Receive a weekly summary and discussion of the top papers of the week by leading researchers in the field.
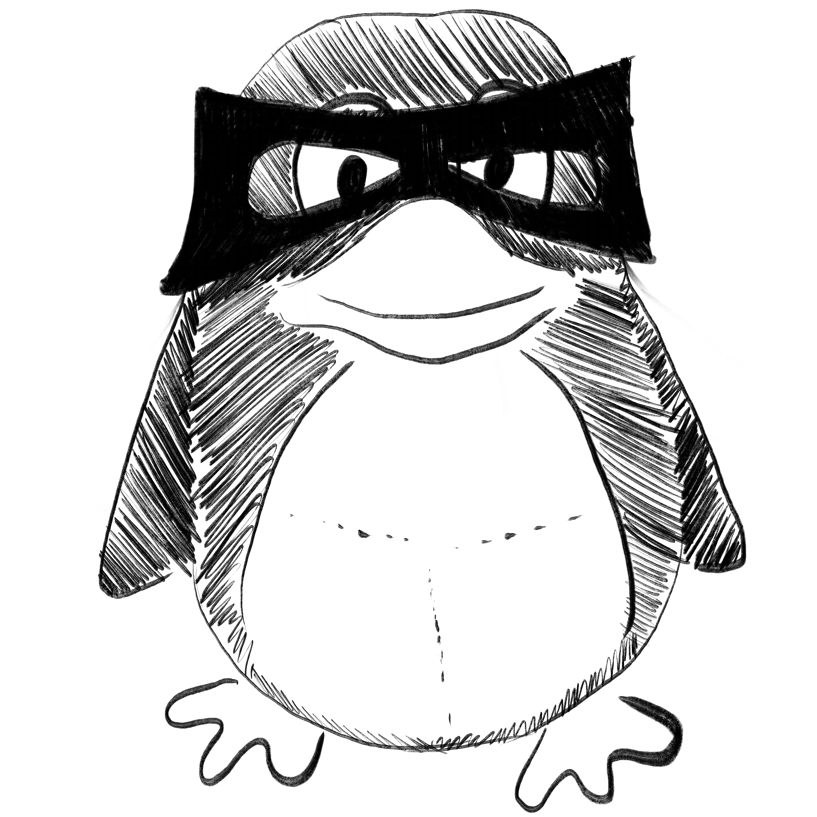
DeepLBCEPred: A Bi-LSTM and multi-scale CNN-based deep learning method for predicting linear B-cell epitopes.
In Frontiers in microbiology
Qi Yue, Zheng Peijie, Huang Guohua
2023
B-cell, CNN, LSTM, epitope, protein sequence
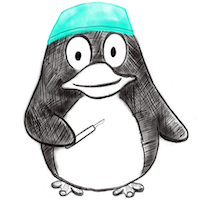
Changes in the gut microbiome of patients with type a aortic dissection.
In Frontiers in microbiology
OBJECTIVE :
METHODS :
RESULTS :
CONCLUSION :
Jiang Fei, Cai Meiling, Peng Yanchun, Li Sailan, Liang Bing, Ni Hong, Lin Yanjuan
2023
16S rDNA sequencing, SCFAs, aortic dissection, gut microbiome, metabolomics
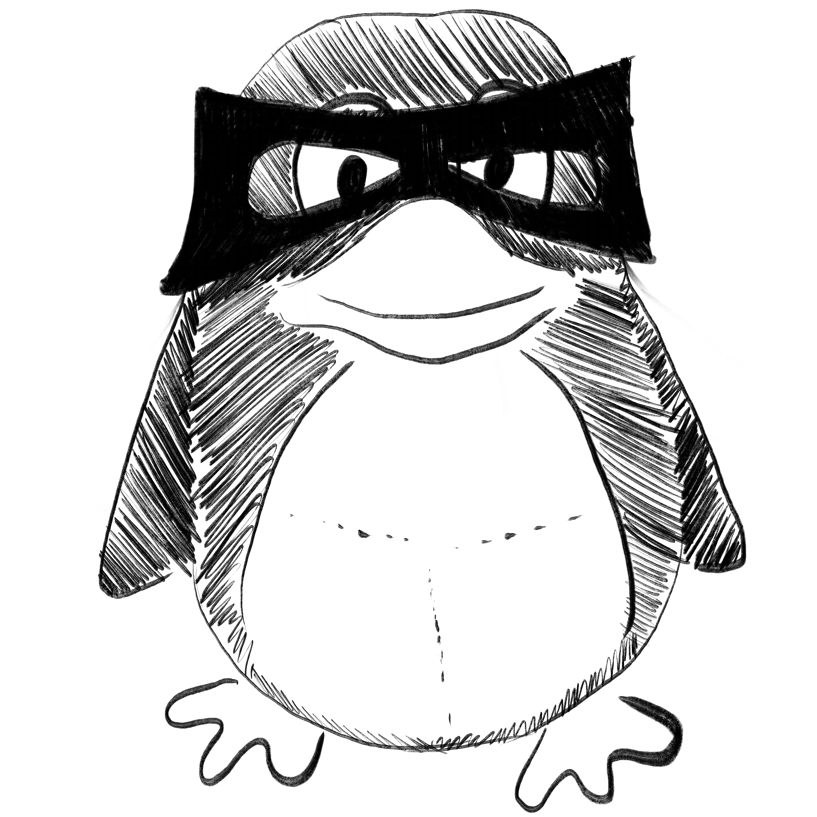
Machine learning-based ozone and PM2.5 forecasting: Application to multiple AQS sites in the Pacific Northwest.
