Receive a weekly summary and discussion of the top papers of the week by leading researchers in the field.
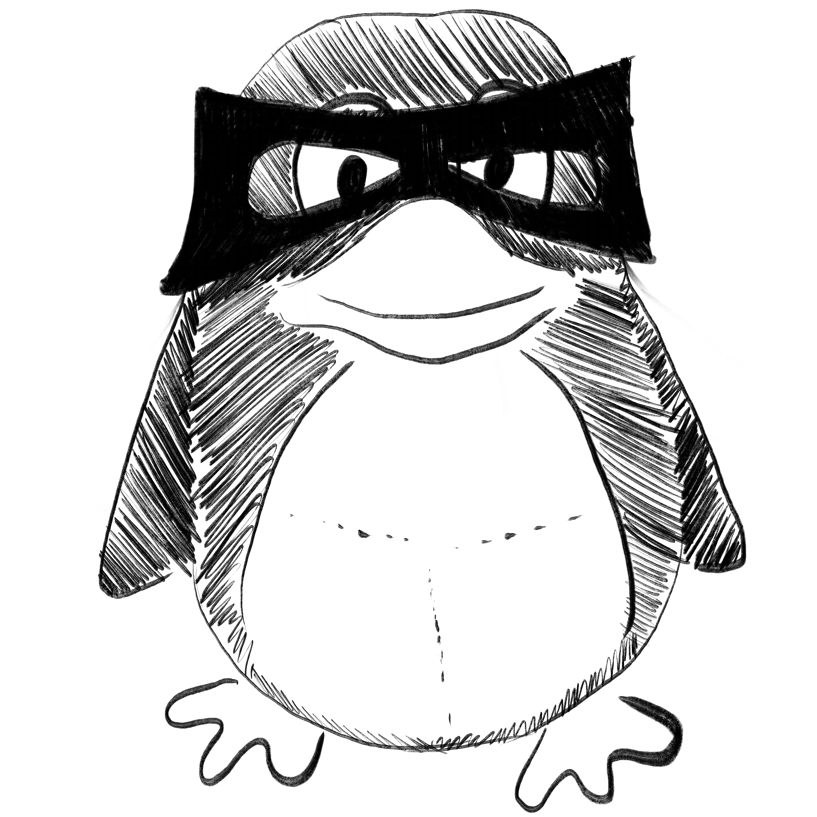
Responsible model deployment via model-agnostic uncertainty learning.
In Machine learning
Lahoti Preethi, Gummadi Krishna, Weikum Gerhard
2023
Failure analysis, Trustworthy ML, Uncertainty modeling
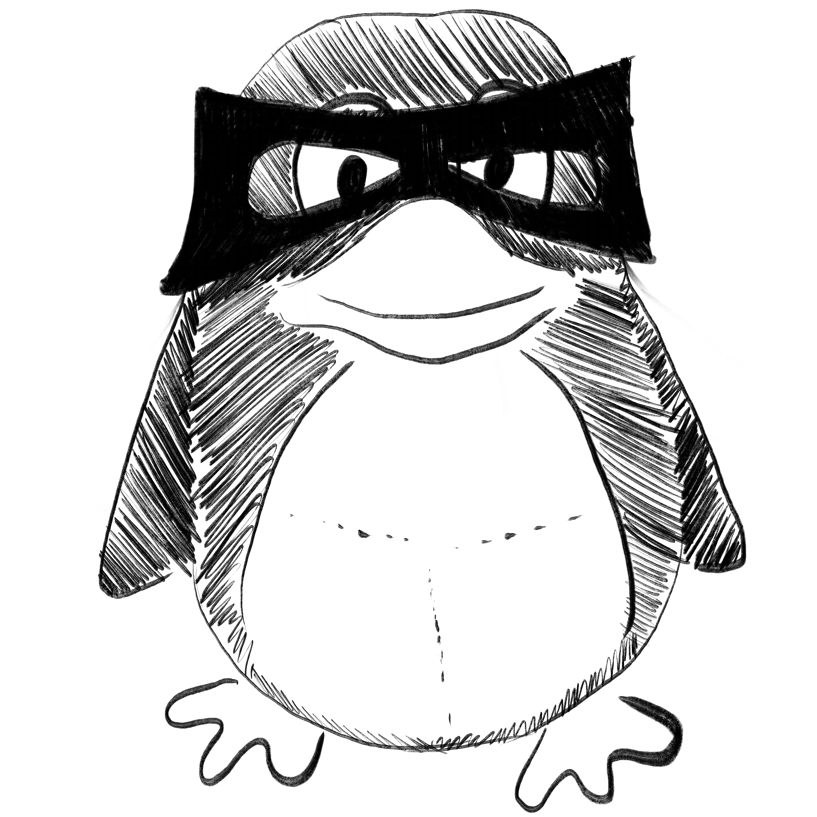
Stroke risk study based on deep learning-based magnetic resonance imaging carotid plaque automatic segmentation algorithm.
