Receive a weekly summary and discussion of the top papers of the week by leading researchers in the field.
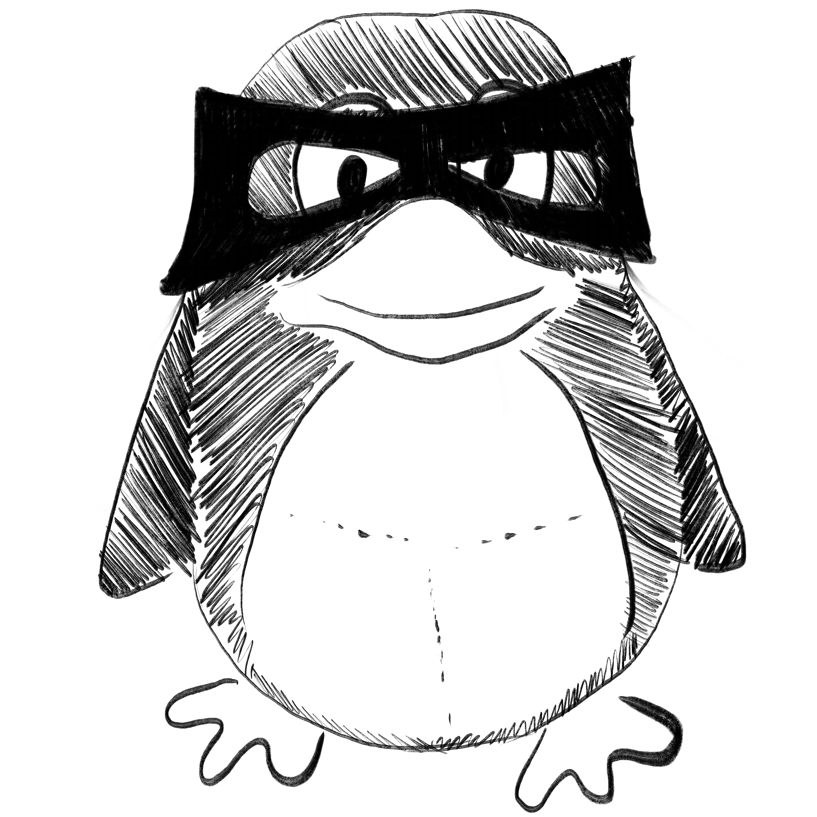
i-Sheet: A Low-Cost Bedsheet Sensor for Remote Diagnosis of Isolated Individuals.
In IEEE sensors journal
Tapwal Riya, Misra Sudip, Deb Pallav Kumar
2023-Jan
Artificial intelligence, COVID-19, remote monitoring, sensors, smart bedsheet
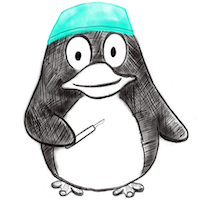
HALOS: Hallucination-free Organ Segmentation after Organ Resection Surgery
ArXiv Preprint
Anne-Marie Rickmann, Murong Xu, Tom Nuno Wolf, Oksana Kovalenko, Christian Wachinger
2023-03-14
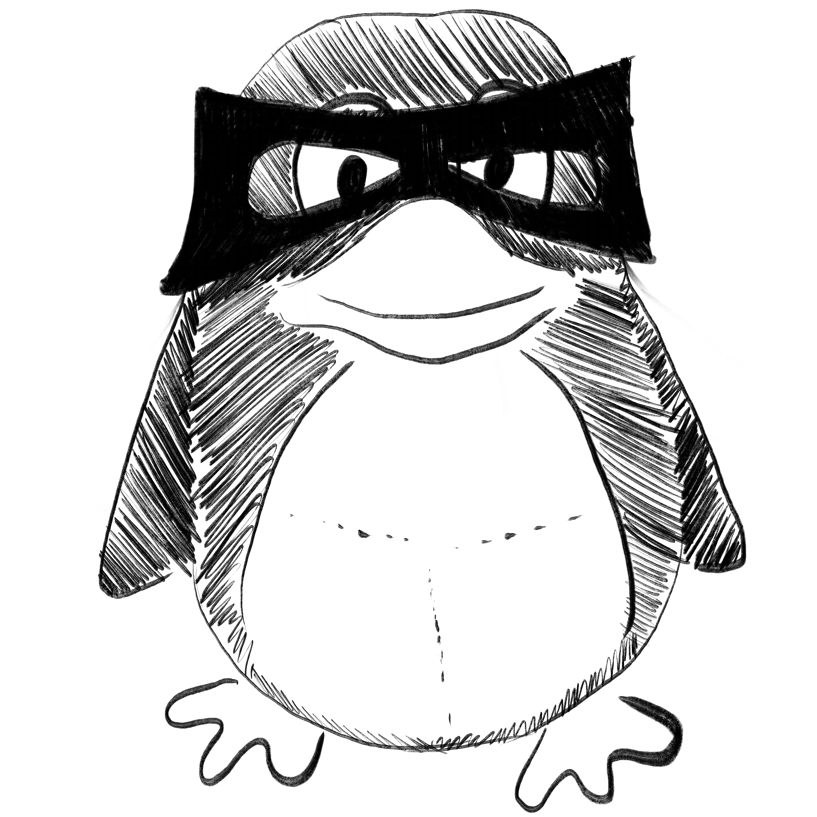
Data-Driven Technologies as Enablers for Value Creation in the Prevention of Surgical Site Infections: a Systematic Review.
