Receive a weekly summary and discussion of the top papers of the week by leading researchers in the field.
Deep learning for predicting epidermal growth factor receptor mutations of non-small cell lung cancer on PET/CT images.
In Quantitative imaging in medicine and surgery
BACKGROUND :
METHODS :
RESULTS :
CONCLUSIONS :
Xiao Zhenghui, Cai Haihua, Wang Yue, Cui Ruixue, Huo Li, Lee Elaine Yuen-Phin, Liang Ying, Li Xiaomeng, Hu Zhanli, Chen Long, Zhang Na
2023-Mar-01
EfficientNet-V2 Model, epidermal growth factor receptor (EGFR), positron emission tomography/computed tomography (PET/CT)
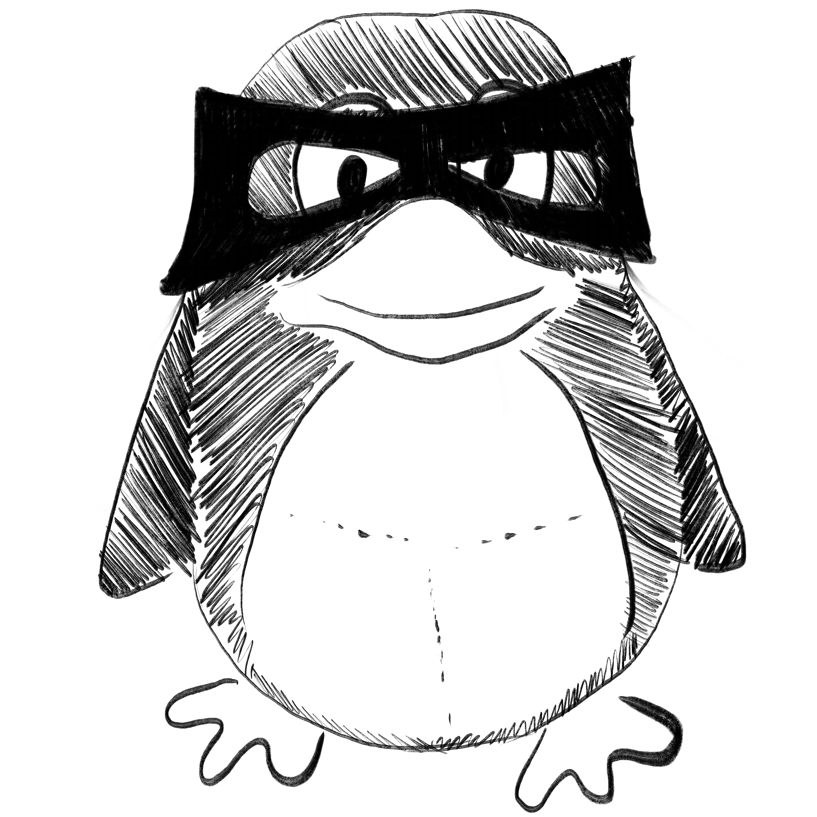
Detecting obstructive coronary artery disease with machine learning: rest-only gated single photon emission computed tomography myocardial perfusion imaging combined with coronary artery calcium score and cardiovascular risk factors.
In Quantitative imaging in medicine and surgery
BACKGROUND :
METHODS :
RESULTS :
CONCLUSIONS :
Liu Bao, Yu Wenji, Zhang Feifei, Shi Yunmei, Yang Le, Jiang Qi, Wang Yufeng, Wang Yuetao
2023-Mar-01
Machine learning (ML), coronary artery calcium score (CACS), coronary artery disease (CAD), myocardial perfusion imaging (MPI), single photon emission computed tomography (SPECT)
Machine learning for predicting accuracy of lung and liver tumor motion tracking using radiomic features.
In Quantitative imaging in medicine and surgery
BACKGROUND :
METHODS :
RESULTS :
CONCLUSIONS :
Li Guangjun, Zhang Xiangyu, Song Xinyu, Duan Lian, Wang Guangyu, Xiao Qing, Li Jing, Liang Lan, Bai Long, Bai Sen
2023-Mar-01
Tumor motion, machine learning, radiomics, respiratory motion, tumor tracking
Dark blood T2-weighted imaging of the human heart with AI-assisted compressed sensing: a patient cohort study.
