Receive a weekly summary and discussion of the top papers of the week by leading researchers in the field.
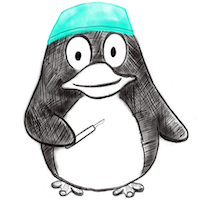
Kinematic Data-Based Action Segmentation for Surgical Applications
ArXiv Preprint
Adam Goldbraikh, Omer Shubi, Or Rubin, Carla M Pugh, Shlomi Laufer
2023-03-14
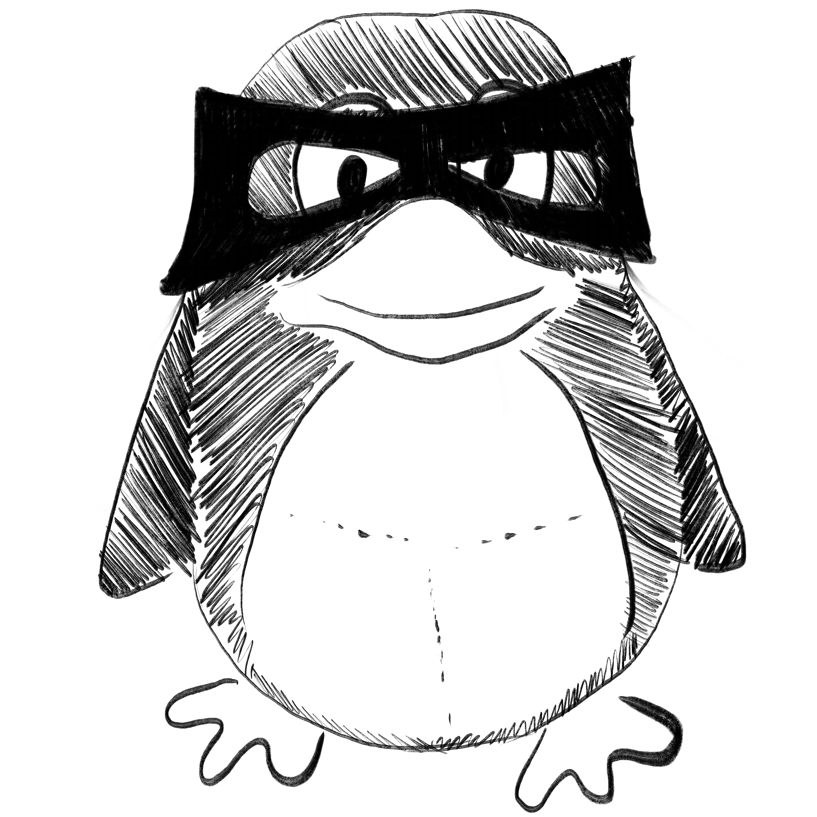
Enabling Artificial Intelligence of Things (AIoT) Healthcare Architectures and Listing Security Issues.
In Computational intelligence and neuroscience
Pise Anil Audumbar, Almuzaini Khalid K, Ahanger Tariq Ahamed, Farouk Ahmed, Pant Kumud, Pareek Piyush Kumar, Nuagah Stephen Jeswinde
2022
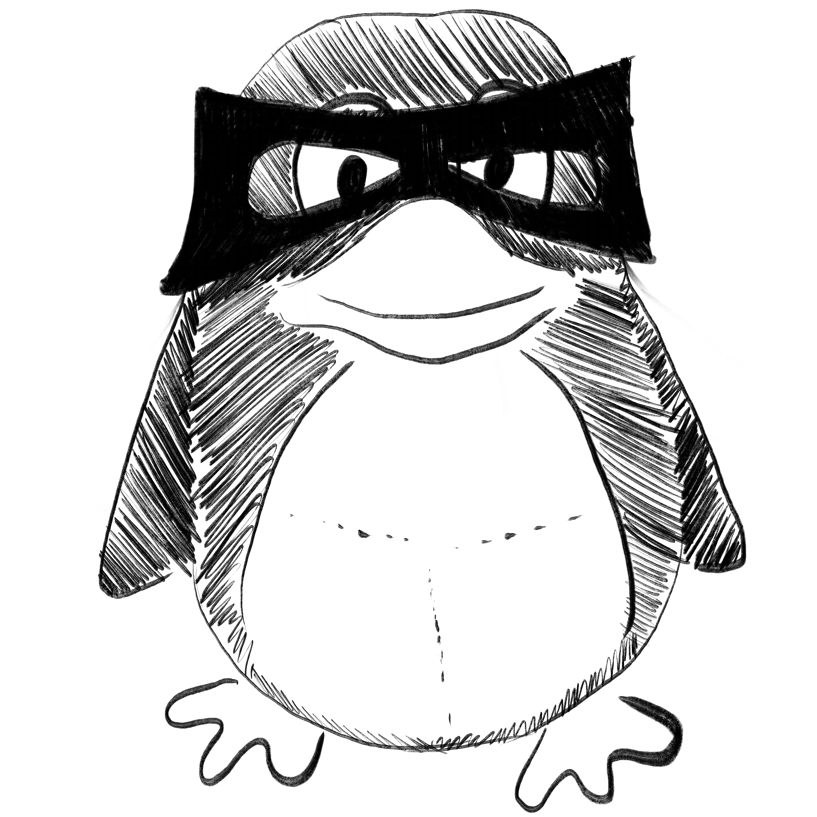
Amygdala's T1-weighted image radiomics outperforms volume for differentiation of anxiety disorder and its subtype.
