Receive a weekly summary and discussion of the top papers of the week by leading researchers in the field.
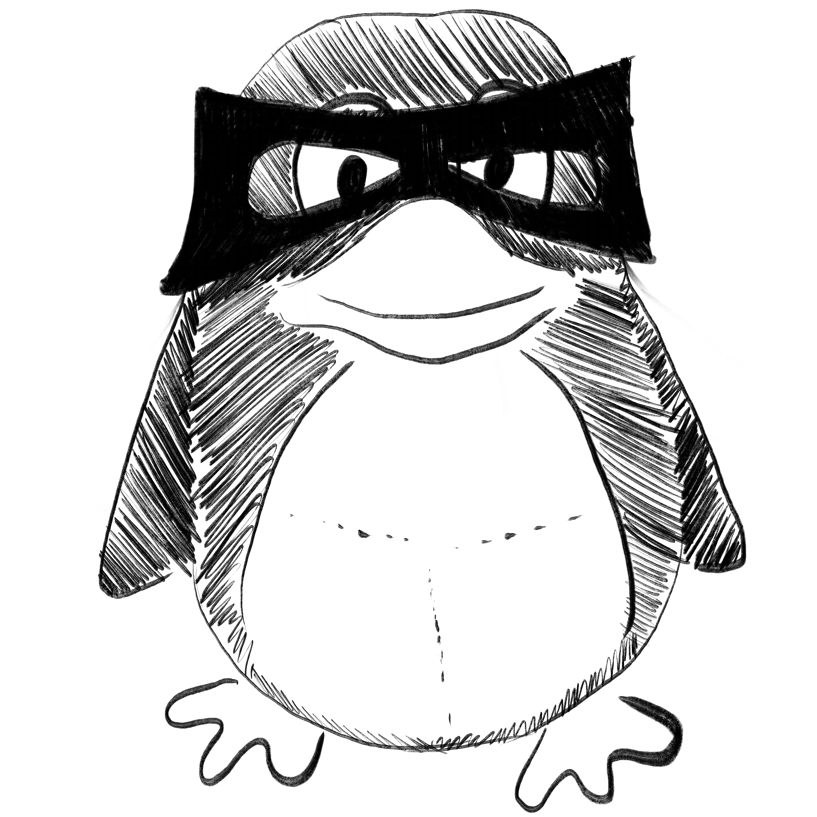
Investigating a neural language model's replicability of psycholinguistic experiments: A case study of NPI licensing.
In Frontiers in psychology ; h5-index 92.0
Shin Unsub, Yi Eunkyung, Song Sanghoun
2023
BERT, NPI licensing, grammatical illusion, licensing strength, negative polarity items, neural language model, psycholinguistics, scale of negativity
Exploring the impact of sentiment on multi-dimensional information dissemination using COVID-19 data in China.
In Computers in human behavior ; h5-index 125.0
Luo Han, Meng Xiao, Zhao Yifei, Cai Meng
2023-Jul
COVID-19, Emotional response, Information authority, Information dissemination, Information richness, Topic influence
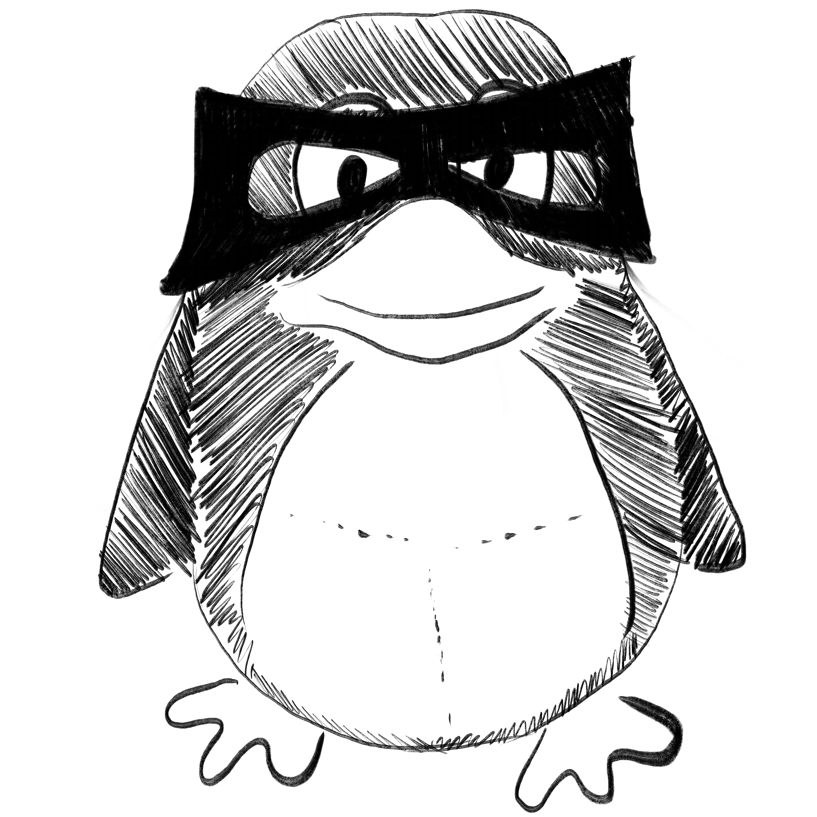
Normalization and possibility of classification analysis using the optimal warping paths of dynamic time warping in gait analysis.
