Receive a weekly summary and discussion of the top papers of the week by leading researchers in the field.
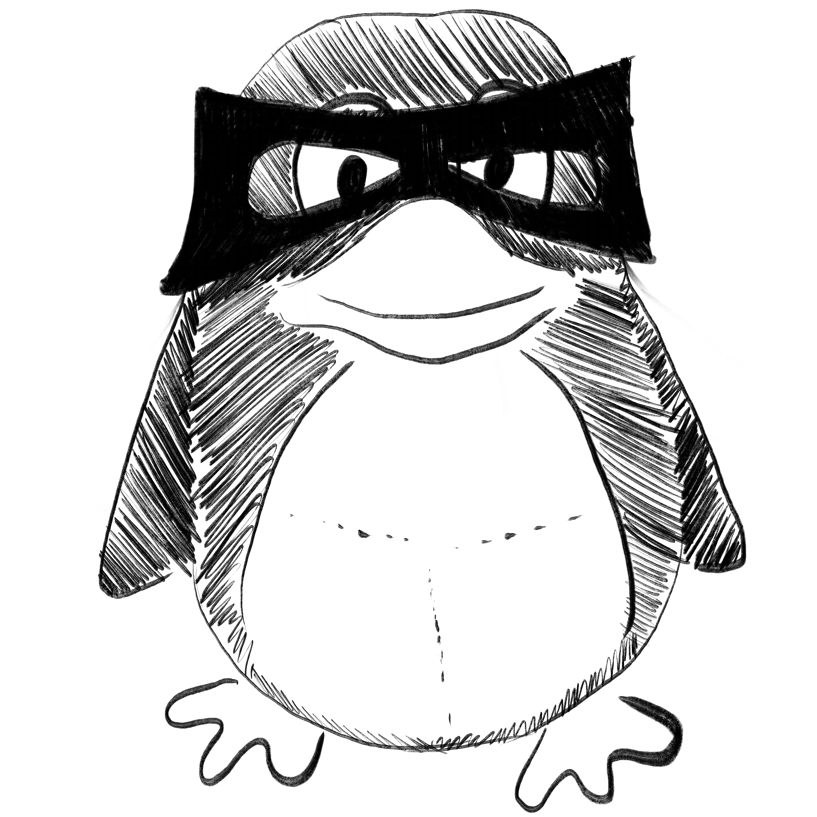
Artificial intelligence promotes shared decision-making through recommending tests to febrile pediatric outpatients.
In World journal of emergency medicine
BACKGROUND :
METHODS :
RESULTS :
CONCLUSION :
Li Wei-Hua, Dong Bin, Wang Han-Song, Yuan Jia-Jun, Qian Han, Zheng Ling-Ling, Lin Xu-Lin, Wang Zhao, Liu Shi-Jian, Ning Bo-Tao, DanTian Zhao
2023
Artificial intelligence, Medical examinations, Pediatric outpatient, Shared decision- making
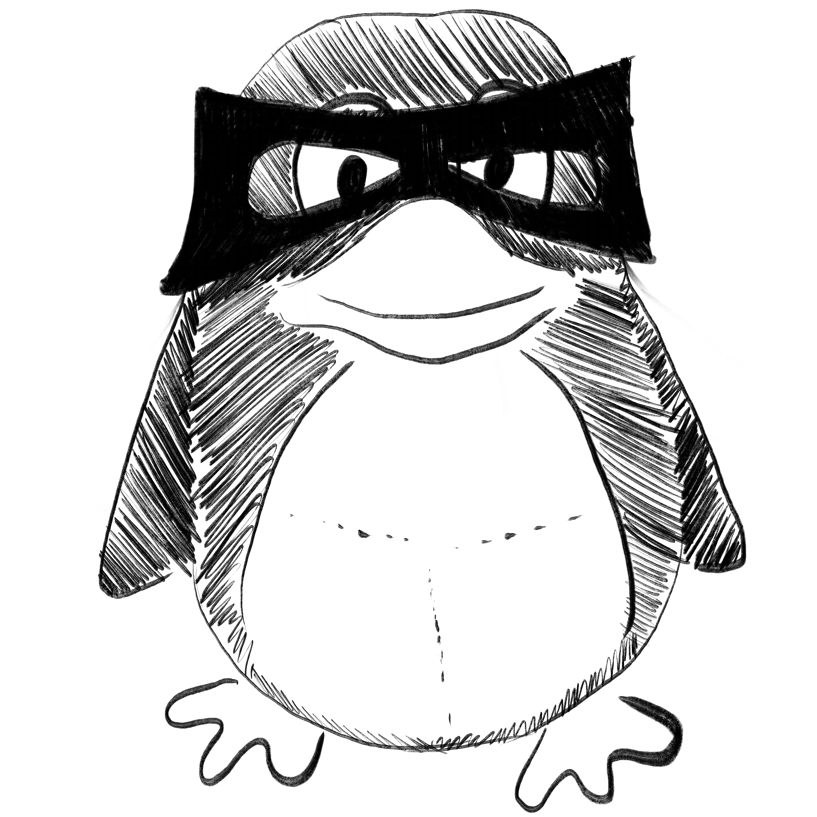
Investigating the human spirit and spirituality in pediatric patients with kidney disease.