In Frontiers in psychiatry
INTRODUCTION :
METHODS :
RESULTS :
DISCUSSION :
Li Qingfeng, Wang Wenzheng, Hu Zhishan
2023
amygdala, anxiety disorder, generalized anxiety disorder, magnetic resonance imaging, radiomics
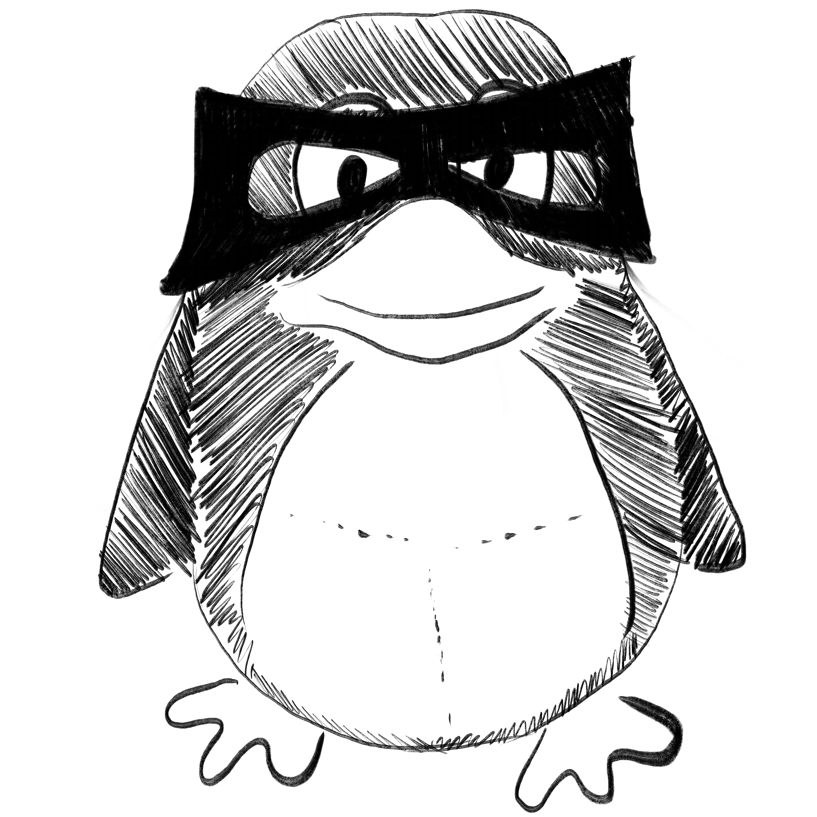
Synapses, predictions, and prediction errors: A neocortical computational study of MDD using the temporal memory algorithm of HTM.
