Receive a weekly summary and discussion of the top papers of the week by leading researchers in the field.
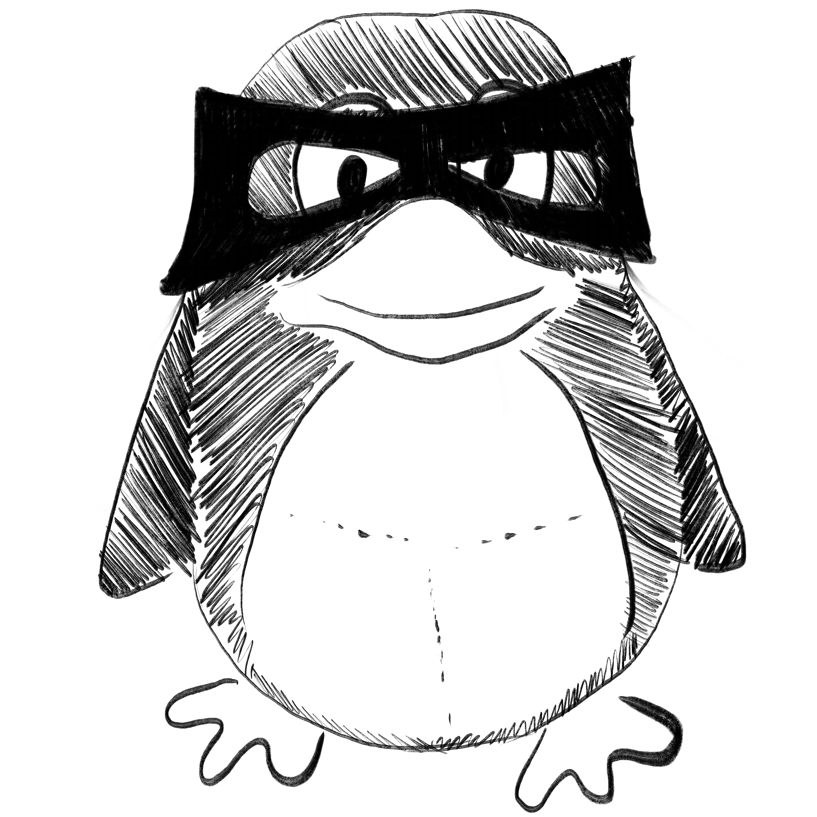
The language-related cerebro-cerebellar pathway in humans: a diffusion imaging-based tractographic study.
In Quantitative imaging in medicine and surgery
BACKGROUND :
METHODS :
RESULTS :
CONCLUSIONS :
Yin Hu, Zong Fangrong, Deng Xiaofeng, Zhang Dong, Zhang Yan, Wang Shuo, Wang Yu, Zhao Jizong
2023-Mar-01
Diffusion magnetic resonance imaging, cerebellum, language, shape analysis, tractography, white matter asymmetry
A deep learning model based on the attention mechanism for automatic segmentation of abdominal muscle and fat for body composition assessment.
In Quantitative imaging in medicine and surgery
BACKGROUND :
METHODS :
RESULTS :
CONCLUSIONS :
Shen Hao, He Pin, Ren Ya, Huang Zhengyong, Li Shuluan, Wang Guoshuai, Cong Minghua, Luo Dehong, Shao Dan, Lee Elaine Yuen-Phin, Cui Ruixue, Huo Li, Qin Jing, Liu Jun, Hu Zhanli, Liu Zhou, Zhang Na
2023-Mar-01
CT scan, deep learning, fat segmentation, muscle segmentation, sarcopenia
Group theoretic particle swarm optimization for multi-level threshold lung cancer image segmentation.
In Quantitative imaging in medicine and surgery
BACKGROUND :
METHODS :
RESULTS :
CONCLUSIONS :
Lan Kun, Zhou Jianqiang, Jiang Xiaoliang, Wang Jun, Huang Shigao, Yang Jie, Song Qun, Tang Rui, Gong Xueyuan, Liu Kexing, Wu Yaoyang, Li Tengyue
2023-Mar-01
Lung cancer detection, evolutionary computation, group theory, medical image segmentation, metaheuristic
A machine learning approach for preoperatively assessing pulmonary function with computed tomography in patients with lung cancer.
In Quantitative imaging in medicine and surgery
BACKGROUND :
METHODS :
RESULTS :
CONCLUSIONS :
Meng Hongjia, Liu Yun, Xu Xiaoyin, Liao Yuting, Liang Hengrui, Chen Huai
2023-Mar-01
Machine learning, assessment, computed tomography (CT), lung cancer, pulmonary function
Early prediction of acute pancreatitis severity based on changes in pancreatic and peripancreatic computed tomography radiomics nomogram.
In Quantitative imaging in medicine and surgery
BACKGROUND :
METHODS :
RESULTS :
CONCLUSIONS :
Zhao Yanmei, Wei Jiayi, Xiao Bo, Wang Liu, Jiang Xian, Zhu Yuanzhong, He Wenjing
2023-Mar-01
Radiomics, acute pancreatitis (AP), enhanced computed tomography (enhanced CT), machine learning, nomogram
Radiation dose reduction using deep learning-based image reconstruction for a low-dose chest computed tomography protocol: a phantom study.
In Quantitative imaging in medicine and surgery
BACKGROUND :
METHODS :
RESULTS :
CONCLUSIONS :
Jung Yunsub, Hur Jin, Han Kyunghwa, Imai Yasuhiro, Hong Yoo Jin, Im Dong Jin, Lee Kye Ho, Desnoyers Melissa, Thomsen Brian, Shigemasa Risa, Um Kyounga, Jang Kyungeun
2023-Mar-01
Low-dose chest computed tomography (LDCT), chest phantom, deep learning-based image reconstruction (DLIR)
Weekly Summary
Receive a weekly summary and discussion of the top papers of the week by leading researchers in the field.