In Journal of exercise rehabilitation
Lee Hyun-Seob
2023-Feb
Classification analysis, Dynamic time warping, Gait, Machine learning, Similarity
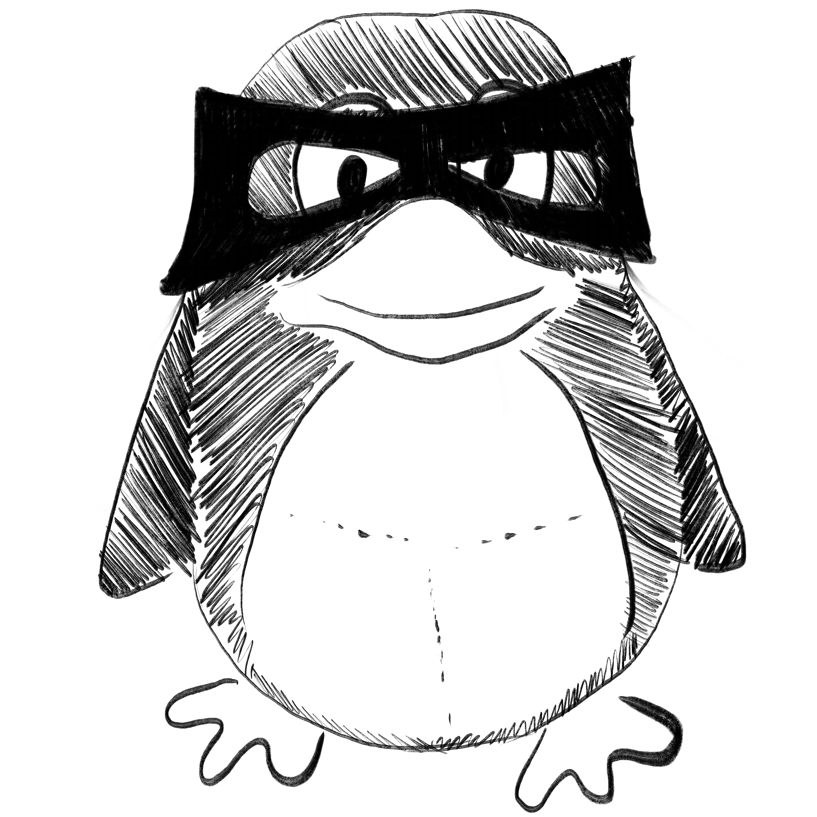
Pathogen-driven cancers from a structural perspective: Targeting host-pathogen protein-protein interactions.
In Frontiers in oncology
Ozdemir Emine Sila, Nussinov Ruth
2023
artificial intelligence, cancer therapeutics, drug discovery, host-pathogen interactions, machine learning, protein-protein interactions
Incorporating the synthetic CT image for improving the performance of deformable image registration between planning CT and cone-beam CT.
In Frontiers in oncology
OBJECTIVE :
METHODS :
RESULTS :
CONCLUSION :
Li Na, Zhou Xuanru, Chen Shupeng, Dai Jingjing, Wang Tangsheng, Zhang Chulong, He Wenfeng, Xie Yaoqin, Liang Xiaokun
2023
breast cancer, deep learning, deformable image registration (DIR), radiation therapy, synthetic image
Artificial-intelligence-based computed tomography histogram analysis predicting tumor invasiveness of lung adenocarcinomas manifesting as radiological part-solid nodules.
In Frontiers in oncology
BACKGROUND :
METHODS :
RESULTS :
CONCLUSION :
Gao Jian, Qi Qingyi, Li Hao, Wang Zhenfan, Sun Zewen, Cheng Sida, Yu Jie, Zeng Yaqi, Hong Nan, Wang Dawei, Wang Huiyang, Yang Feng, Li Xiao, Li Yun
2023
CT histogram, lung adenocarcinoma, part-solid nodule, three-dimensional index, tumor invasiveness
Weekly Summary
Receive a weekly summary and discussion of the top papers of the week by leading researchers in the field.