-protein interactions
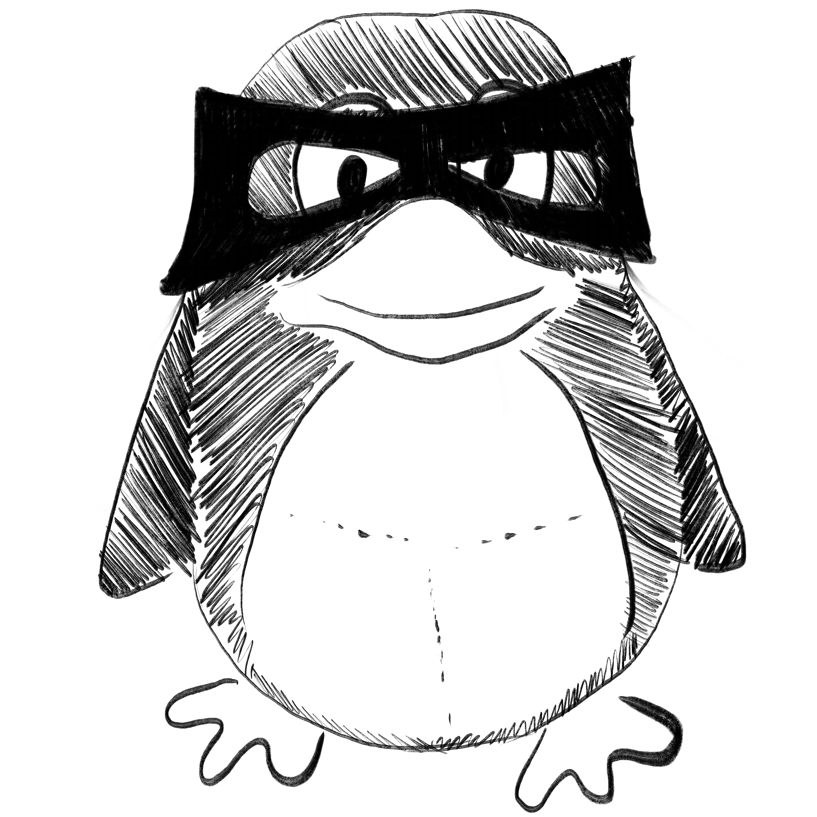
FPUS23: An Ultrasound Fetus Phantom Dataset with Deep Neural Network Evaluations for Fetus Orientations, Fetal Planes, and Anatomical Features
ArXiv Preprint
Bharath Srinivas Prabakaran, Paul Hamelmann, Erik Ostrowski, Muhammad Shafique
2023-03-14
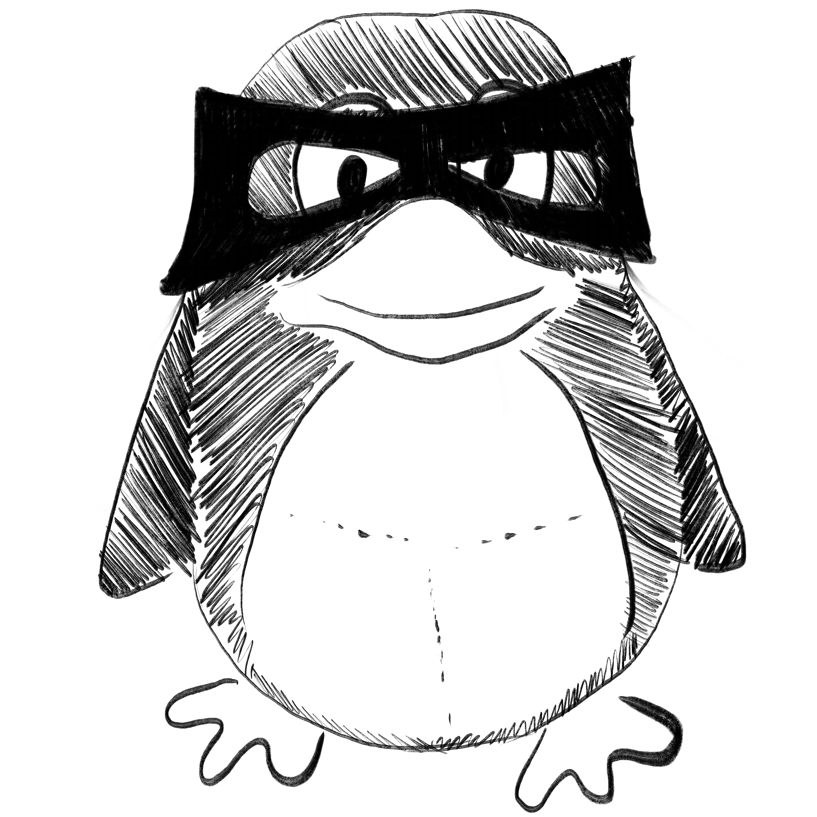
Analyzing the Relationship among Social Capital, Dynamic Capability, and Farmers' Cooperative Performance Using Lightweight Deep Learning Model: A Case Study of Liaoning Province.
In Computational intelligence and neuroscience
Zhang Simeng, Wu Dongli
2023
Weekly Summary
Receive a weekly summary and discussion of the top papers of the week by leading researchers in the field.