Receive a weekly summary and discussion of the top papers of the week by leading researchers in the field.
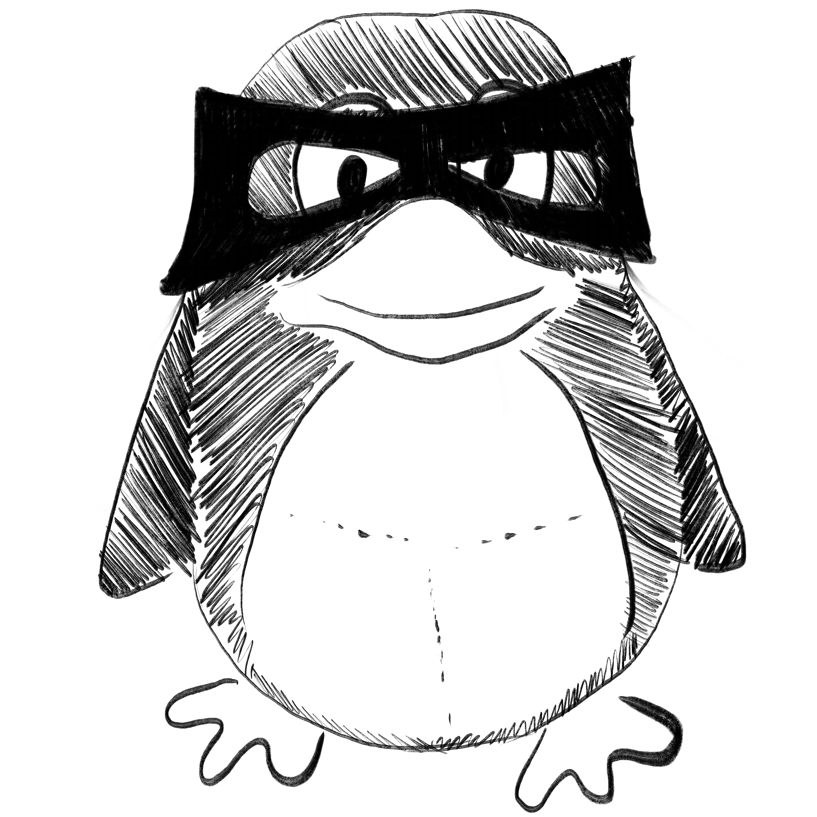
Predicting Personalized Responses to Dietary Fiber Interventions: Opportunities for Modulation of the Gut Microbiome to Improve Health.
In Annual review of food science and technology
Kok Car Reen, Rose Devin, Hutkins Robert
2022-Nov-29
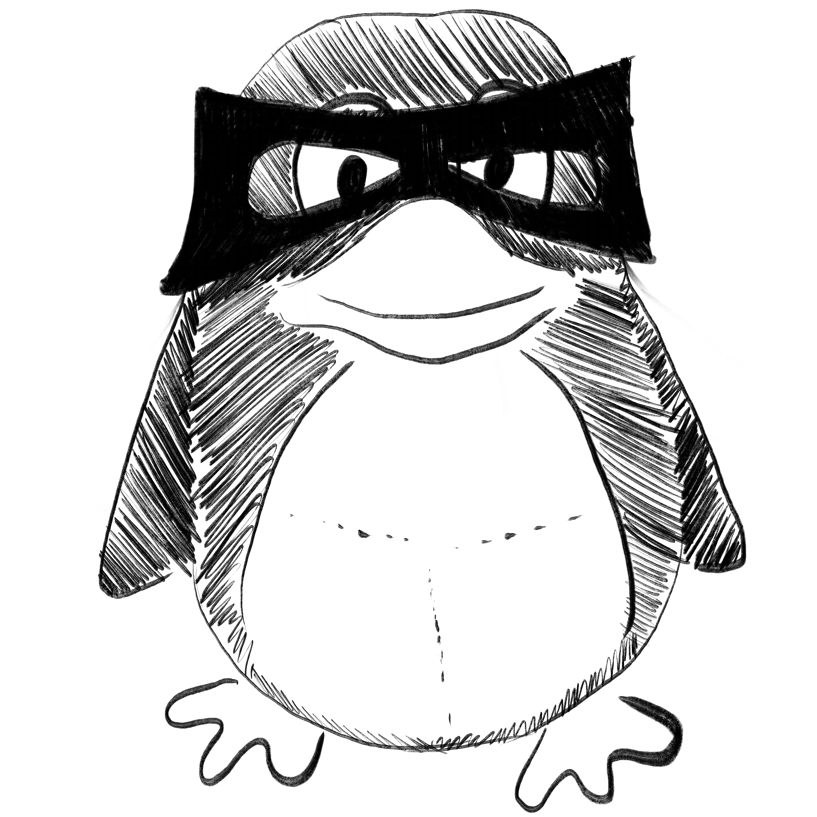
Advanced Scalability for Light Field Image Coding.