In Quantitative imaging in medicine and surgery
BACKGROUND :
METHODS :
RESULTS :
CONCLUSIONS :
Yan Xianghu, Ran Lingping, Zou Lixian, Luo Yi, Yang Zhaoxia, Zhang Shiyu, Zhang Shuheng, Xu Jian, Huang Lu, Xia Liming
2023-Mar-01
Artificial intelligence (AI), MRI, T2-weighted imaging, compressed sensing, dark blood
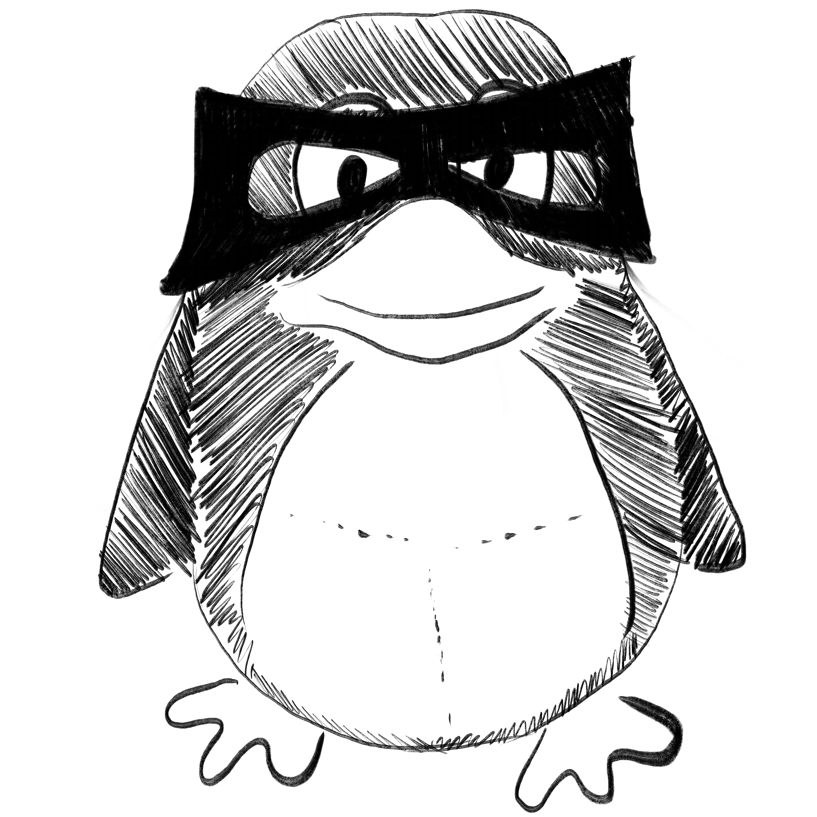
A systematic review of the modelling of patient arrivals in emergency departments.
In Quantitative imaging in medicine and surgery
BACKGROUND :
METHODS :
RESULTS :
CONCLUSIONS :
Jiang Shancheng, Liu Qize, Ding Beichen
2023-Mar-01
AED patient arrivals, PRISMA, healthcare data analytics, predicting models
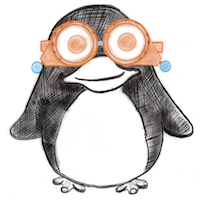
Deep learning-based image analysis of eyelid morphology in thyroid-associated ophthalmopathy.
In Quantitative imaging in medicine and surgery
BACKGROUND :
METHODS :
RESULTS :
CONCLUSIONS :
Shao Ji, Huang Xingru, Gao Tao, Cao Jing, Wang Yaqi, Zhang Qianni, Lou Lixia, Ye Juan
2023-Mar-01
Thyroid-associated ophthalmopathy (TAO), automatic measurement, deep learning, eyelid morphology, facial images
Weekly Summary
Receive a weekly summary and discussion of the top papers of the week by leading researchers in the field.