In Frontiers in pediatrics
Woroniecki Robert, Moritz Michael L
2023
hope, quality of life, religious beliefs, resilience, social determinants of health, spirit, spirituality
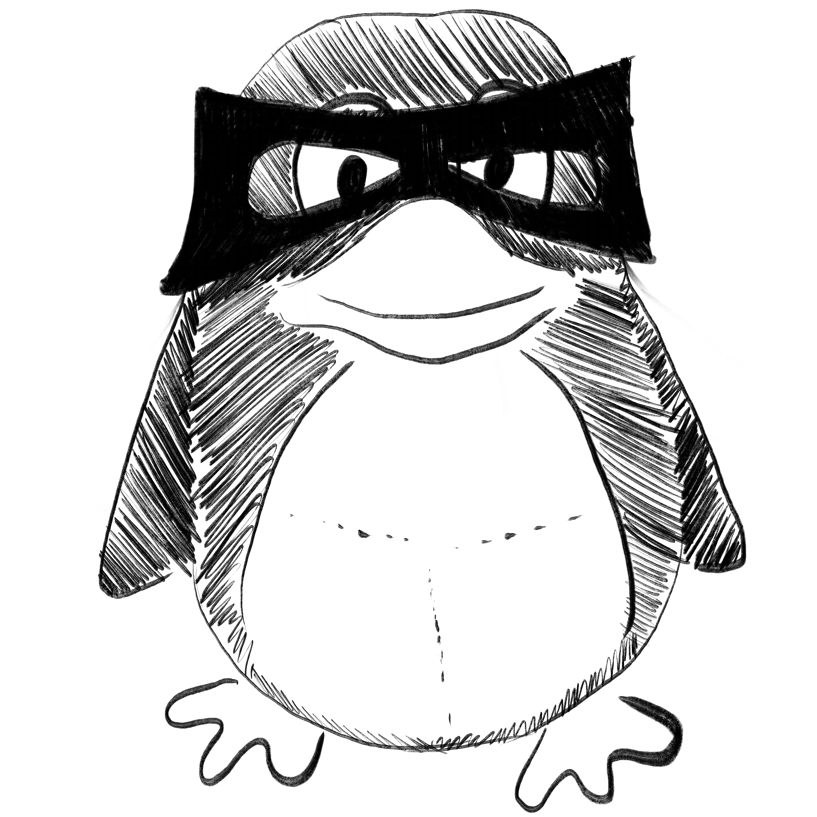
Predicting Synthesizability using Machine Learning on Databases of Existing Inorganic Materials.
In ACS omega
Zhu Ruiming, Tian Siyu Isaac Parker, Ren Zekun, Li Jiali, Buonassisi Tonio, Hippalgaonkar Kedar
2023-Mar-07
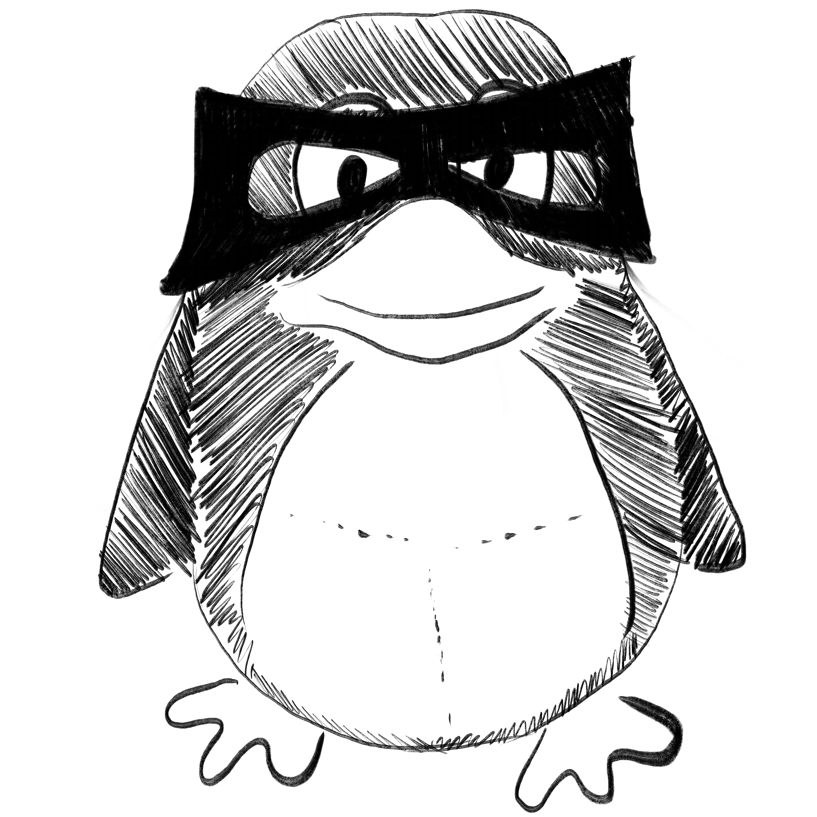
Interpretable Skin Cancer Classification based on Incremental Domain Knowledge Learning.