In IEEE transactions on image processing : a publication of the IEEE Signal Processing Society
Amirpour Hadi, Guillemot Christine, Ghanbari Mohammad, Timmerer Christian
2022-Nov-29
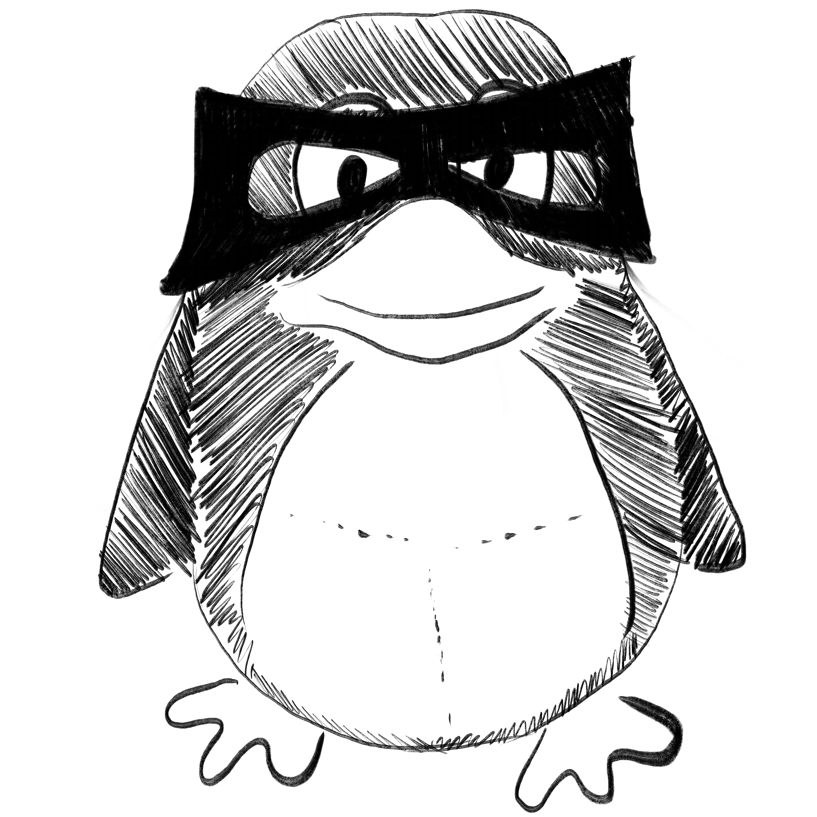
SleepPPG-Net: A Deep Learning Algorithm for Robust Sleep Staging From Continuous Photoplethysmography.
In IEEE journal of biomedical and health informatics
Kotzen Kevin, Charlton Peter H, Salabi Sharon, Amar Lea, Landesberg Amir, Behar Joachim A
2022-Nov-29
A 2.5D Deep Learning-Based Method for Drowning Diagnosis Using Post-Mortem Computed Tomography.