In Frontiers in big data
Fan Kai, Dhammapala Ranil, Harrington Kyle, Lamb Brian, Lee Yunha
2023
PM2.5, air quality forecasts, machine learning, multiple linear regression, ozone, random forest
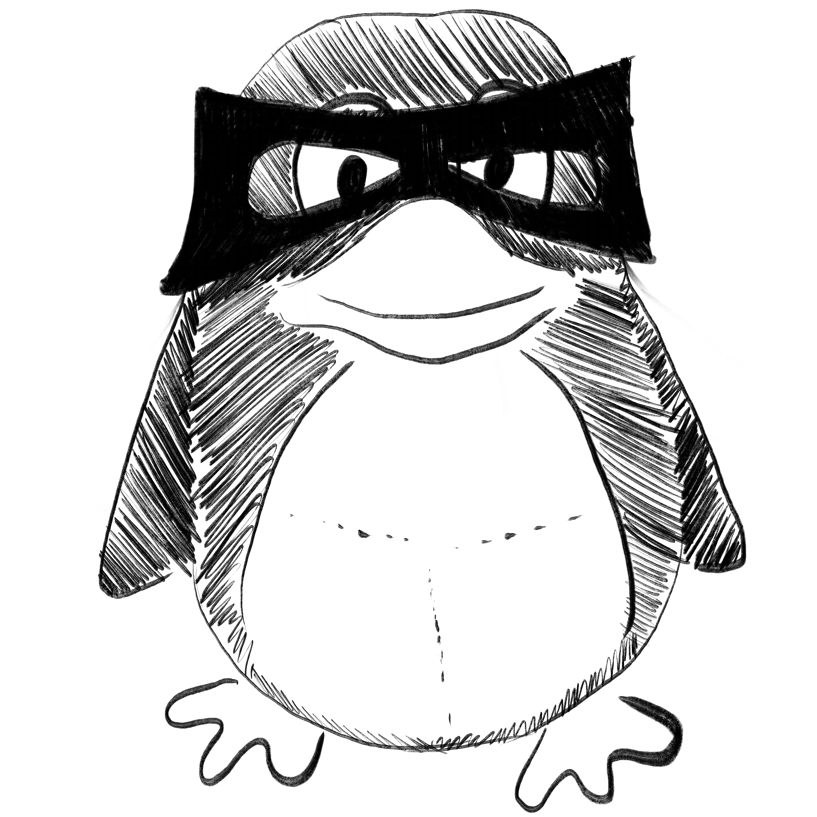
Automatic measurement of exophthalmos based orbital CT images using deep learning.
In Frontiers in cell and developmental biology
Zhang Yinghuai, Rao Jing, Wu Xingyang, Zhou Yongjin, Liu Guiqin, Zhang Hua
2023
CT images, deep learning, exophthalmos, orbital diseases, thyroid-associated ophthalmopathy
Prolonged air leak after video-assisted thoracic anatomical pulmonary resections: a clinical predicting model based on data from the Italian VATS group registry, a machine learning approach.
In Journal of thoracic disease ; h5-index 52.0
BACKGROUND :
METHODS :
RESULTS :
CONCLUSIONS :
Divisi Duilio, Pipitone Marco, Perkmann Reinhold, Bertolaccini Luca, Curcio Carlo, Baldinelli Francesco, Crisci Roberto, Zaraca Francesco
2023-Feb-28
Prolonged air leak (PAL), risk factors, risk predictive model, video-assisted thoracoscopic surgery lobectomy (VATS lobectomy)
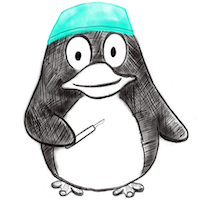
Value of contrast-enhanced magnetic resonance imaging-T2WI-based radiomic features in distinguishing lung adenocarcinoma from lung squamous cell carcinoma with solid components >8 mm.
In Journal of thoracic disease ; h5-index 52.0
BACKGROUND :
METHODS :
RESULTS :
CONCLUSIONS :
Yang Maoyuan, Shi Liang, Huang Tianwei, Li Guangzheng, Shao Hancheng, Shen Yijun, Zhu Jun, Ni Bin
2023-Feb-28
Radiomics, lung adenocarcinoma, lung squamous cell carcinoma, machine learning, nomogram
Weekly Summary
Receive a weekly summary and discussion of the top papers of the week by leading researchers in the field.