In Journal of healthcare informatics research
Rezk Eman, Eltorki Mohamed, El-Dakhakhni Wael
2023-Mar
Artificial intelligence, Clinical images, Domain knowledge, Interpretability, Skin cancer, Skin lesion taxonomy
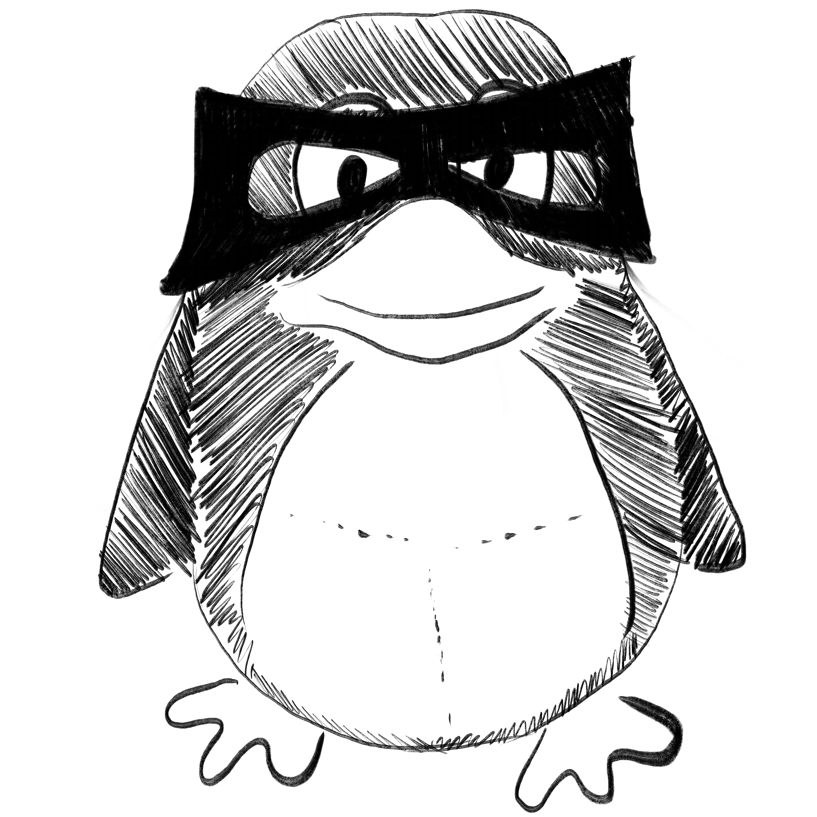
Prediction of Prednisolone Dose Correction Using Machine Learning.
In Journal of healthcare informatics research
UNLABELLED :
SUPPLEMENTARY INFORMATION :
Sato Hiroyasu, Kimura Yoshinobu, Ohba Masahiro, Ara Yoshiaki, Wakabayashi Susumu, Watanabe Hiroaki
2023-Mar
Drug safety, Imbalanced data, Machine learning, Prednisolone, Prescription error
Exploring potential barriers in equitable access to pediatric diagnostic imaging using machine learning.
In Frontiers in public health
Taheri-Shirazi Maryam, Namdar Khashayar, Ling Kelvin, Karmali Karima, McCradden Melissa D, Lee Wayne, Khalvati Farzad
2023
appointment scheduling, logistic regression, no-show, random forest, waiting room time
Weekly Summary
Receive a weekly summary and discussion of the top papers of the week by leading researchers in the field.