In IEEE journal of biomedical and health informatics
Zeng Yuwen, Zhang Xiaoyong, Kawasumi Yusuke, Usui Akihito, Ichiji Kei, Funayama Masato, Homma Noriyasu
2022-Nov-29
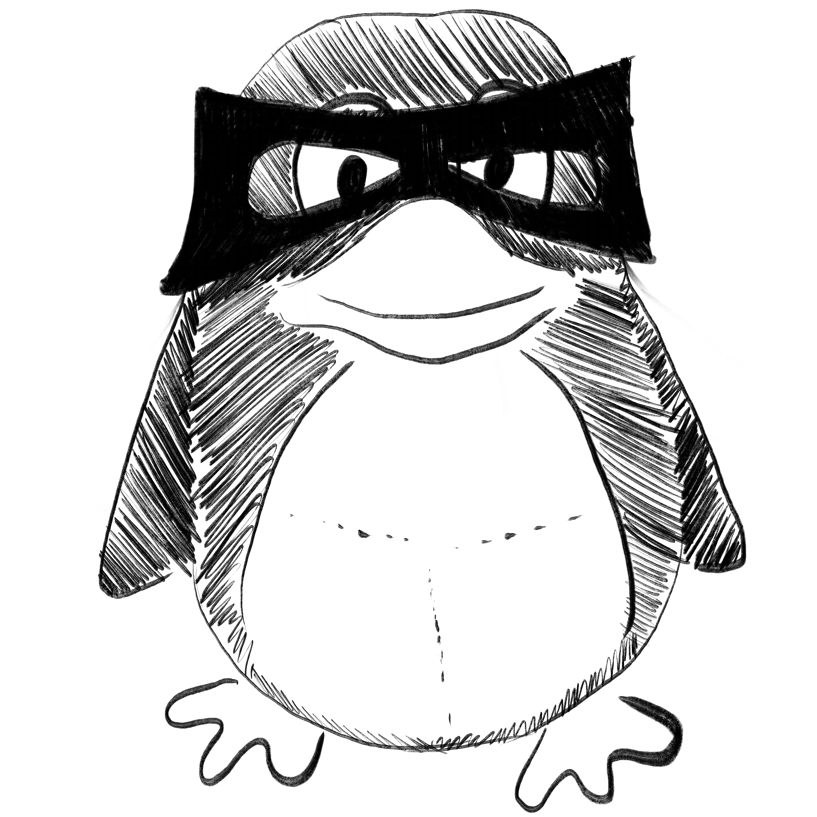
Identifying and distinguishing of essential tremor and Parkinson's disease with grouped stability analysis based on searchlight-based MVPA.
In Biomedical engineering online
BACKGROUND :
PURPOSE :
METHODS :
RESULTS :
CONCLUSIONS :
Cheng FuChao, Duan YuMei, Jiang Hong, Zeng Yu, Chen XiaoDan, Qin Ling, Zhao LiQin, Yi FaSheng, Tang YiQian, Liu Chang
2022-Nov-28
Essential tremor, Grouped stability analysis, MVPA, Neuroimaging biomarkers, Parkinson’s disease
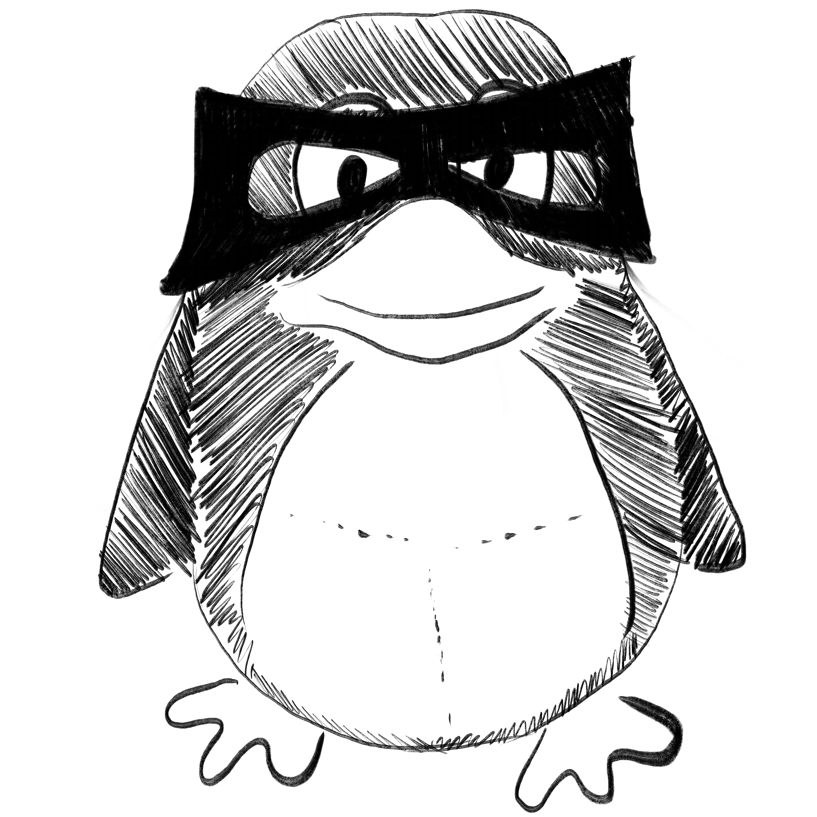
Disease-related compound identification based on deeping learning method.
In Scientific reports ; h5-index 158.0
Yang Bin, Bao Wenzheng, Wang Jinglong, Chen Baitong, Iwamori Naoki, Chen Jiazi, Chen Yuehui
2022-Nov-29
Weekly Summary
Receive a weekly summary and discussion of the top papers of the week by leading researchers in the field.