In Frontiers in psychiatry
INTRODUCTION :
METHODS :
RESULTS :
DISCUSSION :
Sherif Mohamed A, Khalil Mostafa Z, Shukla Rammohan, Brown Joshua C, Carpenter Linda L
2023
MDD, confidence, hierarchical temporal memory (HTM), ketamine, prediction error, predictions, psilocybin
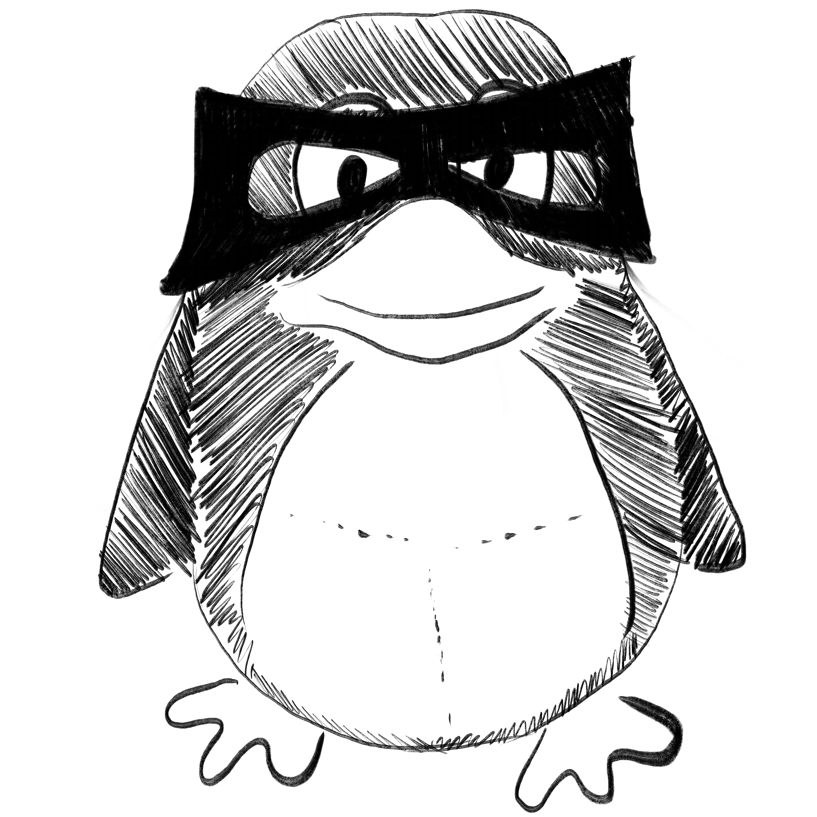
Characteristics of circulating small noncoding RNAs in plasma and serum during human aging.
In Aging medicine (Milton (N.S.W))
OBJECTIVE :
METHODS :
RESULTS :
CONCLUSION :
Xiao Ping, Shi Zhangyue, Liu Chenang, Hagen Darren E
2023-Mar
aging clock, circulating sncRNAs, human aging, machine learning
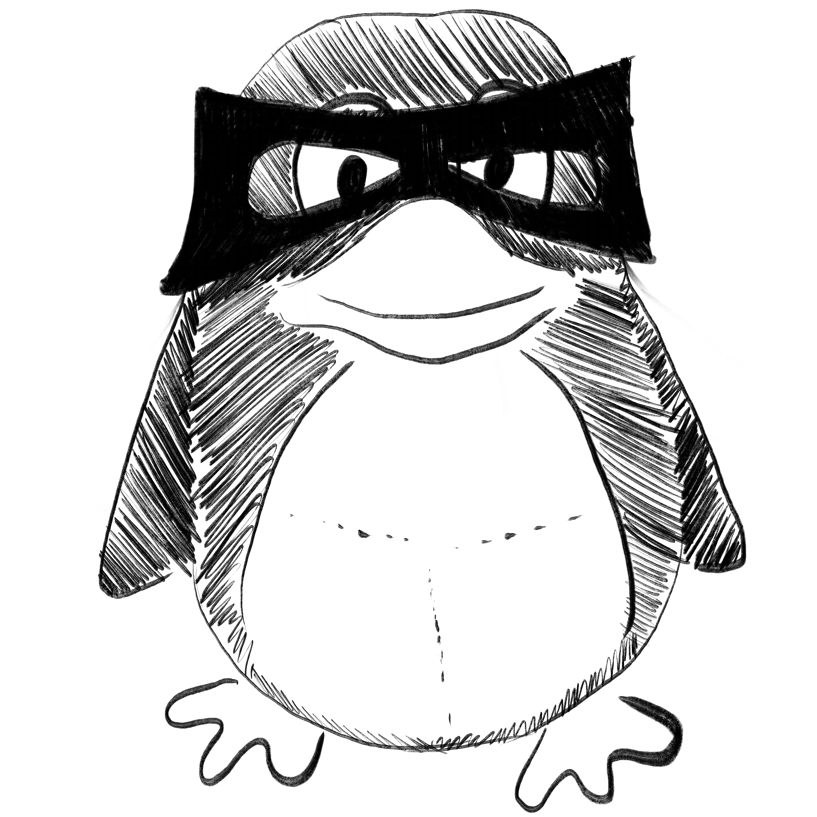
A systematic comparison of deep learning methods for EEG time series analysis.
In Frontiers in neuroinformatics
Walther Dominik, Viehweg Johannes, Haueisen Jens, Mäder Patrick
2023
attention, feed forward neural networks, recurrent neural networks, time series analysis, transformer networks
Weekly Summary
Receive a weekly summary and discussion of the top papers of the week by leading researchers in the field.