In Frontiers in cardiovascular medicine
INTRODUCTION :
METHODS :
RESULTS :
CONCLUSION :
Chen Ya-Fang, Chen Zhen-Jie, Lin You-Yu, Lin Zhi-Qiang, Chen Chun-Nuan, Yang Mei-Li, Zhang Jin-Yin, Li Yuan-Zhe, Wang Yi, Huang Yin-Hui
2023
MRI carotid plaque, YOLO V3, deep learning, stroke risk, transfer learning
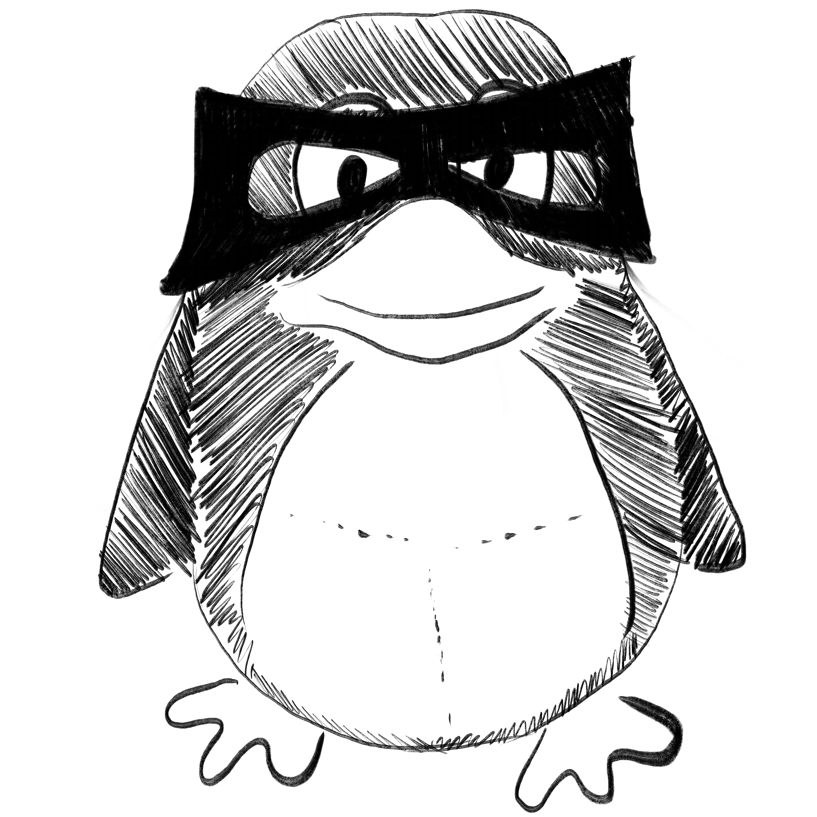
Artificial intelligence guidance of advanced heart failure therapies: A systematic scoping review.
In Frontiers in cardiovascular medicine
INTRODUCTION :
METHODS :
RESULTS :
CONCLUSION :
Al-Ani Mohammad A, Bai Chen, Hashky Amal, Parker Alex M, Vilaro Juan R, Aranda Juan M, Shickel Benjamin, Rashidi Parisa, Bihorac Azra, Ahmed Mustafa M, Mardini Mamoun T
2023
LVAD, artificial intelligence, deep learning, heart transplantation, machine learning, mechanical circulatory support
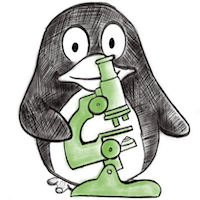
5-Year progression prediction of endplate defects: Utilizing the EDPP-Flow convolutional neural network based on unbalanced data.
In Journal of orthopaedics
BACKGROUND :
PURPOSE :
MATERIALS AND METHODS :
RESULTS :
CONCLUSION :
Cheung Jason Pui Yin, Kuang Xihe, Zhang Teng, Wang Kun, Yang Cao
2023-Apr
Convolutional neural network, Deep learning, Disease progression prediction, Lumbar disc degeneration, Unbalanced data
External validation of a convolutional neural network for the automatic segmentation of intraprostatic tumor lesions on 68Ga-PSMA PET images.
In Frontiers in medicine
INTRODUCTION :
METHODS :
RESULTS :
DISCUSSION :
Ghezzo Samuele, Mongardi Sofia, Bezzi Carolina, Samanes Gajate Ana Maria, Preza Erik, Gotuzzo Irene, Baldassi Francesco, Jonghi-Lavarini Lorenzo, Neri Ilaria, Russo Tommaso, Brembilla Giorgio, De Cobelli Francesco, Scifo Paola, Mapelli Paola, Picchio Maria
2023
PSMA, convolutional neural network, external validation, prostate cancer, segmentation
Identifying patients with acute ischemic stroke within a 6-h window for the treatment of endovascular thrombectomy using deep learning and perfusion imaging.
In Frontiers in medicine
INTRODUCTION :
METHODS :
RESULTS :
DISCUSSION :
Gao Hongyu, Bian Yueyan, Cheng Gen, Yu Huan, Cao Yuze, Zhang Huixue, Wang Jianjian, Li Qian, Yang Qi, Wang Lihua
2023
acute ischemic stroke, deep learning, endovascular thrombectomy, perfusion imaging, stroke onset time
Weekly Summary
Receive a weekly summary and discussion of the top papers of the week by leading researchers in the field.