In Journal of healthcare informatics research
UNLABELLED :
SUPPLEMENTARY INFORMATION :
Irgang Luís, Barth Henrik, Holmén Magnus
2023-Mar
Healthcare technology, Infection prevention and control, Surgical site infections, Systematic review, Technology implementation, Value-based care
Can Patients with Dementia Be Identified in Primary Care Electronic Medical Records Using Natural Language Processing?
In Journal of healthcare informatics research
UNLABELLED :
SUPPLEMENTARY INFORMATION :
Maclagan Laura C, Abdalla Mohamed, Harris Daniel A, Stukel Therese A, Chen Branson, Candido Elisa, Swartz Richard H, Iaboni Andrea, Jaakkimainen R Liisa, Bronskill Susan E
2023-Mar
Artificial intelligence, Dementia, Electronic health records, Natural language processing, Primary health care
Patterns of structure-function association in normal aging and in Alzheimer's disease: Screening for mild cognitive impairment and dementia with ML regression and classification models.
In Frontiers in aging neuroscience ; h5-index 64.0
BACKGROUND :
OBJECTIVE :
MATERIALS AND METHODS :
RESULTS :
CONCLUSION :
Statsenko Yauhen, Meribout Sarah, Habuza Tetiana, Almansoori Taleb M, Gorkom Klaus Neidl-Van, Gelovani Juri G, Ljubisavljevic Milos
2022
“Alzheimers disease”, aging, artificial intelligence, brain morphometry, cognitive decline, cognitive score, neurophysiological test, structural-functional association
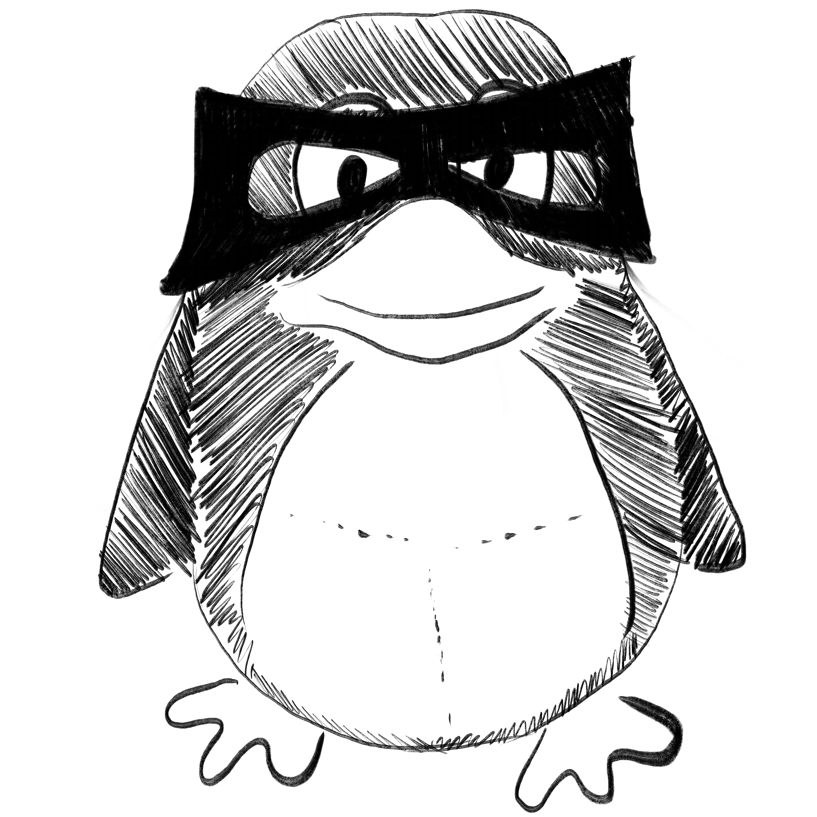
AI avatar tells you what happened: The first test of using AI-operated children in simulated interviews to train investigative interviewers.
In Frontiers in psychology ; h5-index 92.0
Haginoya Shumpei, Ibe Tatsuro, Yamamoto Shota, Yoshimoto Naruyo, Mizushi Hazuki, Santtila Pekka
2023
artificial intelligence, child sexual abuse, investigative interviewing, serious game, simulation training
Weekly Summary
Receive a weekly summary and discussion of the top papers of the week by leading researchers in the field.